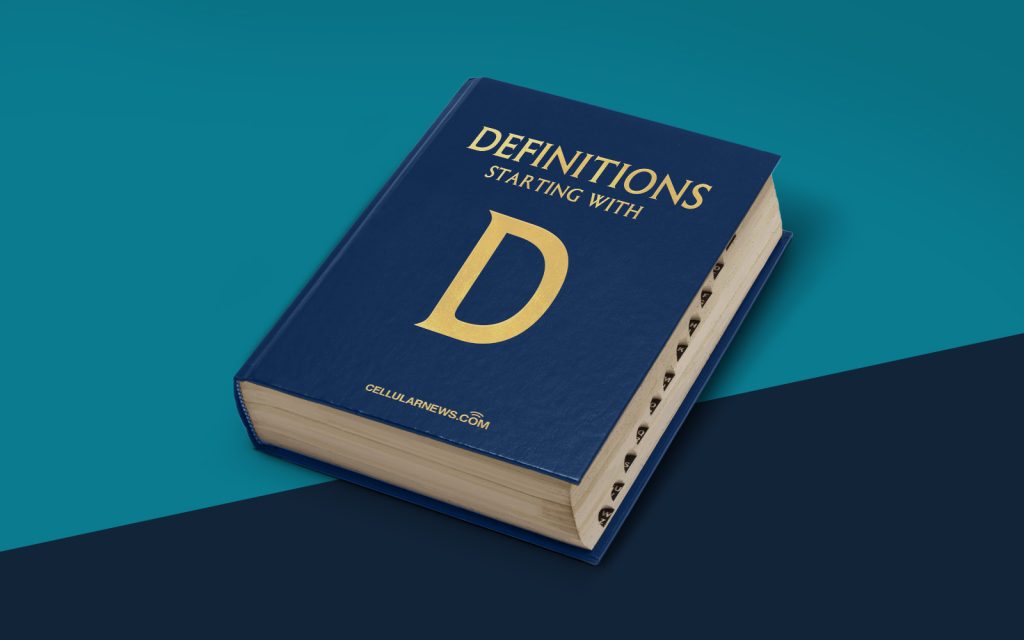
Welcome to the Definitions category: Demystifying Deep Reinforcement Learning (Deep RL)
Have you ever wondered what makes artificial intelligence (AI) systems capable of making decisions and taking actions in complex environments? Deep Reinforcement Learning (Deep RL) is the answer! In this article, we delve into the fascinating world of Deep RL, explaining its concept, benefits, and applications. By the end of this read, you’ll have a clear understanding of the fundamentals of Deep RL and how it revolutionizes the field of AI.
Key Takeaways:
- Deep Reinforcement Learning combines Deep Learning and Reinforcement Learning techniques to build AI systems capable of learning and making decisions in complex environments.
- Deep RL has vast applications, ranging from robotics and self-driving cars to game playing and healthcare.
Unraveling the Depths of Deep Reinforcement Learning
In simple terms, Deep RL is an approach that allows AI systems to learn to make decisions and take actions based on the feedback they receive from their environment. This feedback, known as rewards, guides the system to optimize its behavior over time, ultimately maximizing its cumulative rewards.
Here’s a breakdown of the key components and concepts behind Deep RL:
- Deep Learning: Deep RL leverages the power of Deep Learning, a subset of AI that uses artificial neural networks to learn and uncover patterns from vast amounts of data. Deep neural networks consist of multiple layers and are capable of automatically extracting high-level features, delivering superior performance in various tasks.
- Reinforcement Learning (RL): RL involves an agent interacting with an environment, learning to maximize its rewards by taking actions that yield positive outcomes. The agent learns through a trial-and-error process, receiving rewards or penalties based on its actions. Over time, it adapts its behavior to achieve the maximum cumulative reward.
- Value Function: In Deep RL, the agent uses a value function to estimate the expected future rewards for each state or action. This function guides the agent’s decision-making process, enabling it to choose the actions that yield the highest long-term reward.
Now that we have a basic understanding of Deep RL, let’s explore its applications across various domains:
The Limitless Applications of Deep RL
The versatility of Deep RL makes it applicable to a wide range of fields. Here are just a few examples:
- Robotics: Deep RL enables robots to learn complex tasks autonomously, such as grasping objects or navigating through challenging environments.
- Autonomous Vehicles: Self-driving cars can benefit from Deep RL by learning to make decisions in real-time, leading to safer and more efficient transportation systems.
- Game Playing: Deep RL has been instrumental in achieving human-level performance in games such as Go, chess, and video games, demonstrating its ability to learn and strategize.
- Healthcare: Deep RL can aid in optimizing treatment plans, drug discovery, and patient monitoring, ultimately improving healthcare outcomes.
These are just a few examples, and the potential applications of Deep RL are continuously expanding as researchers and practitioners explore its capabilities.
In Conclusion
Deep Reinforcement Learning brings together the power of Deep Learning and Reinforcement Learning to create AI systems that can learn, make decisions, and take actions in complex environments. With its growing applications across various domains, Deep RL is revolutionizing the field of AI, bringing us closer to achieving truly intelligent machines.
So next time you encounter the term “Deep Reinforcement Learning,” you’ll have a solid understanding of what it means and how it transforms AI. Stay curious, keep exploring, and embrace the future of intelligent systems!