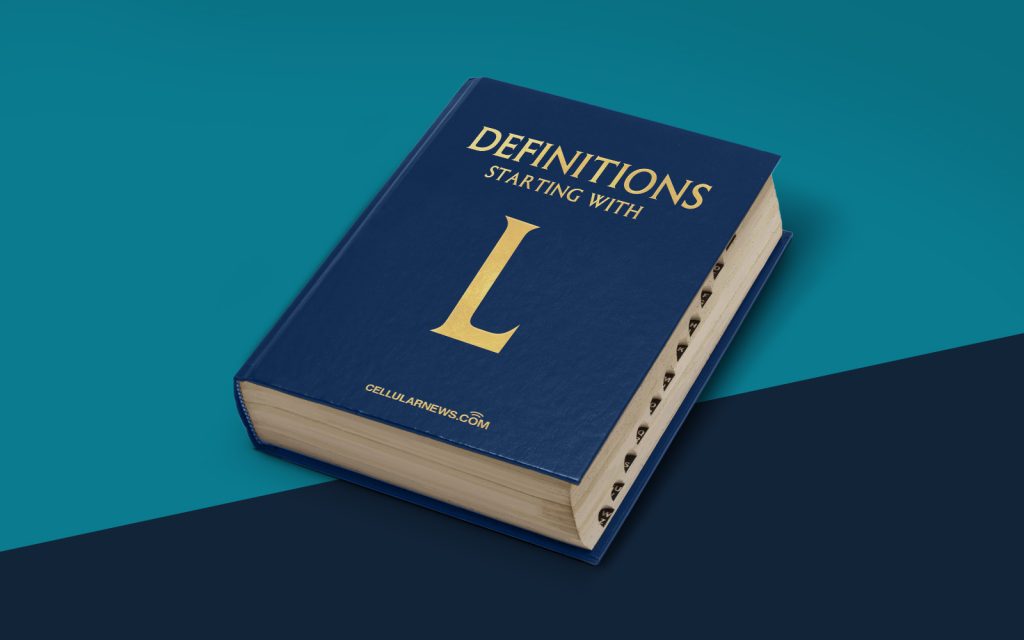
Understanding Layer-Wise Relevance Propagation
Welcome to another installment of our “DEFINITIONS” series, where we delve into the intricacies of various topics in the world of technology and beyond. Today, we will be exploring the concept of Layer-Wise Relevance Propagation (LRP) — a powerful technique used in the field of machine learning and neural networks. So, sit back, relax, and let us guide you through this fascinating subject!
Key Takeaways:
- Layer-Wise Relevance Propagation (LRP) is a method used in machine learning to understand the contribution of specific features or neurons in the input data to the final prediction of a neural network model.
- LRP helps to uncover the reasoning behind a neural network’s decision-making process, making it an invaluable tool for interpretability and explainability in various fields such as healthcare, finance, and autonomous vehicles.
Now, let’s dive deeper into the world of Layer-Wise Relevance Propagation and explore what makes it such a crucial technique in the realm of artificial intelligence.
Layer-Wise Relevance Propagation is a method that enables us to understand the inner workings of complex neural networks by attributing relevance values to individual input features. By estimating the contribution of each feature to the final prediction, LRP allows us to gain insights into why a neural network made a particular decision. This interpretability is crucial in areas where transparency and accountability are of utmost importance.
Here are a few key points to help you grasp the essence of Layer-Wise Relevance Propagation:
- Relevance Propagation: LRP involves propagating relevance values from the output layer back to the input layer of a neural network. This propagation process helps in identifying the features or neurons that strongly influenced the final prediction.
- Layer-by-Layer Analysis: Layer-Wise Relevance Propagation operates in a step-by-step manner, analyzing the contributions at each layer of the network. By dissecting the model layer by layer, LRP enables us to understand which features or neurons played a significant role at each step of the decision-making process.
- Deep Learning Clarity: Neural networks, particularly deep learning models, can be seen as black boxes due to their complex nature. Layer-Wise Relevance Propagation unravels this black box by shedding light on the features that strongly influenced the final decision. This clarity aids in building trust and understanding the underlying mechanisms of neural networks.
In summary, Layer-Wise Relevance Propagation is an essential tool in the field of machine learning that brings transparency and interpretability to complex neural network models. By attributing relevance values to individual features or neurons, LRP allows us to understand the reasoning behind a model’s decision-making process. This technique is vital for diverse industries, opening doors to increased trust, accountability, and reliable decision-making systems.
We hope this article has shed light on the fascinating world of Layer-Wise Relevance Propagation. Stay tuned for more enlightening discussions in our “DEFINITIONS” series!