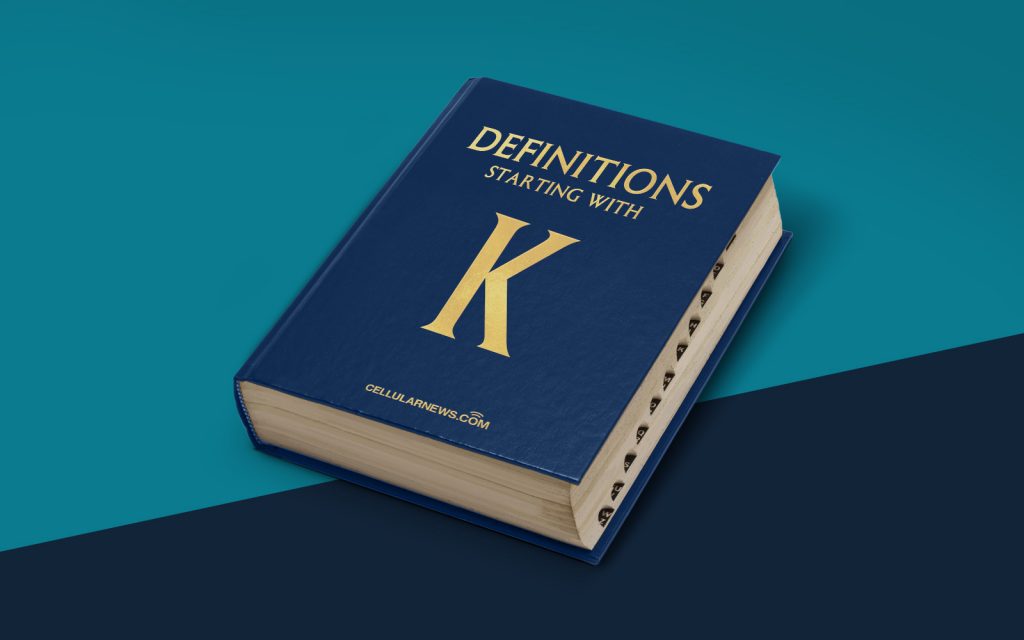
DEFINITIONS: Knowledge Discovery in Databases (KDD)
Welcome to our “DEFINITIONS” category where we delve into various terms and concepts related to technology, computing, and data. In this blog post, we are going to explore the fascinating field of Knowledge Discovery in Databases (KDD). So, without further ado, let’s dive in!
What is Knowledge Discovery in Databases (KDD)?
Knowledge Discovery in Databases, commonly known as KDD, refers to the process of extracting and discovering valuable information and patterns from large volumes of data. It encompasses a range of techniques, including data mining, machine learning, statistics, and database systems, to uncover hidden insights and transform them into actionable knowledge.
Key Takeaways:
- KDD involves the exploration and analysis of vast amounts of data to discover meaningful patterns, trends, and relationships.
- It utilizes various techniques such as data mining, machine learning, and statistical analysis to uncover valuable knowledge hidden within the data.
The Process of KDD
The process of Knowledge Discovery in Databases consists of several distinct steps:
- Data Cleaning: This step involves removing irrelevant or noisy data, handling missing values, and resolving inconsistencies within the dataset.
- Data Integration: In this step, multiple data sources are combined and integrated to create a unified dataset for analysis.
- Data Selection: Relevant subsets of data are selected based on specific criteria or requirements.
- Data Transformation: The selected data is transformed using various techniques, such as normalization or aggregation, to facilitate the discovery of patterns or trends.
- Data Mining: This is the core step of KDD, where algorithms and techniques are applied to extract useful patterns, relationships, and knowledge from the transformed data.
- Pattern Evaluation: The discovered patterns are then evaluated based on their usefulness, validity, and potential for actionable insights.
- Knowledge Presentation: Finally, the insights and knowledge gained from the patterns are presented in a suitable form, such as reports, visualizations, or predictive models.
Applications of KDD
KDD has a wide range of applications across various industries and domains. Here are a few examples:
- Business: KDD helps companies gain insights into customer behavior, market trends, and optimize their processes for better decision-making.
- Healthcare: KDD aids in discovering patterns in medical data, enabling early detection of diseases, prediction of patient outcomes, and personalized treatment plans.
- Finance: KDD assists in fraud detection, risk assessment, and investment strategies by analyzing vast financial datasets.
- Research: KDD aids scientists in analyzing large datasets to identify patterns, trends, and correlations, leading to breakthroughs in various fields.
Conclusion
Knowledge Discovery in Databases (KDD) is a powerful approach to extracting valuable insights from large volumes of data. It involves the use of various techniques and algorithms to discover hidden patterns and transform them into actionable knowledge. With its wide range of applications, KDD is an essential tool for businesses, researchers, and decision-makers who seek to gain a competitive edge and unlock the potential of their data. By harnessing the power of KDD, organizations can make informed decisions, improve processes, and drive innovation.