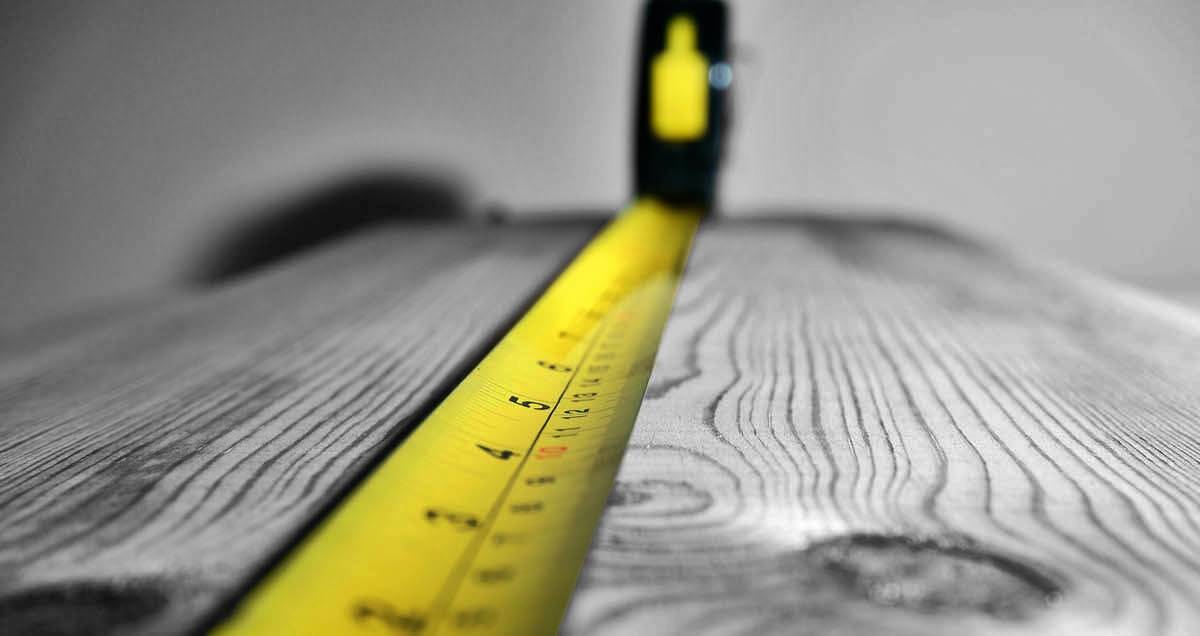
When it comes to managing data, ensuring its integrity is of utmost importance. Data integrity refers to the accuracy, consistency, and reliability of data stored and processed within a system or database. It plays a crucial role in various industries, such as finance, healthcare, and e-commerce, where the integrity of data directly impacts decision-making processes and business operations.
Measuring data integrity involves assessing the quality and completeness of data, as well as identifying any errors, inconsistencies, or discrepancies that may exist. By regularly measuring data integrity, organizations can proactively detect and resolve issues, ensuring that their data remains reliable and trustworthy.
In this article, we will explore various methods and techniques to measure data integrity effectively. We will delve into the importance of data validation, data cleansing, and data auditing in maintaining data accuracy. So, if you’re ready to take control of your data’s integrity, let’s dive in!
Inside This Article
- Overview of Data Integrity
- Importance of Data Integrity
- Common Methods to Measure Data Integrity
- Factors Affecting Data Integrity Measurement
- Conclusion
- FAQs
Overview of Data Integrity
Data integrity refers to the accuracy, consistency, and reliability of data throughout its lifecycle. It ensures that data remains intact and unaltered, maintaining its integrity and trustworthiness. In today’s data-driven world, where organizations rely heavily on data for decision-making and operations, maintaining data integrity is of paramount importance.
Data integrity involves various aspects, including data creation, storage, transmission, and retrieval. It ensures that data remains complete, consistent, and reliable across different systems, applications, and processes. By maintaining data integrity, organizations can confidently rely on their data to drive insights, make informed decisions, and achieve their business objectives.
Data integrity is crucial for several reasons. It helps prevent data corruption, unauthorized modifications, and data loss. Without data integrity, organizations risk making decisions based on inaccurate or incomplete information, which can have detrimental effects on their operations, reputation, and customer satisfaction.
