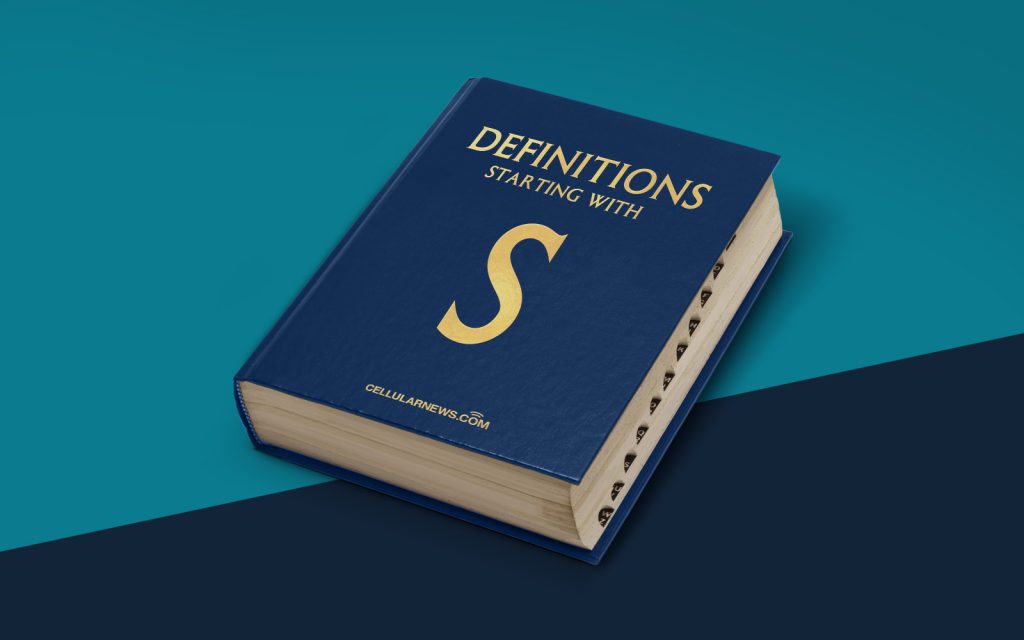
Welcome to the World of Synthetic Data!
Have you ever wondered what synthetic data is and how it is changing the way we approach data analysis? Well, you’re in the right place! In this article, we will explore the concept of synthetic data and its importance in various fields. So, buckle up and let’s dive into the fascinating world of synthetic data!
Key Takeaways:
- Synthetic data is artificially generated data that mimics real-world data patterns.
- It helps protect sensitive information while providing privacy-preserving and legally-compliant datasets for analysis.
What is Synthetic Data?
Synthetic data refers to artificially generated data that imitates real-world data patterns, while ensuring that no sensitive or identifiable information is included. It is created using algorithms that replicate the statistical properties, such as distributions and correlation, of the original data. Essentially, synthetic data serves as a substitute for real data, enabling researchers, developers, and analysts to work with privacy-preserving and legally-compliant datasets.
Why is Synthetic Data Important?
Now that we know what synthetic data is, let’s explore its significance:
- Data Privacy: In today’s data-driven world, preserving privacy is crucial. Synthetic data allows organizations to share and analyze data without compromising the privacy of individuals or violating data protection regulations.
- Cost and Accessibility: Generating synthetic data is significantly more cost-effective and accessible than gathering real-world data. It eliminates the need for expensive data collection processes and reduces the risk associated with handling sensitive information.
- Model Testing and Development: Synthetic data provides a boon for researchers and developers by allowing them to validate and fine-tune models without using real data. This accelerates the development phase and helps identify any potential issues or biases before applying the model to real-world scenarios.
- Data Diversity: Synthetic data allows for the creation of diverse datasets by manipulating various parameters. Researchers and analysts can generate data with different demographics, variations, or scenarios to study the impact on models and algorithms.
- Machine Learning: Synthetic data plays a vital role in training machine learning models. By expanding the size and diversity of training datasets, it enhances the model’s ability to generalize and perform well in real-world situations.
Synthetic data opens up a world of possibilities and solutions across various sectors, including healthcare, finance, transportation, and more. Its applications are broad, ranging from fraud detection and predictive analytics to driverless car testing and medical research. With its ability to ensure privacy and accessibility, synthetic data is revolutionizing the way we handle and analyze data in the digital era.
So, whether you’re a data scientist, developer, or analyst, embracing the power of synthetic data can help you unlock remarkable insights while upholding privacy and compliance. It’s time to embrace this innovative approach and explore the endless possibilities that synthetic data has to offer!