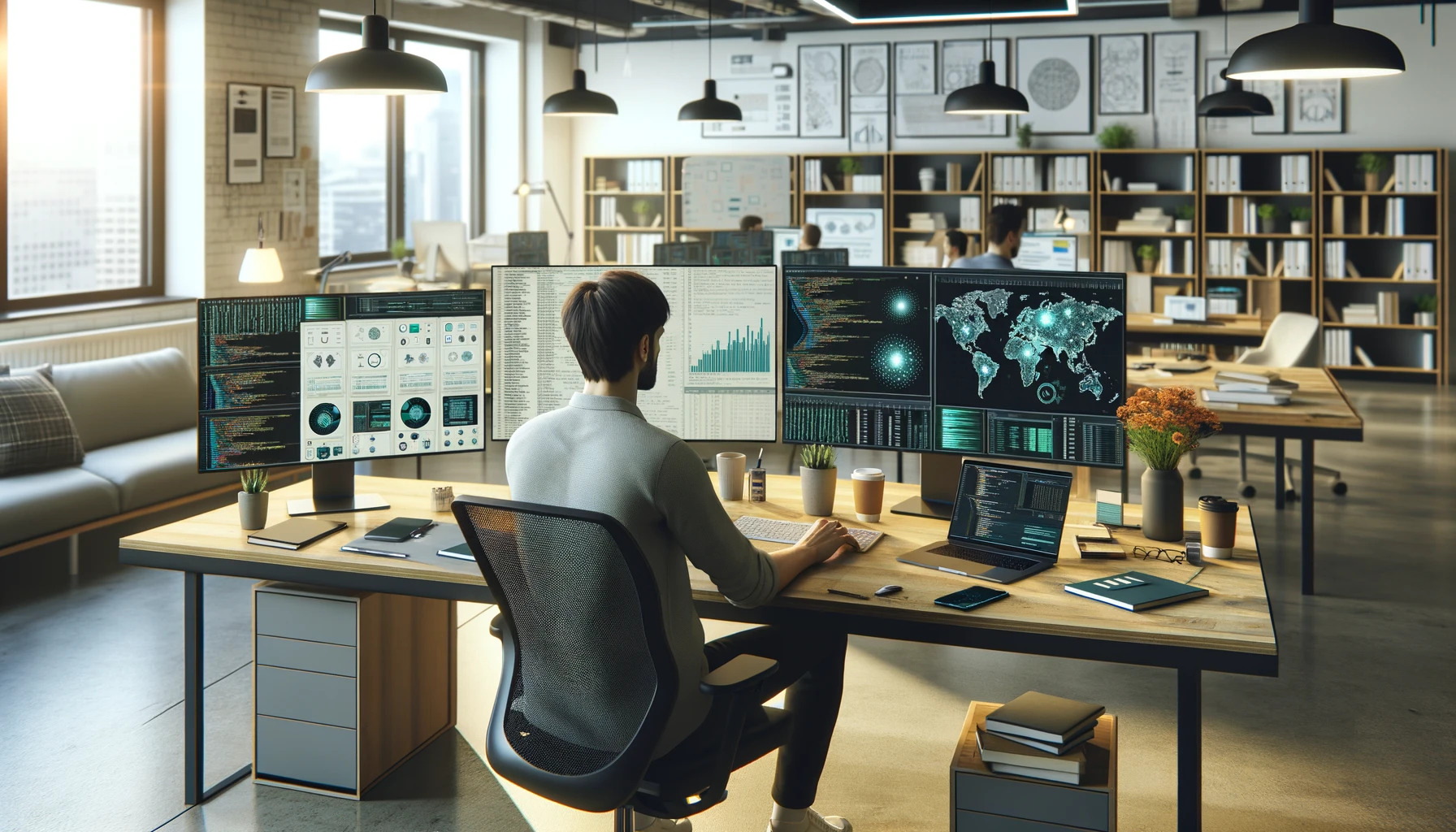
In today’s data-driven world, hiring a data scientist has become crucial for businesses looking to gain a competitive edge. A data scientist is a skilled professional who possesses the expertise to analyze complex datasets, extract valuable insights, and make data-driven decisions. With the increasing demand for data-driven strategies, finding the right data scientist can be a daunting task.
