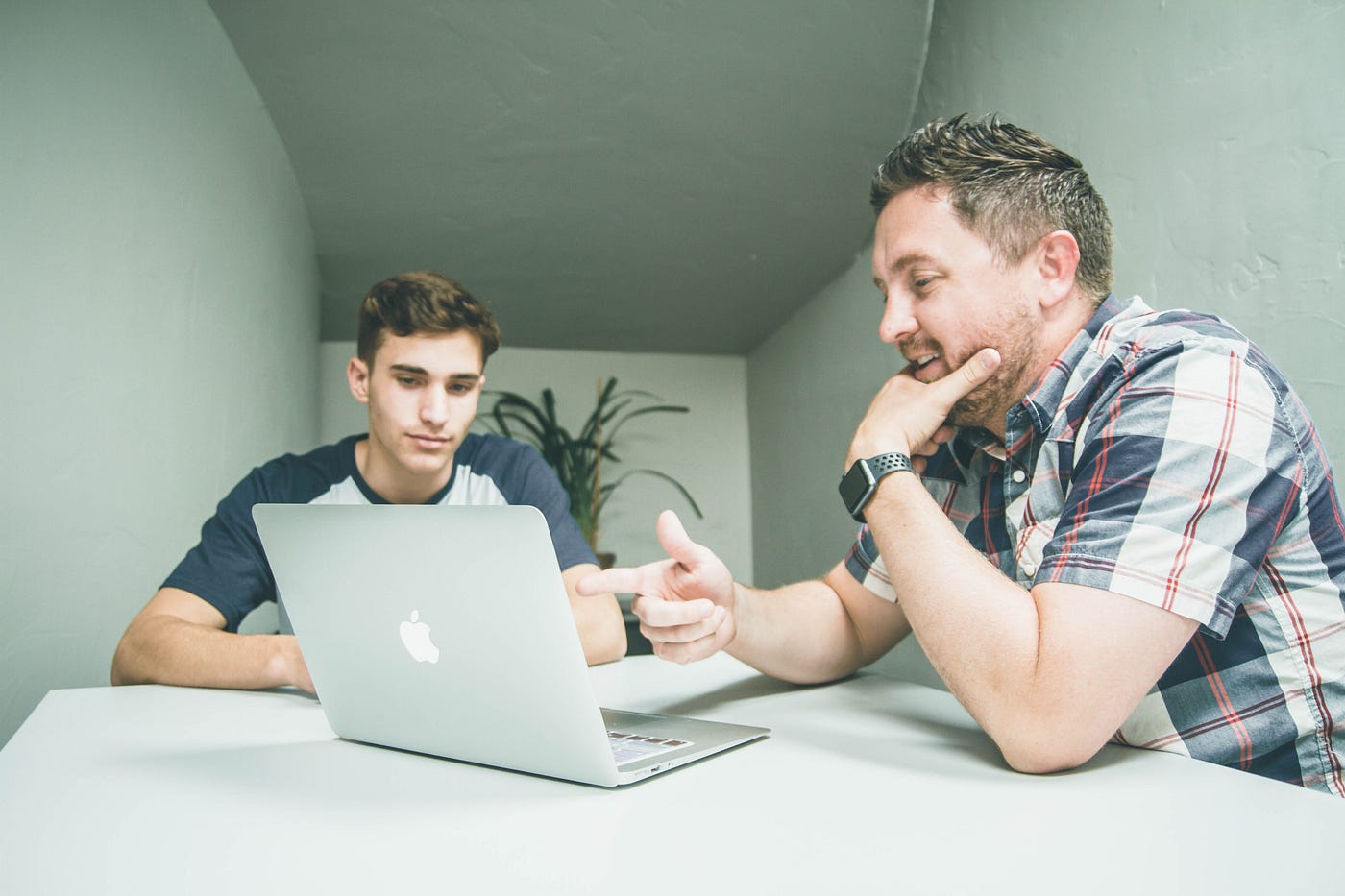
Are you fascinated by the world of data science and considering a career as a data scientist? One of the most common questions aspiring data scientists have is, “How long does it take to become a data scientist?” The answer to this question can vary depending on individual circumstances and the level of expertise you aim to achieve. While some people may be able to enter the field relatively quickly, others may require a more extensive education and practical experience. In this article, we will explore the different factors that can influence the time it takes to become a data scientist, including educational background, prior knowledge, and the learning resources available. So, if you’ve been wondering about the journey to becoming a data scientist, read on to discover the key aspects and timeframes to keep in mind.
