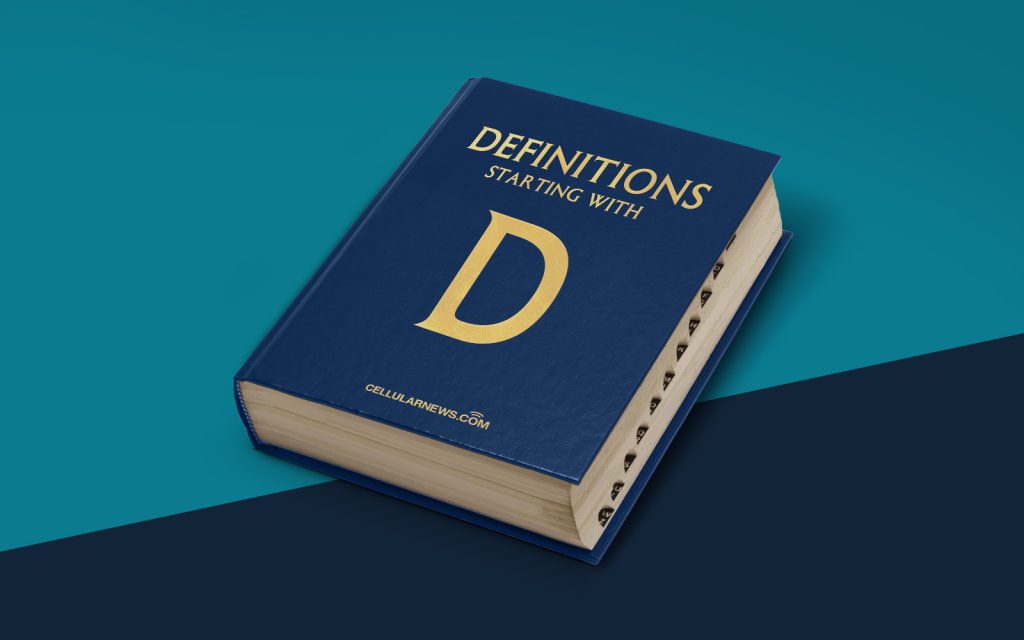
Defining Data Quality Management (DQM)
Welcome to another exciting installment of our “Definitions” category! In today’s post, we will be delving into the fascinating world of Data Quality Management (DQM). If you’ve ever wondered what DQM is all about, you’ve come to the right place! So, let’s get started and uncover the mysteries of Data Quality Management.
Key Takeaways
- Data Quality Management (DQM) is the process of ensuring that data is accurate, reliable, consistent, and relevant.
- Effective DQM is crucial for businesses to make informed decisions, improve operational efficiency, and ensure compliance.
The Importance of Data Quality Management
In today’s fast-paced digital landscape, organizations generate and handle massive amounts of data on a daily basis. Whether it’s customer information, financial records, or operational metrics, data plays a vital role in driving business growth and success. However, with such vast amounts of data, ensuring its quality is of utmost importance. This is where Data Quality Management (DQM) comes into play.
Data Quality Management (DQM) is the process of implementing strategies, technologies, and best practices to ensure that data is accurate, reliable, consistent, and relevant. It involves assessing the quality of data, identifying any issues or errors, and taking necessary actions to mitigate them. By improving the quality of data, organizations can make informed and reliable decisions, enhance operational efficiency, and stay compliant with industry regulations.
Now that we know what Data Quality Management (DQM) is, let’s take a closer look at why it is so important:
- Enhanced Decision-making: High-quality data provides valuable insights that help organizations make informed and data-driven decisions. When the data is accurate, reliable, and consistent, leaders can trust it to provide accurate and meaningful information for analysis and decision-making processes.
- Operational Efficiency: Inaccurate or inconsistent data can lead to inefficiencies and errors in business operations. By implementing effective DQM practices, organizations can ensure that data is up to date, standardized, and complete, thus improving operational efficiency.
- Regulatory Compliance: Many industries have strict regulations regarding data quality and privacy. By adopting DQM practices, organizations can ensure that their data is compliant with these regulations, thus avoiding legal complications and penalties.
- Customer Satisfaction: Data quality directly impacts customer satisfaction. Inaccurate customer data can lead to poor customer experiences, missed opportunities, and damage to the brand reputation. With DQM, businesses can maintain accurate and complete customer data, leading to improved customer satisfaction and loyalty.
Data Quality Management (DQM) is a continuous process that requires a combination of technology, data governance, and human intervention. By investing in DQM, organizations can unlock the true potential of their data, drive business growth, and stay ahead of the competition.
We hope this article has shed some light on the intricacies of Data Quality Management (DQM). Remember, data is the lifeblood of businesses in the digital age, and ensuring its quality should be a top priority. Stay tuned for more informative posts in our “Definitions” category, where we demystify complex concepts and bring clarity to your digital journey.