However, by following a strategic approach and understanding the key factors to consider, businesses can effectively hire a data scientist who meets their requirements. In this article, we will delve into the essential steps you need to take to hire a data scientist, from defining your hiring needs to evaluating candidates’ technical skills and assessing their cultural fit within your organization.
Inside This Article
- Understanding the Role of a Data Scientist
- Defining the Required Skills and Qualifications
- Sourcing and Screening Candidates
- Conducting Technical Interviews
- Evaluating Candidates’ Problem-Solving Abilities
- Assessing Communication and Collaboration Skills
- Checking References and Background
- Making the Final Decision
- Onboarding and Integration of the Data Scientist
- Conclusion
- FAQs
Understanding the Role of a Data Scientist
Data is increasingly becoming a valuable asset for businesses across industries. Harnessing the power of data requires skilled professionals who can extract insights, make data-driven decisions, and uncover patterns and trends. This is where data scientists come in.
A data scientist is a multidisciplinary expert who uses their knowledge of mathematics, statistics, and programming to analyze complex datasets and derive meaningful insights. They possess a unique blend of technical skills and business acumen, enabling them to identify opportunities for optimization, create predictive models, and drive strategic decision-making.
At its core, the role of a data scientist involves collecting, organizing, and analyzing vast amounts of data to extract patterns, uncover correlations, and identify trends. They develop algorithms, build predictive models, and use machine learning techniques to generate actionable insights. These insights help businesses make informed decisions, improve processes, and achieve their objectives.
Data scientists also play a crucial role in data governance and data quality management. They ensure that data is accurately collected, stored, and maintained. They implement data cleaning techniques, handle missing data, and address data inconsistencies to ensure the reliability and accuracy of the analysis.
Moreover, data scientists are proficient in programming languages such as Python, R, or SQL, which they use to manipulate and analyze data. They are skilled in data visualization, using tools like Tableau or matplotlib to present their findings in a clear and concise manner. This enables stakeholders to understand the insights and make data-driven decisions.
Additionally, data scientists need to possess strong problem-solving and critical thinking abilities. They are experienced in formulating hypotheses, performing experiments, and iterating through various approaches to find optimal solutions. They are not only comfortable with handling complex datasets but also have a keen eye for detail and an analytical mindset.
Defining the Required Skills and Qualifications
When it comes to hiring a data scientist, it’s crucial to clearly define the required skills and qualifications for the role. Data science is a complex field that requires a unique combination of technical expertise, analytical thinking, and problem-solving skills. By clearly outlining the necessary qualifications, you can attract candidates who possess the right skill set and ensure a successful hiring process.
Here are some key skills and qualifications to consider when defining the requirements for a data scientist:
- Analytical Thinking: A data scientist should have excellent analytical thinking skills to be able to process and interpret large datasets. They should possess a deep understanding of statistical analysis and mathematical modeling to derive meaningful insights.
- Programming: Proficiency in programming languages such as Python, R, or SQL is essential for a data scientist. They should be able to write efficient and clean code to extract and manipulate data, create algorithms, and build predictive models.
- Machine Learning: In today’s data-driven world, knowledge of machine learning algorithms and techniques is highly desired. A data scientist should be comfortable implementing and fine-tuning algorithms like regression, random forest, or neural networks to develop accurate predictive models.
- Domain Knowledge: Depending on your industry or specific business needs, it may be important to seek a data scientist with domain knowledge. This understanding of the industry can help them better analyze and interpret data in context, leading to more meaningful insights and recommendations.
- Data Visualization: The ability to effectively communicate data-driven insights is crucial. Data scientists should be skilled in using data visualization tools and techniques to create clear and engaging visualizations that convey complex information in a digestible manner.
Additionally, soft skills such as strong communication, problem-solving, and collaboration abilities are also important for a data scientist’s success. They should be able to effectively communicate their findings to both technical and non-technical stakeholders, collaborate with cross-functional teams, and adapt to evolving project requirements.
By defining the required skills and qualifications, you can create a job description that attracts the right candidates and ensures a successful hiring process. It’s important to keep in mind that the specific skills and qualifications needed may vary depending on the goals of your organization and the nature of the data science projects to be undertaken.
Sourcing and Screening Candidates
When it comes to hiring a data scientist, the process of finding and selecting the right candidate can be challenging. Sourcing and screening candidates plays a crucial role in ensuring that you hire a highly skilled and qualified individual who can effectively handle the specific data challenges your company faces.