Inside This Article
- The Importance of Educational Background in Becoming a Data Scientist
- The Role of Practical Experience in Becoming a Data Scientist
- The Timeframe for Mastering Technical Skills as a Data Scientist
- The Continuous Learning Process in the Journey of Becoming a Data Scientist
- Conclusion
- FAQs
The Importance of Educational Background in Becoming a Data Scientist
When it comes to pursuing a career as a data scientist, having a strong educational background is of great importance. While there is no specific degree requirement to become a data scientist, a solid educational foundation can give you a competitive edge in this rapidly evolving field.
One of the key benefits of a formal education in data science or a related field is the acquisition of fundamental knowledge and skills. The coursework typically covers essential topics such as mathematics, statistics, programming, and data analysis techniques. These foundational concepts serve as building blocks for more advanced data science practices.
Obtaining a degree in data science, computer science, statistics, or a similar field not only equips you with the necessary technical skills but also provides a theoretical understanding of data science concepts. This theoretical knowledge enables you to understand the underlying principles behind algorithms, models, and statistical methods, allowing you to apply them effectively in real-world scenarios.
Furthermore, pursuing a degree in data science often provides opportunities for hands-on experience through practical projects and internships. These experiences allow you to work with real datasets, apply analytical techniques, and gain practical problem-solving skills. Employers value this practical experience, as it demonstrates your ability to apply your knowledge in real-world contexts.
In addition to the technical skills and theoretical understanding, a strong educational background can also provide you with critical thinking and analytical skills. Data scientists are constantly faced with complex problems and vast amounts of data, and the ability to think critically and analytically is crucial in extracting meaningful insights from the data.
While having a formal education can greatly enhance your chances of becoming a data scientist, it is important to note that continuous learning and staying up-to-date with the latest advancements are equally important. The field of data science is ever-evolving, and new tools, techniques, and methodologies emerge regularly. Therefore, it is essential to have a strong foundation that allows you to adapt and learn throughout your career as a data scientist.
The Role of Practical Experience in Becoming a Data Scientist
When it comes to becoming a successful data scientist, there’s no denying the crucial role that practical experience plays. While education and theoretical knowledge are important, it is through practical application that aspiring data scientists refine their skills and truly grasp the intricacies of their field.
Practical experience allows data scientists to put their knowledge into action and gain invaluable insights by working with real-world data sets. It helps in understanding the challenges, limitations, and nuances of working with data in practical scenarios.
One of the key benefits of practical experience is the ability to learn from mistakes. Data scientists often encounter unexpected issues and obstacles when working with real data, and it is through these challenges that they gain valuable problem-solving skills. By analyzing and troubleshooting these issues, data scientists develop a deeper understanding of the data and the tools they use.
Another significant role of practical experience is developing domain expertise. As data scientists work on projects in specific industries or domains, they gain valuable knowledge and insights that are crucial for understanding the underlying context of the data. This domain expertise allows data scientists to better interpret and analyze the data, resulting in more accurate and actionable insights.
Furthermore, practical experience provides data scientists with the opportunity to work in cross-functional teams. Collaborating with professionals from different backgrounds such as business analysts, software developers, and domain experts enhances their communication and teamwork skills. This interdisciplinary collaboration enables data scientists to gain a broader perspective on problem-solving and fosters a more holistic approach to data analysis.
It is important to note that practical experience can be gained through various means. For example, internships, freelance projects, or personal projects can all contribute to building practical experience. Additionally, participating in data science competitions or contributing to open-source projects are great ways to gain exposure and improve practical skills.
The Timeframe for Mastering Technical Skills as a Data Scientist
Becoming a data scientist requires a solid understanding of technical skills, such as programming languages, statistical analysis, machine learning, and data visualization. But how long does it take to master these skills? The timeframe for mastering technical skills as a data scientist varies depending on various factors, including one’s prior knowledge, dedication, learning resources, and practice.
For someone starting from scratch, it may take around six months to a year to become proficient in the foundational skills required for a data scientist. This includes learning programming languages like Python or R, understanding data manipulation and cleaning, and getting familiar with statistics and mathematical concepts.
As the journey progresses, aspiring data scientists delve deeper into more advanced concepts and techniques. This could involve mastering machine learning algorithms, building predictive models, and exploring big data frameworks. This intermediate stage may take an additional year or two, depending on the depth of knowledge one aims to achieve.
Keep in mind that mastery is a continual process, and the field of data science is constantly evolving. Data scientists must stay updated with the latest techniques, technologies, and advancements in the industry. This requires an ongoing investment of time and effort in continuous learning and professional development.
