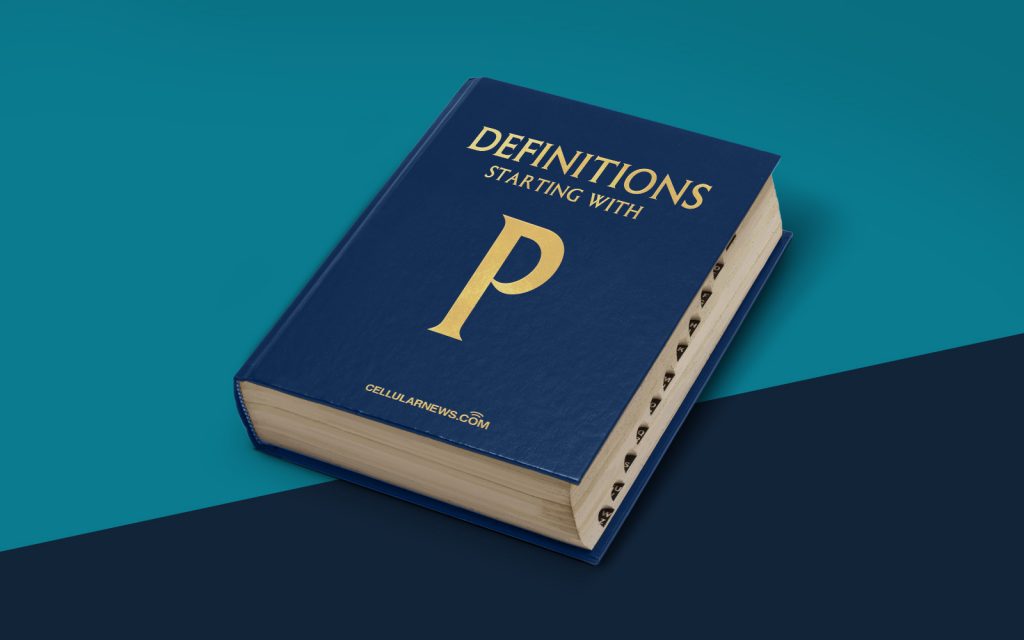
What is Predictive Modeling?
Welcome to the “DEFINITIONS” category on our page! In this post, we will dive into the fascinating world of predictive modeling and demystify this often misunderstood concept. So, sit back, relax, and let’s explore what predictive modeling is all about!
Predictive modeling is a powerful analytical technique used in data science to forecast future outcomes based on historical data. It involves building a mathematical model that captures patterns and relationships between variables, enabling you to make informed predictions about future events or trends.
Key Takeaways:
- Predictive modeling is an analytical technique used to forecast future outcomes based on historical data.
- It involves building a mathematical model that captures patterns and relationships between variables.
Now that we have a general understanding of what predictive modeling entails, let’s delve deeper into the process. Here are the key steps involved:
- Data Collection: The first step in predictive modeling is gathering and organizing relevant data. This can include historical records, customer information, market trends, or any other data that might be valuable in predicting the desired outcome.
- Data Preprocessing: Once the data is collected, it needs to be cleaned and prepared for analysis. This involves removing outliers, dealing with missing values, and transforming variables to ensure the data is in a suitable format for modeling.
- Feature Selection and Engineering: In this step, relevant features (variables) that are most likely to have an impact on the outcome are selected. It may also involve creating new features by combining existing ones or extracting meaningful information.
- Model Development: This is where the magic happens! Various modeling techniques like regression, classification, or machine learning algorithms are applied to analyze the data and build a predictive model.
- Model Evaluation and Validation: Once the model is developed, it needs to be tested and evaluated to ensure its accuracy and reliability. This is done by using unseen data or cross-validation techniques.
- Prediction and Deployment: After the model’s performance is deemed satisfactory, it is ready to be deployed in real-world scenarios, where it can be used to make predictions and provide valuable insights.
Predictive modeling has a wide range of applications across various industries. From predicting customer behavior, demand forecasting, fraud detection, to medical diagnosis and risk assessment, the possibilities are endless.
So, why should you care about predictive modeling? Here are two key takeaways to remember:
- Enhanced Decision-Making: Predictive modeling empowers businesses to make data-driven decisions, leading to improved efficiency and accuracy in forecasting future outcomes.
- Competitive Edge: By leveraging predictive modeling, companies gain a competitive advantage by identifying opportunities, mitigating risks, and staying ahead of the curve in a rapidly changing market.
And there you have it! A comprehensive overview of what predictive modeling is all about. We hope this blog post has demystified the concept and highlighted its importance in various industries. Now you can impress your friends by explaining this complex analytical technique!
Stay tuned for more exciting definitions in our “DEFINITIONS” category. Until then, happy modeling!