To begin with, you need to determine where and how to source potential candidates. Traditional methods such as job boards and industry-specific websites can be a starting point, but it’s also important to explore other avenues. Utilize professional networking platforms like LinkedIn or attend data science conferences to connect with potential candidates.
When screening resumes and applications, make sure to look for relevant experience, educational background, and technical skills. Look for experience in handling large datasets, proficiency in programming languages such as Python or R, and knowledge of machine learning algorithms and statistical analysis techniques.
In addition to technical qualifications, it’s essential to assess candidates’ ability to think critically and problem-solve. Look for evidence of project experience, research papers, or open-source contributions that demonstrate their analytical thinking and problem-solving abilities.
Consider implementing skill assessments or coding challenges to further evaluate candidates’ technical prowess. This can give you a clearer understanding of their ability to apply theoretical knowledge to practical data scenarios.
During the screening process, it’s important to consider cultural fit and how well candidates can collaborate with your existing data science team. Look for individuals who possess strong communication skills, as data scientists often need to effectively communicate complex concepts and findings to non-technical stakeholders.
Checking references and conducting thorough background checks are also crucial steps in the screening process. Reach out to previous employers or supervisors to gather insights about a candidate’s work ethic, problem-solving abilities, and overall performance.
Once you have narrowed down the pool of candidates, it’s time to conduct in-depth technical interviews. These interviews should focus on the candidates’ problem-solving abilities, analytical skills, and their ability to apply their knowledge to real-world scenarios.
By effectively sourcing and screening candidates, you increase your chances of hiring a data scientist who not only possesses the technical skills required for the role, but also fits well with your company culture and can contribute positively to your team.
Conducting Technical Interviews
When it comes to hiring a data scientist, conducting technical interviews is a crucial step in the process. This is where you get the chance to assess a candidate’s technical knowledge, problem-solving abilities, and analytical skills. To make the most of this interview, it’s important to have a well-structured and strategic approach. Here are some key factors to consider:
1. Prepare a Range of Technical Questions: Before the interview, take the time to create a comprehensive list of technical questions that cover various aspects of data science. These questions should assess the candidate’s knowledge of programming languages, statistical analysis, machine learning algorithms, data manipulation, and visualization techniques. It’s important to have a mix of theoretical and practical questions to gauge the candidate’s ability to apply their knowledge in real-world scenarios.
2. Set Up a Practical Coding Challenge: In addition to asking theoretical questions, it can be valuable to include a practical coding challenge as part of the interview process. This gives candidates the opportunity to demonstrate their programming skills and problem-solving abilities in real-time. Choose a challenge that aligns with the tasks they would encounter in their role, such as data cleaning, feature engineering, or building predictive models. Assess their coding style, efficiency, and understanding of best practices.
3. Evaluate the Thought Process: During the technical interview, it’s important to not only focus on the correct answer but also assess the candidate’s thought process. Encourage them to talk through their approach and explain their reasoning behind each step. This will give you insights into their analytical thinking, ability to break down complex problems, and attention to detail. Look for candidates who can articulate their thoughts clearly and logically.
4. Assess Domain Knowledge: Depending on the nature of your business or industry, it may be necessary to evaluate a candidate’s domain knowledge. Ask questions specific to your field to gauge their understanding and familiarity with relevant concepts, datasets, and challenges. This will help you assess how quickly they can adapt to your organization’s specific needs and contribute effectively to data-driven decision-making.
5. Create a Collaborative Environment: Data scientists often need to work collaboratively with teams and stakeholders. Use the technical interview as an opportunity to assess a candidate’s communication and teamwork skills. Include questions that require them to explain complex concepts to non-technical individuals or discuss their approach to working on a group project. A strong candidate will be able to communicate effectively, listen actively, and collaborate efficiently.
6. Provide Feedback: After each technical interview, take the time to provide constructive feedback to the candidate. This not only helps them understand their strengths and areas for improvement but also reflects your commitment to a fair and transparent hiring process. Be specific in your feedback, highlighting their technical competencies and suggesting areas where they can further develop their skills.
By following these guidelines, you can conduct effective technical interviews that provide valuable insights into a candidate’s abilities and compatibility with your organization. Remember, the goal is not just to hire a data scientist but to find the right fit who can contribute to your business’s success.
