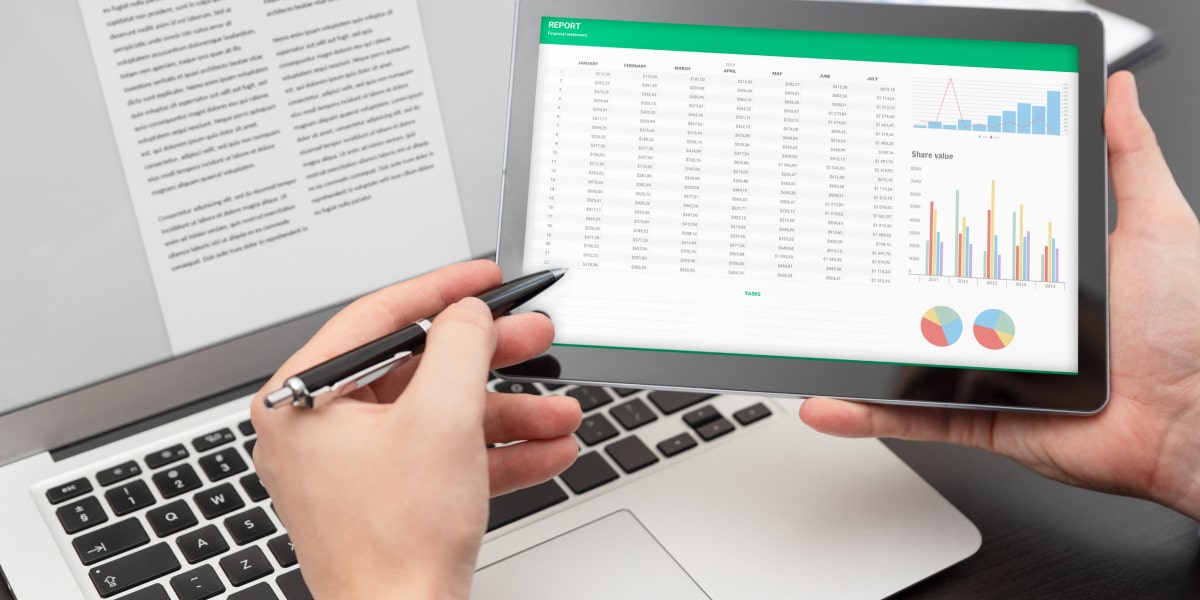
Are you interested in finding a lucrative way to make money from the comfort of your own home? Look no further than data mining! With the advancement of technology and the increasing amount of data available, data mining has become a valuable skill in the digital age. Whether you are a seasoned data analyst or a curious beginner, data mining offers opportunities to not only extract valuable insights but also generate income. In this article, we will explore the world of data mining and how you can leverage this skillset to make money. From understanding the basics of data mining to exploring various methods and platforms, we will provide you with a comprehensive guide to help you kickstart your journey towards financial success. So, let’s dive in and discover the exciting world of data mining!
Inside This Article
- Understanding Data Mining
- Choosing the Right Data Mining Techniques
- Collecting and Preparing Data
- Analyzing Data
- Interpreting and Extracting Insights
- Monetizing from Data Mining
- Conclusion
- FAQs
Understanding Data Mining
Data mining is a critical process in extracting valuable insights and patterns from a large set of data. It involves the use of advanced algorithms and techniques to discover hidden relationships and trends, enabling businesses to make informed decisions.
At its core, data mining is about uncovering patterns and knowledge from raw data. It goes beyond simple data analysis by using sophisticated techniques to unearth valuable information that may not be immediately apparent.
Data mining can be applied to various industries, including marketing, finance, healthcare, and manufacturing, among others. It allows businesses to understand customer behavior, identify market trends, optimize processes, and predict future outcomes.
One of the primary goals of data mining is to transform raw and unstructured data into actionable insights. By analyzing large datasets, businesses can gain a deeper understanding of their operations and identify areas for improvement.
Data mining encompasses a range of techniques, including association rule mining, classification, clustering, and anomaly detection. Each technique serves a specific purpose and helps businesses in different domains extract meaningful information.
Association rule mining identifies relationships between different variables in a dataset, enabling businesses to understand how certain factors influence one another. Classification techniques, on the other hand, categorize data into predefined classes or categories based on specific criteria.
Clustering algorithms group similar data points together, allowing businesses to segment their customer base or identify distinct patterns in their datasets. Anomaly detection methods, on the contrary, detect unusual or abnormal data points that deviate from the norm.