Measuring data integrity allows organizations to assess the quality and reliability of their data. It provides insights into the accuracy and consistency of the data, helping identify any potential issues or vulnerabilities. By understanding the level of data integrity, organizations can take appropriate measures to strengthen data management processes, ensure data accuracy, and mitigate risks.
There are various methods to measure data integrity, which we will explore in the next section. These methods involve assessing data quality, data validation techniques, and statistical analysis to detect anomalies or inconsistencies in the data. By applying these measurement techniques, organizations can gain a deeper understanding of their data integrity and take necessary actions to improve it.
Factors such as data governance, data security measures, data storage systems, and data validation processes significantly impact data integrity measurement. Organizations need to establish robust data management practices, implement secure storage solutions, and enforce data validation protocols to ensure data integrity throughout its lifecycle.
To summarize, data integrity is a critical aspect of data management. It ensures that data remains accurate, consistent, and reliable. Measuring data integrity helps organizations assess the quality of their data and identify areas for improvement. By prioritizing data integrity, organizations can make more informed decisions, improve operational efficiency, and gain a competitive advantage in today’s data-centric landscape.
Importance of Data Integrity
Data integrity is a crucial aspect of any organization’s data management strategy. It refers to the accuracy, consistency, and reliability of data throughout its lifecycle. Ensuring data integrity is essential because it provides numerous benefits and safeguards against potential risks. Let’s explore why data integrity is of paramount importance.
1. Trustworthy Decision Making: Data plays a vital role in shaping strategic decisions. With data integrity, organizations can rely on the accuracy and consistency of their data to make well-informed decisions. This enables them to assess market trends, identify potential risks, and seize opportunities for growth.
2. Regulatory Compliance: Various industries have strict regulations regarding data privacy and protection. Adhering to these regulations is crucial to maintaining legal compliance. Data integrity ensures that sensitive information remains secure and accurate, reducing the risk of penalty fines and reputational damage.
3. Enhanced Customer Satisfaction: Data integrity is directly related to customer satisfaction. When customers provide information to an organization, they expect it to be safeguarded and used accurately. With data integrity, organizations can maintain trust and deliver personalized experiences, resulting in improved customer satisfaction and long-term loyalty.
4. Reduced Operational Risks: Inaccurate or inconsistent data can lead to operational inefficiencies and errors. Data integrity mitigates these risks by ensuring that data is reliable and consistent. Organizations can streamline their operations, improve productivity, and reduce the likelihood of costly mistakes.
5. Data-driven Insights: Reliable data is the foundation for generating meaningful insights. When data integrity is maintained, organizations can leverage data analytics to gain actionable insights and a competitive edge. This allows them to identify trends, optimize processes, and make data-driven decisions that drive business growth.
6. Protection against Data Breaches: Data breaches present significant risks to organizations, including financial losses, reputational damage, and legal implications. By prioritizing data integrity, organizations can establish robust security measures, such as encryption and access controls, to protect data from unauthorized access and minimize the risk of data breaches.
Common Methods to Measure Data Integrity
Measuring data integrity is crucial for businesses to ensure the accuracy, reliability, and consistency of their data. There are several common methods that organizations can employ to assess the integrity of their data. Let’s take a closer look at these methods:
1. Data Verification: This method involves comparing the data in the system with the original source or a trusted reference dataset. By performing checks and validations, organizations can identify any discrepancies or errors in the data.
2. Data Sampling: In this method, a representative sample of the data is selected and analyzed. By examining a subset of the data, organizations can gain insights into the overall quality and integrity of the entire dataset. Sampling can help identify anomalies, outliers, or missing values within the data.
3. Data Reconciliation: This method involves cross-checking data between different systems or sources to ensure consistency and accuracy. By comparing data from multiple sources, organizations can identify and resolve any discrepancies, duplicates, or inconsistencies in the data.
4. Data Auditing: Data auditing involves systematically reviewing and examining data to ensure compliance with predefined standards and policies. This method helps organizations identify any unauthorized changes, modifications, or access to the data, ensuring that the data remains secure and intact.
5. Data Profiling: Data profiling involves analyzing and assessing the structure, content, and quality of the data. By examining various attributes such as completeness, uniqueness, and consistency, organizations can gain insights into the integrity of the data and identify any potential data issues or anomalies.
6. Data Monitoring: This method involves continuously monitoring the data to detect any changes or anomalies. By implementing data monitoring tools and techniques, organizations can quickly identify any unauthorized modifications, data loss, or data corruption, ensuring the integrity of the data.