Evaluating Candidates’ Problem-Solving Abilities
When hiring a data scientist, one of the most crucial aspects to assess is their problem-solving abilities. Data scientists are tasked with analyzing complex datasets, identifying patterns, and developing innovative solutions to solve business challenges.
During the evaluation process, consider the following factors to gauge a candidate’s problem-solving skills:
- Technical Competency: Assess the candidate’s knowledge of data science algorithms, statistical modeling, machine learning techniques, and programming languages such as Python or R. Ask them to explain how they would approach different types of problems and the methodologies they would employ.
- Creativity: Look for candidates who can think outside the box and come up with unconventional solutions. Present them with hypothetical scenarios or real-world challenges and evaluate their ability to generate innovative ideas and approaches.
- Quantitative Analysis: Evaluate the candidate’s ability to analyze numerical data and draw meaningful insights. Assess their mathematical skills, statistical knowledge, and their proficiency in using data visualization tools to communicate findings effectively.
- Problem-Solving Process: Inquire about the candidate’s problem-solving process. Do they follow a systematic approach? Do they define the problem clearly, break it down into manageable steps, and iterate through different solutions? Look for candidates who demonstrate a structured and analytical approach.
- Communication: Assess how effectively the candidate can communicate their problem-solving process and findings to both technical and non-technical stakeholders. Look for clear and concise explanations, the ability to present complex concepts in a simplified manner, and effective storytelling skills.
It’s important to remember that problem-solving abilities are not solely determined by a candidate’s technical knowledge. Look for candidates who show curiosity, adaptability, and a growth mindset. They should be willing to learn new tools and techniques, explore different approaches, and continuously improve their problem-solving skills.
Lastly, consider providing candidates with a practical problem-solving exercise or a case study, to assess their abilities in an applied setting. This will give you a better understanding of their approach, methodology, and overall problem-solving capabilities.
By thoroughly evaluating a candidate’s problem-solving abilities, you can ensure that the data scientist you hire is equipped to tackle complex challenges and contribute to the success of your organization.
Assessing Communication and Collaboration Skills
When hiring a data scientist, assessing their communication and collaboration skills is crucial. Data scientists not only need to have strong technical abilities but also the ability to effectively communicate their findings and collaborate with different stakeholders within the organization. Here are some key strategies for evaluating a candidate’s communication and collaboration skills:
1. Interviewing Techniques: During the interview, ask the candidate about their past experiences working in teams and how they effectively communicated complex data-driven insights to non-technical stakeholders. Look for examples of projects where the candidate had to collaborate with others, handle conflicts, and ensure effective communication throughout the process.
2. Presentation Skills: Request the candidate to prepare and deliver a presentation on a relevant topic. This will allow you to assess their ability to convey complex information in a clear and concise manner. Pay attention to their presentation style, use of visuals, and how well they engage the audience.
3. Group Exercises: Organize interactive group exercises as part of the interview process. This will give you the opportunity to observe how the candidate interacts with others, listens to different perspectives, and contributes effectively to collaborative tasks.
4. References and Feedback: Contact references provided by the candidate and inquire about their communication and collaboration skills. Previous team members or managers can provide valuable insights into how the candidate interacts with others and handles collaborative projects.
5. Problem-Solving Scenarios: Present the candidate with hypothetical scenarios or case studies involving data-driven challenges that require collaboration. Evaluate how they approach these scenarios, their ability to communicate their thought processes, and their willingness to seek input from others.
6. Soft Skills Assessment: Consider incorporating personality assessments or behavioral interviews to gain a deeper understanding of a candidate’s communication and collaboration style. These assessments can help gauge their ability to work in diverse teams and adapt to different communication styles.
Assessing a candidate’s communication and collaboration skills is crucial for ensuring they can effectively communicate complex concepts, work well in cross-functional teams, and contribute to the overall success of your data-driven initiatives. By taking the time to evaluate these skills, you can ensure that you hire a data scientist who not only possesses technical expertise but also excels in collaborative and communicative abilities.