By understanding the fundamentals of data mining and its various techniques, businesses can leverage their data to gain a competitive advantage. With the ability to extract valuable insights from their datasets, they can make data-driven decisions and optimize their operations.
Furthermore, data mining allows businesses to identify patterns and trends that may not be immediately apparent, providing them with a deeper understanding of their customers and markets. This information can be used to develop targeted marketing campaigns, improve customer satisfaction, and drive revenue growth.
Overall, data mining is a powerful tool that enables businesses to extract valuable knowledge from their data. By analyzing and interpreting large datasets, they can uncover hidden patterns, make informed decisions, and ultimately achieve success in their respective industries.
Choosing the Right Data Mining Techniques
Data mining techniques play a crucial role in extracting meaningful insights from vast amounts of data. With the abundance of data available, it is essential to select the right techniques to ensure accurate and valuable results. In this section, we will explore the key considerations when choosing data mining techniques.
Identify your objective: The first step in selecting data mining techniques is to clearly define your objective. Determine what specific information or insights you want to obtain from your data analysis. Are you looking to make predictions, identify patterns, or discover associations? By understanding your objective, you can narrow down the techniques that align with your goals.
Understand your data: Familiarize yourself with the characteristics of your data, such as its size, structure, and type. Consider whether your data is structured, where it is organized in a consistent format, or unstructured, where it may exist in the form of text documents, images, or videos. This understanding will guide you in selecting techniques that are suitable for handling your data.
Determine the complexity: Data mining techniques vary in their complexity and computational requirements. Some techniques, like decision trees or clustering algorithms, are relatively simple and can be easily implemented. On the other hand, techniques like neural networks or support vector machines are more complex and may require additional computational resources. Assess the level of complexity that aligns with your data and available resources.
Consider the scalability: Scalability is an important factor to consider when choosing data mining techniques. If you anticipate working with large datasets or expect your data to grow in the future, select techniques that can handle the volume of data efficiently. Techniques like parallel processing or distributed computing can help improve scalability and speed up the analysis process.
Evaluate the appropriateness: Different data mining techniques excel in different scenarios. Evaluate each technique’s strengths and weaknesses to determine its suitability for your specific data and objectives. For example, if you are looking to uncover hidden patterns in your data, association rule mining techniques may be more appropriate, whereas if you need to make predictive models, regression or decision tree techniques may be better suited.
Consider interpretability: Depending on your needs, the interpretability of the results may be crucial. Some techniques, such as decision trees or logistic regression, provide easily interpretable models, allowing you to understand the underlying factors that influence the results. Others, like neural networks or support vector machines, may produce more accurate predictions but lack interpretability. Factor in the trade-off between interpretability and predictive power when selecting techniques.
Stay updated: The field of data mining is constantly evolving, with new techniques emerging and existing techniques being improved. Stay updated with the latest developments and advancements in data mining to ensure you are utilizing the most effective and efficient techniques available. Regularly explore research papers, attend conferences, and engage in online communities to stay abreast of the latest trends in the field.
By considering these factors, you can choose the right data mining techniques that align with your objectives, data characteristics, available resources, and desired level of interpretability. Selecting the appropriate techniques will enable you to extract valuable insights from your data and make informed decisions for your business or research.
Collecting and Preparing Data
Data collection is an essential step in the data mining process. To effectively gather relevant data, you need to have a clear understanding of your objectives and the specific information you are seeking. With cell phones becoming an integral part of our lives, there is a wealth of valuable data that can be collected for analysis and insights.
One common method of data collection is through surveys or questionnaires. These can be conducted online or in-person, targeting cell phone users to gather information about their preferences, usage patterns, and satisfaction levels. It is important to design well-structured and unbiased surveys to ensure accurate data.
Besides surveys, another way to collect data is by leveraging the power of social media. By scraping social media platforms, you can access a wealth of user-generated content about cell phone brands, features, and reviews. This data can provide valuable insights into customer sentiment and trends.
Web scraping is another effective technique for data collection. By crawling websites that contain relevant information, you can extract data on cell phone specifications, pricing, and user reviews. This information can be used for market analysis and competitor research.
Once the data is collected, it needs to be prepared for analysis. This involves several steps, including data cleaning, integration, and transformation. Data cleaning ensures that the collected data is accurate and reliable by removing duplicate entries, correcting errors, and handling missing values.
Data integration involves merging data from various sources into a single, unified dataset. This is crucial when dealing with data collected from different surveys, social media platforms, or websites. By integrating the data, you can gain a comprehensive view of the cell phone market and user preferences.
