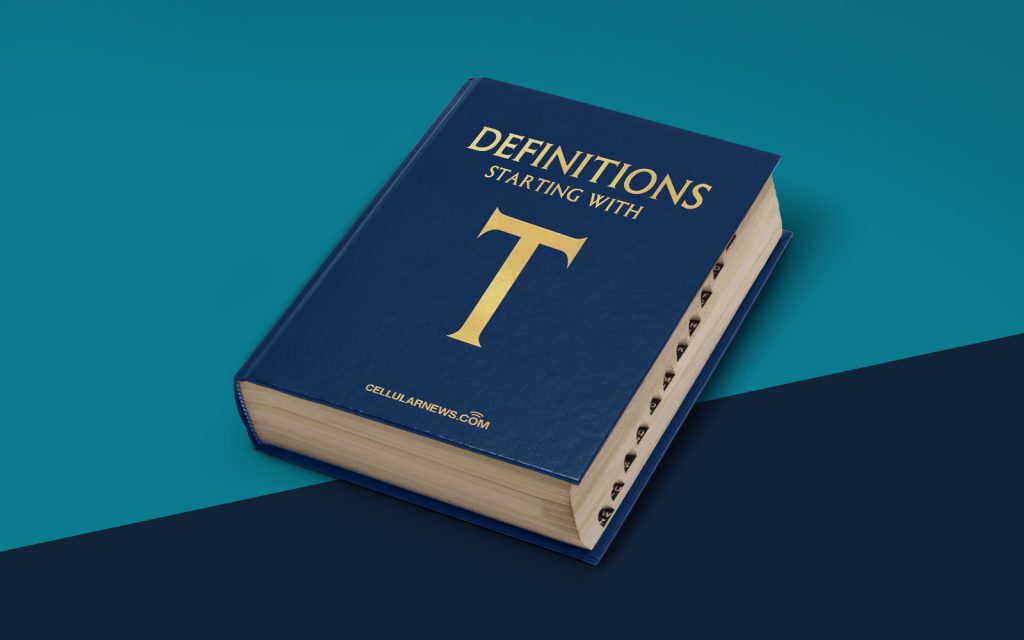
What is a Tensor Processing Unit (TPU)? A Comprehensive Definition
Welcome to the DEFINITIONS category on our page! In this post, we’re going to dive deep into the fascinating world of Tensor Processing Units (TPUs) and help demystify this cutting-edge technology. If you’ve ever wondered what a TPU is and how it impacts various industries, you’re in the right place. So, let’s get started!
Key Takeaways:
- A Tensor Processing Unit (TPU) is a specialized chip developed by Google to accelerate machine learning workloads efficiently.
- TPUs are designed to provide superior performance in tasks that involve large-scale parallel computation and deep neural networks.
Now that we have our key takeaways, let’s delve into the world of TPUs and explore their purpose, capabilities, and impact in today’s technology-driven landscape.
Understanding TPUs:
A Tensor Processing Unit (TPU) is a specialized hardware chip developed by Google specifically for accelerating machine learning workloads. More than just a traditional central processing unit (CPU) or graphics processing unit (GPU), TPUs are designed with a focus on high performance in tasks that involve large-scale parallel computation and deep neural networks.
TPUs are built using a custom architecture optimized for the TensorFlow framework, a popular open-source machine learning library. This specialized architecture enables TPUs to process and execute complex mathematical operations required for training and running artificial intelligence models with exceptional speed and efficiency.
The Advantages of TPUs:
So, why would someone choose a TPU over a traditional CPU or GPU? Here are some key advantages that make TPUs stand out:
- Superior Performance: TPUs are specifically designed to accelerate machine learning workloads, providing faster processing speeds compared to CPUs and even GPUs in many cases. This speed boost allows for quicker training and inference times, enabling organizations to make data-driven decisions more efficiently.
- Energy Efficiency: TPUs excel in terms of energy efficiency, consuming significantly less power compared to CPUs and GPUs. This helps reduce operational costs and lowers the environmental impact of running resource-intensive machine learning processes.
- Scalability: As TPUs are purpose-built for parallel computation, they can scale to handle large datasets and complex neural network models. This scalability enables researchers and developers to tackle more significant challenges in the realm of artificial intelligence.
Applications of TPUs:
TPUs find applications across various industries, driving advancements in numerous fields. Here are some examples:
- Healthcare: TPUs help in analyzing large medical datasets and improving disease diagnosis accuracy.
- Finance: TPUs enable faster fraud detection and risk assessment in real-time financial transactions.
- Autonomous Vehicles: TPUs support the development of self-driving cars by accelerating computer vision and sensor data processing.
- Natural Language Processing: TPUs enhance language understanding and translation capabilities, improving virtual assistants and chatbots.
- Scientific Research: TPUs aid in scientific simulations, accelerating discoveries and breakthroughs in various fields.
As TPUs continue to advance, their applications will proliferate, revolutionizing industries and driving innovation.
In Conclusion:
In summary, a Tensor Processing Unit (TPU) is a specialized chip designed by Google to accelerate machine learning workloads efficiently. With exceptional performance, energy efficiency, and scalability, TPUs have become a game-changer in the field of artificial intelligence. Whether it’s in healthcare, finance, autonomous vehicles, or scientific research, TPUs continue to shape the future of various industries, unlocking new possibilities for innovation and growth.
We hope this comprehensive definition has shed light on the world of TPUs and their significance in the realm of machine learning. Stay tuned for more engaging content in our DEFINITIONS category, where we demystify complex concepts to help you stay informed!