However, it’s important to note that the timeframe for mastering technical skills as a data scientist is not set in stone. Each individual’s learning curve is different, and some may grasp concepts faster than others. The key is to set realistic goals, stay consistent with learning, and embrace a growth mindset.
Ultimately, becoming a proficient data scientist is a combination of theoretical knowledge, practical experience, and continuous learning. It’s essential to not only focus on technical skills but also develop a strong problem-solving ability, critical thinking, and effective communication skills.
The Continuous Learning Process in the Journey of Becoming a Data Scientist
Becoming a data scientist is not a one-time achievement but a continuous journey of learning and growth. In the rapidly evolving field of data science, staying up-to-date with the latest advancements, techniques, and tools is crucial. As data scientists, we are constantly faced with new challenges, emerging technologies, and changing business requirements that require us to continuously enhance our skills and knowledge.
One of the key aspects of the continuous learning process is keeping abreast of the latest industry trends and research. This involves reading research papers, attending conferences and webinars, and participating in online forums and communities. By staying connected with the broader data science community, we can gain valuable insights, engage in discussions, and learn from the experiences and expertise of others.
Another important aspect of the continuous learning process is experimenting with new techniques and tools. Data science is a field that thrives on innovation and experimentation. As data scientists, we are constantly exploring different algorithms, models, and frameworks to find the most effective solutions for the problems we encounter. By being open to trying new approaches and methodologies, we can expand our skill set and stay ahead in the ever-changing data science landscape.
Mentorship and collaboration also play a vital role in the continuous learning process. By seeking guidance from experienced data scientists and working on projects with colleagues, we can learn from their expertise and gain valuable practical knowledge. Sharing ideas and collaborating with others fosters a culture of learning and growth, allowing us to learn from different perspectives and approaches.
Furthermore, continuous learning involves honing our technical skills. Data science requires proficiency in programming languages like Python and R, as well as in-depth knowledge of statistics, data visualization, machine learning, and data manipulation techniques. Regular practice, taking online courses, attending workshops, and participating in hands-on projects are effective ways to improve our technical skills and stay ahead of emerging trends.
Lastly, a growth mindset is essential to the continuous learning process. Embracing challenges, seeking feedback, and persistently working on improving our skills are key characteristics of a successful data scientist. The field of data science is constantly evolving, and being adaptable and willing to learn from failures and setbacks is crucial in this journey.
Conclusion
In conclusion, becoming a data scientist is not an overnight process. It requires dedication, continuous learning, and practical experience. The time it takes to become a data scientist can vary depending on various factors such as prior knowledge, educational background, level of commitment, and the resources available. However, with a structured approach and the right resources, one can expect to embark on a data science career within a year or two.
It is important to remember that becoming a data scientist is a journey rather than a destination. Even after entering the field, professionals in this domain need to stay updated with the latest techniques, tools, and algorithms to keep up with the evolving industry. Continuous learning and staying curious are crucial to excel in this field.
So, if you are considering a career in data science, take the plunge and embark on this exciting and rewarding journey. With the right mindset, perseverance, and a passion for solving complex problems, you can thrive in the world of data science.
FAQs
FAQ 1: What is a data scientist?
A data scientist is a professional who collects, analyzes, and interprets complex data to help businesses make data-driven decisions. They utilize their expertise in mathematics, statistics, and programming to extract insights from large datasets and create models and algorithms to solve real-world problems.
FAQ 2: How long does it take to become a data scientist?
The time it takes to become a data scientist can vary depending on various factors like prior knowledge, educational background, and the learning path you choose. On average, it takes about 2-4 years to acquire the necessary skills and experience to become a proficient data scientist.
FAQ 3: What qualifications or education do I need to become a data scientist?
While there is no fixed educational requirement to become a data scientist, a strong foundation in mathematics, statistics, and computer science is essential. Most data scientists have a bachelor’s or master’s degree in a relevant field like mathematics, statistics, computer science, or data science. However, it is also possible to enter the field through online courses, bootcamps, and self-study.
FAQ 4: What skills are required to become a data scientist?
To become a successful data scientist, you need a combination of technical and soft skills. Technical skills include proficiency in programming languages like Python and R, knowledge of statistical analysis and machine learning algorithms, data visualization, and working with big data tools. Soft skills like critical thinking, problem-solving, communication, and the ability to work in a team are also important for a data scientist.
FAQ 5: How can I start my journey to become a data scientist?
To start your journey to become a data scientist, it is advisable to begin with a strong foundation in mathematics and statistics. Familiarize yourself with programming languages like Python or R. Explore online resources, such as tutorials and courses, to learn about data science concepts and techniques. Practice on real-world datasets and actively participate in data science communities to gain practical experience and grow your network.