Data transformation includes converting the data into a usable format for analysis. This may involve reformatting data types, aggregating data at different levels, or creating new variables based on existing data. The goal is to transform the data into a structured format that can be easily analyzed and interpreted.
Overall, collecting and preparing data is a crucial step in the data mining process. It lays the foundation for accurate and meaningful analysis, enabling you to extract valuable insights and make informed decisions in the world of cell phones.
Analyzing Data
Once you have collected and prepared your data, the next crucial step in the data mining process is analyzing the data. This step involves exploring the data, identifying patterns, and gaining insights that can be used to make informed decisions and drive business growth.
There are various techniques and tools available to analyze data, depending on the nature of the data and the objectives of your analysis. Let’s take a look at some key approaches:
- Descriptive Analysis: This method involves summarizing and visualizing the data to understand its characteristics, such as mean, median, mode, standard deviation, and distribution. Descriptive analysis provides a high-level overview of the data.
- Diagnostic Analysis: This approach involves identifying the factors or variables that contribute to a particular outcome or event. It helps to understand the relationship between different variables and uncover the reasons behind specific patterns or trends in the data.
- Predictive Analysis: Using historical data, predictive analysis aims to forecast future trends or outcomes. It utilizes statistical algorithms and machine learning techniques to build models that can make predictions based on patterns and relationships found in the data.
- Prescriptive Analysis: This type of analysis goes beyond prediction and provides recommendations on what actions to take to achieve desired outcomes. It considers various constraints and objectives to suggest optimal solutions.
- Text and Sentiment Analysis: Text mining techniques can be used to extract insights from unstructured text data, such as customer reviews or social media posts. Sentiment analysis can help understand customer opinions and attitudes towards products or services.
During the analysis phase, it is essential to prioritize information that is relevant to your business objectives. This involves filtering the data and focusing on key variables or patterns that are most meaningful for your analysis.
Additionally, data visualization plays a crucial role in analyzing and presenting the findings. Visualizing data in the form of charts, graphs, or interactive dashboards makes it easier to understand patterns, spot outliers, and communicate insights effectively.
Remember, the analysis is not a one-time process. It is an iterative and ongoing activity that requires continuous monitoring and updating as new data becomes available. Regular analysis helps identify emerging trends and opportunities, enabling you to stay ahead in a rapidly changing business landscape.
By effectively analyzing your data, you can uncover valuable insights that can drive strategic decision-making, improve operational efficiency, and ultimately lead to business success.
Interpreting and Extracting Insights
Once you have collected and analyzed your data, the next step in the data mining process is interpreting and extracting insights from the findings. This crucial step involves analyzing patterns, trends, and correlations to gain valuable insights that can inform decision-making and drive business growth.
Interpreting the data requires a deep understanding of the context and domain knowledge. It’s important to consider the specific objectives of your data mining project and align the findings with your business goals. By doing so, you can extract meaningful insights that have a direct impact on your bottom line.
One of the key techniques used in interpreting data is data visualization. By presenting the findings in a visually appealing and easily understandable format, you can effectively communicate the insights to stakeholders and facilitate informed decision-making. Visualizing the data allows you to uncover hidden patterns, identify outliers, and spot trends that may not be apparent through raw data alone.
When interpreting the data, it’s important to ask the right questions. This involves digging deeper into the findings and exploring the factors that drive certain patterns or correlations. By asking insightful questions, you can uncover the underlying causes behind the data and gain a more comprehensive understanding of the situation.
Another important aspect of interpreting data is validating the findings and checking for any errors or biases. This involves conducting rigorous testing and evaluation to ensure the accuracy and reliability of the insights. It’s important to be aware of any limitations or potential biases in the data and address them appropriately to ensure the validity of the findings.
Once you have interpreted the data, the next step is to extract actionable insights. These insights are often used to guide decision-making and drive business strategies. Extracting insights involves distilling the complex findings into concise and actionable recommendations that can be easily understood and implemented.
It’s essential to communicate the extracted insights effectively to the relevant stakeholders. This involves presenting the findings in a clear and concise manner, highlighting the key takeaways, and explaining the implications for the business. By effectively communicating the insights, you can ensure that they are understood and acted upon by the decision-makers.
Extracting insights from data mining is an iterative process. As you gain more insights, it’s important to continue refining and updating your analysis to uncover deeper insights and improve decision-making. By continuously interpreting and extracting insights, you can drive continuous improvement and stay ahead in today’s data-driven landscape.