Checking References and Background
When hiring a data scientist, it’s crucial to thoroughly check their references and background to ensure you’re making an informed decision. This step allows you to gain additional insights into the candidate’s previous work experiences, performance, and character. Here are some key considerations to keep in mind when checking references and conducting background checks:
1. Contacting Previous Employers: Reach out to the candidate’s previous employers or supervisors to get a better understanding of their work ethics, job performance, and level of professionalism. Ask questions about their technical proficiency, ability to meet deadlines, and overall compatibility with the team.
2. Verifying Educational Background: Double-check the candidate’s educational qualifications by contacting the institutions mentioned in their resume. This helps ensure that their academic credentials are accurate and align with the requirements of the position.
3. Assessing Project Contributions: Request specific information about the candidate’s contributions to past projects. This can include the impact they made, the complexity of the tasks they handled, and the overall success of the project. This will give you a better idea of their technical abilities and problem-solving skills.
4. Cultural Fit: Inquire about how well the candidate worked within the company culture. Assess whether they demonstrated strong communication skills, adaptability, and ability to work well in a team environment. Consider how they may fit in with your organization’s values and team dynamics.
5. Background Checks: Consider conducting background checks to ensure the candidate has a clean record and there are no red flags that could pose a risk to your organization. This can involve verifying employment history, criminal records, and any potential conflicts of interest.
6. Online Presence: Take the time to review the candidate’s online presence, such as their professional social media profiles and contributions to the data science community. This can provide further insights into their expertise, thought leadership, and overall reputation.
By thoroughly checking references and conducting background checks, you can gather valuable information that will inform your hiring decision. This process helps validate the information provided by the candidate during the interview process and ensures you make the best possible choice for your organization.
Making the Final Decision
After going through the sourcing, screening, and interview processes, it’s time to make the final decision and select the data scientist who is the best fit for your company. This decision carries significant weight as it will have a direct impact on your organization’s ability to extract actionable insights from data and drive informed decision making.
Here are some key factors to consider when making the final decision:
- Technical Expertise: Evaluate each candidate’s technical skills and knowledge, focusing on their proficiency in programming languages, statistical modeling, machine learning algorithms, and data manipulation techniques. Consider their ability to apply these skills to real-world business problems.
- Problem-Solving Ability: Assess the candidate’s problem-solving capabilities by reviewing their performance in technical interviews and coding challenges. Look for individuals who can think critically, approach problems systematically, and come up with innovative and effective solutions.
- Domain Knowledge: Consider the candidate’s understanding of your industry and the specific challenges you face. Look for candidates who have previous experience working with similar data sets or have expertise in your industry, as this can lead to quicker insights and better decision making.
- Communication and Collaboration Skills: Evaluate the candidate’s ability to communicate complex findings and insights effectively to both technical and non-technical stakeholders. Look for candidates who can effectively collaborate with cross-functional teams and translate business requirements into data-driven solutions.
- Cultural Fit: Assess how well the candidate aligns with your company’s values, mission, and work culture. Consider their willingness to learn, adapt, and contribute to the team dynamic. Look for individuals who show enthusiasm, a growth mindset, and a passion for leveraging data to drive results.
It is essential to involve key stakeholders in the decision-making process, such as the hiring manager, the data science team, and other relevant team leads. Collect feedback from each interview and assessment stage to gain a holistic view of the candidates and their suitability for the role.
Ultimately, the final decision should be based on a combination of technical skills, problem-solving abilities, domain knowledge, communication skills, and cultural fit. It is crucial to prioritize the strengths and qualifications that align with your organization’s specific needs and goals.
Remember, hiring a data scientist is not only about filling a position but also about finding a long-term contributor who can add value to your team and help drive data-driven initiatives forward. Take the time to thoroughly assess each candidate and make an informed decision that will positively impact your company’s growth and success.
Onboarding and Integration of the Data Scientist
Once you have successfully selected a data scientist for your team, the next crucial step is to focus on their onboarding and integration process. This stage is crucial to ensure that the new data scientist feels welcomed, equipped, and ready to contribute effectively to your organization’s goals.
Here are some essential steps to consider when onboarding and integrating a data scientist:
- Orientation and Introduction: Start by providing the data scientist with a comprehensive orientation to the company, its culture, and the specific team they’ll be working with. Introduce them to key stakeholders and colleagues to facilitate networking and relationship building.
- Access to Tools and Infrastructure: Ensure that the data scientist has access to the necessary tools, software, and infrastructure required for their role. This includes granting access to relevant databases, analytics platforms, and collaboration tools.
