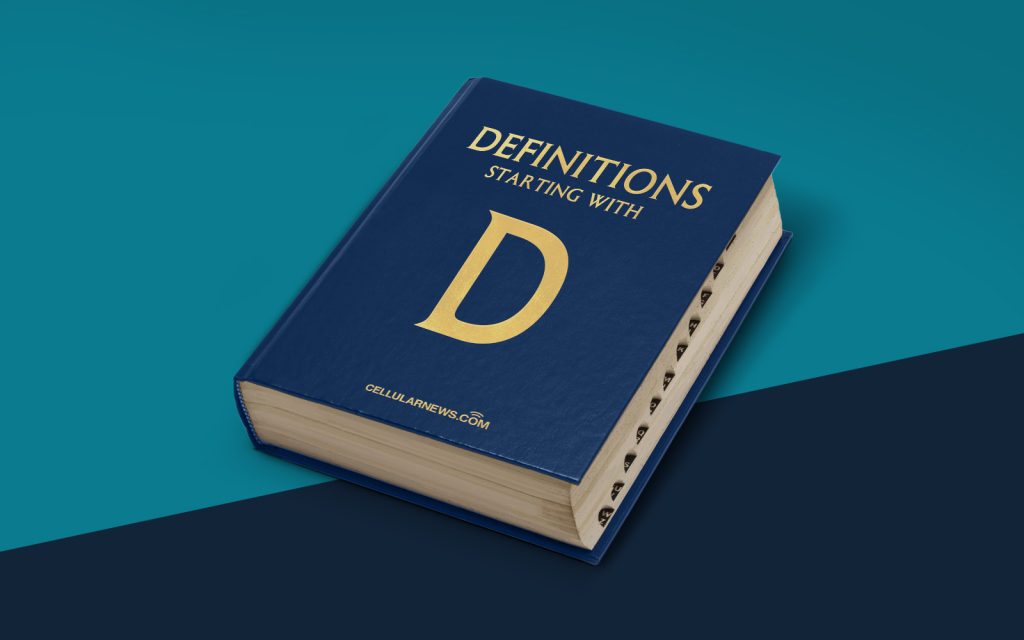
Welcome to the World of Data Quality!
Do you ever wonder why some websites appear on the first page of a Google search while others are buried in the depths of the internet? The secret lies in data quality. In this post, we’ll delve into the world of data quality and explore what it means for businesses and organizations.
Key Takeaways
- Data quality refers to the accuracy, completeness, and reliability of data.
- Poor data quality can lead to costly errors, missed opportunities, and a loss of trust in the data.
Why Does Data Quality Matter?
Data quality is crucial for any organization that relies on data to make informed decisions. Here’s why it matters:
- Accurate Insights: High-quality data ensures that the insights drawn from it are accurate and reliable. Without reliable data, businesses may make inaccurate decisions that could harm their operations or miss valuable opportunities.
- Costly Errors: Poor data quality can lead to costly errors. For example, incorrect customer data could result in sending promotions or notifications to the wrong audience, wasting resources and potential revenue.
- Loss of Trust: Inaccurate or unreliable data erodes trust in the information, both internally within the organization and externally among customers and stakeholders. A loss of trust can have detrimental effects on business relationships and reputation.
- Operational Efficiency: Reliable data ensures smooth operations and efficient processes. Inaccurate or incomplete data can lead to delays, redundancies, and unnecessary rework, impacting productivity and profitability.
The Elements of Data Quality
Now that we understand the importance of data quality, let’s explore its key elements:
1. Accuracy
“Garbage in, garbage out” – This adage emphasizes the significance of accurate data. Accurate data is free from errors, inconsistencies, and outdated information. It reflects the true reality or state of affairs and can be relied upon for decision-making purposes.
2. Completeness
Data completeness refers to the presence of all required information. Complete data contains all relevant fields and attributes, leaving no gaps or missing values. Incomplete data can hinder analysis and provide an incomplete picture of the situation.
3. Consistency
Consistent data is uniform and follows a standardized format or structure. Consistency ensures that data can be easily compared, integrated, and analyzed without encountering conflicts or discrepancies. It promotes cohesive decision-making across an organization.
4. Timeliness
Timeliness refers to the currency and relevance of data. Up-to-date data provides the most accurate view of current reality, enabling organizations to make informed and timely decisions. Outdated or obsolete data can lead to misinformed decisions and missed opportunities.
5. Validity
Valid data adheres to defined integrity rules and constraints. It meets the required data formats, boundaries, and relationships set by the organization. Valid data ensures that the information is accurate and meaningful, enabling reliable analysis and decision-making processes.
Wrapping Up
Data quality is essential for any organization that wants to make informed decisions and stay ahead of the competition. Accuracy, completeness, consistency, timeliness, and validity are the key elements that define data quality. By prioritizing data quality, businesses can unlock the full potential of their data and gain a competitive edge in the data-driven world.