Overall, interpreting and extracting insights from data mining is a critical step in the data analysis process. By effectively analyzing the data, asking the right questions, and extracting actionable insights, businesses can make informed decisions and drive growth. It’s important to approach this step with a combination of domain expertise, analytical skills, and effective communication to unlock the true potential of your data.
Monetizing from Data Mining
Data mining, the process of extracting valuable insights and patterns from large datasets, has become an invaluable tool for businesses in today’s data-driven world. While data mining is primarily used to gain a deeper understanding of customer behavior, market trends, and operational efficiency, it also presents opportunities for monetization. In this section, we will explore some strategies and methods for monetizing from data mining.
1. Data-driven Decision Making: One of the most effective ways to monetize data mining is by using the insights gained to make informed business decisions. By leveraging the patterns and correlations discovered through data mining, companies can optimize their operations, improve customer targeting, and enhance their overall decision-making processes. These improvements can lead to increased efficiency and cost savings, ultimately resulting in higher profitability.
2. Customer Segmentation and Targeted Marketing: Data mining provides businesses with the ability to segment their customers based on various criteria such as demographics, interests, purchasing behavior, and more. This segmentation allows companies to tailor their marketing efforts and promotional offers to specific customer groups, increasing the chances of conversion and maximizing the return on investment. By utilizing data mining to optimize customer segmentation and targeted marketing campaigns, businesses can increase revenue and profitability.
3. Personalized Recommendations: E-commerce platforms, streaming services, and content providers can monetize data mining by offering personalized recommendations to their users. By analyzing user behavior and preferences, data mining algorithms can suggest products, movies, music, or content that align with the individual’s interests. This personalized approach enhances the user experience, increases engagement, and drives sales or subscriptions, leading to increased revenue for the platform.
4. Data Monetization and Licensing: In some cases, companies can directly monetize their data by licensing it to third parties. Companies with valuable datasets, such as market research firms or social media platforms, can sell access to their data to other businesses for analysis and insights. This data can be used by other organizations to enhance their decision-making processes or develop new products and services. Data monetization through licensing can be a lucrative revenue stream for companies with rich and high-quality datasets.
5. Data-Driven Products and Services: Data mining can also be used to develop and monetize data-driven products and services. For example, companies can create predictive analytics tools, recommendation engines, or fraud detection systems based on their data mining capabilities. These products and services can be offered to other businesses or directly to consumers, providing an additional revenue stream and establishing the company as a leader in the data analytics space.
6. Data Monetization Partnerships: Another approach to monetizing data mining is through strategic partnerships. Companies can collaborate with other businesses, such as analytics firms or technology providers, to leverage their data mining expertise and develop joint offerings. By combining resources and expertise, both parties can create innovative solutions, reach new markets, and generate additional revenue from data mining-driven products or services.
Conclusion
In conclusion, data mining is a powerful tool that can help individuals and businesses unlock valuable insights and opportunities in the digital age. By harnessing the power of data, you can make informed decisions, uncover hidden patterns, and optimize processes to drive success.
Whether you’re an individual looking to generate income from data mining or a business seeking a competitive edge, it’s important to approach this field with the right skills and mindset. Be sure to stay updated with the latest techniques, tools, and industry trends to stay ahead.
Data mining is a journey that requires continuous learning and adaptation. With the right strategies, resources, and dedication, you can leverage this technology to increase your earning potential and unlock new financial opportunities.
So go ahead, dive into the world of data mining, and pave your way to financial success!
FAQs
1. Can I make money data mining?
Yes, data mining can be a profitable endeavor if done correctly. By extracting valuable insights from large datasets, businesses can make informed decisions and gain a competitive edge in their respective markets.
2. How do I get started with data mining?
To get started with data mining, you will need a solid understanding of statistical analysis, machine learning algorithms, and programming languages such as Python or R. There are various online courses and resources available to help you develop the necessary skills.
3. What types of data can be mined?
You can mine various types of data, including structured, semi-structured, and unstructured data. Structured data includes information stored in databases or spreadsheets, while semi-structured data includes data in formats like XML or JSON. Unstructured data, such as social media posts or customer reviews, can also be mined for valuable insights.
4. Is data mining legal?
Data mining is generally legal as long as it is done in compliance with privacy laws and regulations. It is important to obtain proper consent and ensure the ethical use of data during the mining process.
5. What are some common applications of data mining?
Data mining has a wide range of applications across industries. Some common applications include customer segmentation, fraud detection, market basket analysis, sentiment analysis, and predictive modeling. These applications can help businesses optimize their operations and make data-driven decisions.