- Training and Resources: Provide training sessions and resources to help the data scientist get up to speed with the specific technologies, methodologies, and processes used in your organization. This can include access to online courses, internal documentation, and mentorship programs.
- Clarify Expectations: Clearly communicate the goals, objectives, and expectations for the data scientist’s role. Discuss the specific projects they will be working on and the desired outcomes. Set clear timelines and milestones to ensure alignment between the data scientist’s work and the organization’s objectives.
- Ensure Collaboration: Encourage collaboration and cross-functional interaction with other team members. Foster a culture of knowledge sharing and provide opportunities for the data scientist to contribute their expertise and learn from others.
- Performance Feedback and Evaluation: Establish regular feedback sessions to evaluate the data scientist’s performance, identify areas for improvement, and recognize their achievements. This helps to foster a growth mindset and ensures continuous growth and development.
- Support Integration into the Company Culture: Help the data scientist integrate into the company culture by organizing team-building activities, social events, and encouraging participation in corporate initiatives. This will facilitate their sense of belonging and foster strong working relationships.
- Long-term Development: Provide opportunities for the data scientist’s long-term development and growth within the organization. This can include funding for attending conferences, workshops, and providing opportunities for presenting their work externally.
Remember, the onboarding and integration process is not a one-time event but an ongoing effort. Regular communication, support, and feedback will ensure a smooth transition and help the data scientist become a valuable asset to your organization.
Conclusion
Hiring a data scientist can be a game-changer for your business. These highly skilled professionals bring a unique set of expertise that can unlock valuable insights and drive data-driven decision-making. By following the steps outlined in this article, you can ensure a successful hiring process.
First and foremost, clearly define your specific needs and goals to determine the skills and qualifications required in a data scientist. Conduct a thorough candidate search through various channels such as online job boards, social media, and professional networks.
During the interview process, assess the technical skills of the candidates by posing relevant questions and giving them real-world problem-solving scenarios. It is also crucial to evaluate their analytical thinking abilities, communication skills, and cultural fit within your organization.
After narrowing down the candidates, make sure to check their references and conduct background checks to ensure their qualifications and credibility. Lastly, consider offering a competitive compensation package to attract top talent.
Remember, hiring a data scientist is an investment that can yield significant returns for your business. By taking the time to find the right candidate, you can leverage their expertise to gain valuable insights, improve processes, and drive innovation in today’s data-driven world.
FAQs
1. Why should I hire a data scientist?
Hiring a data scientist can greatly benefit your business in many ways. Data scientists have the expertise to analyze large volumes of data, uncover meaningful insights, and make data-driven decisions. With their skills in machine learning and statistical analysis, they can help your company gain a competitive edge, optimize operations, and identify new growth opportunities.
2. How do I know if I need a data scientist?
If your business deals with a significant amount of data or if you are looking to leverage data analytics to improve your operations, it’s likely that you would benefit from hiring a data scientist. Some signs that indicate a need for a data scientist include struggling to make sense of large datasets, lacking the necessary analytical skills in-house, or wanting to implement machine learning algorithms for predictive analytics.
3. What skills should I look for when hiring a data scientist?
When hiring a data scientist, look for candidates with strong skills in statistics, programming (such as Python or R), and data visualization. They should also have a solid background in machine learning algorithms and experience in working with big data. Additionally, good communication and problem-solving skills are essential for a data scientist to effectively convey insights and solve complex analytical problems.
4. How can I assess the proficiency of a data scientist?
To assess the proficiency of a data scientist, you can consider various factors. Look for candidates with relevant educational qualifications, such as a degree in data science or a related field. You can also evaluate their past projects, research papers, or contributions to the data science community. Additionally, conducting technical interviews or giving them a sample data problem to solve can help you gauge their technical skills and problem-solving abilities.
5. Should I hire a full-time data scientist or outsource the work?
Deciding whether to hire a full-time data scientist or outsource the work depends on your specific circumstances and requirements. If your data analysis needs are ongoing and complex, hiring a full-time data scientist can be a wise investment, as they can dedicate their time and expertise solely to your business. On the other hand, if you have short-term projects or if you don’t have a consistent need for data analysis, outsourcing the work to a specialized data consultancy or freelancer can be a cost-effective solution.