7. Data Integrity Frameworks: Implementing a data integrity framework provides a structured approach to measure and ensure the integrity of the data. These frameworks typically include a set of guidelines, best practices, and metrics that organizations can use to assess and maintain the integrity of their data.
It is essential for organizations to adopt these common methods to measure data integrity regularly. By implementing these methods, businesses can identify and resolve data integrity issues, ensuring the accuracy and reliability of their data, and improving overall business operations.
Factors Affecting Data Integrity Measurement
When it comes to measuring data integrity, there are several factors that can have an impact on the accuracy and reliability of the results. These factors should be taken into consideration to ensure that the data integrity measurement is comprehensive and valid. Below are some of the key factors that can affect the measurement of data integrity:
Data Collection Methodology: The method used to collect data can greatly impact the measurement of data integrity. Whether data is collected manually or automatically, the process should be well-defined and consistent to ensure accurate results.
Data Quality: The quality of the data being measured is a critical factor. If the data contains errors, duplicates, or inconsistencies, it can lead to misleading results and compromise the integrity of the measurement. Therefore, it is important to have mechanisms in place to monitor and improve data quality.
Data Storage and Accessibility: The way data is stored and accessed can affect its integrity. If data is stored in a secure and well-maintained database, with proper access controls in place, the chances of data corruption or tampering are minimized. On the other hand, if data is stored in an unsecured or poorly organized system, it becomes more vulnerable to integrity issues.
Data Validation and Verification: Validating and verifying the data before and after measurement is crucial for ensuring data integrity. This process involves cross-checking the data against known standards, performing consistency checks, and verifying the accuracy of the measurements. By implementing robust data validation and verification procedures, the integrity of the measurement can be enhanced.
Data Governance and Documentation: Having a clear data governance framework and documentation is essential for maintaining data integrity. This includes establishing data standards, guidelines, and practices, as well as documenting the entire data lifecycle. Without proper data governance and documentation, it becomes difficult to track and assess the integrity of the data measurement.
System Security and Compliance: The security measures implemented within the system play a crucial role in data integrity measurement. The system should have adequate security controls to prevent unauthorized access, data breaches, or tampering. Additionally, the system should be compliant with relevant regulations and standards to ensure the integrity of the data measurement.
Human Error and Bias: Human error and bias can significantly impact data integrity measurement. It is important to train and educate the individuals responsible for data collection and measurement to minimize errors and biases. Additionally, implementing quality control measures, such as double-checking data entries, can help mitigate the risks associated with human error and bias.
By considering these factors and implementing appropriate measures, organizations can ensure accurate and reliable data integrity measurement. This, in turn, helps in making informed decisions and maintaining the trustworthiness of the data.
Conclusion
Measuring data integrity is crucial for any organization that wants to maintain accuracy, reliability, and trust in its data. By implementing proper data integrity measures, businesses can ensure that their data is complete, consistent, and free from errors or corruption.
Throughout this article, we explored different methods and techniques for measuring data integrity, including checksums and validation methods. We also discussed the importance of data backups and performed a step-by-step guide on how to measure data integrity using checksums.
Remember, data integrity is not a one-time task but an ongoing process. Regular audits, monitoring, and maintenance are necessary to detect and rectify any issues that may arise. By prioritizing data integrity, organizations can boost their operational efficiency, make informed decisions, and maintain a competitive edge in today’s data-driven world.
So, take the necessary steps to measure and ensure data integrity in your organization, and safeguard the reliability and credibility of your data.
FAQs
1. What is data integrity?
Data integrity refers to the accuracy, completeness, and reliability of data throughout its lifecycle. It ensures that data remains consistent and trustworthy, free from errors, duplications, or unauthorized modifications.
2. Why is data integrity important?
Data integrity is crucial as it ensures the reliability of data for decision-making, business operations, and compliance purposes. Maintaining data integrity helps organizations build trust with customers and stakeholders, avoid costly errors, and ensure the effectiveness of data-driven initiatives.
3. How can data integrity be measured?
Data integrity can be measured through various techniques, such as:
- Data quality assessments
- Data profiling and analysis
- Data validation and verification
- Record-level checks and audits
- Comparison with external sources or benchmarks
These methods help identify and quantify data anomalies, inconsistencies, and errors, providing insights into the overall data integrity.
4. What are the potential causes of data integrity issues?
Several factors can contribute to data integrity issues, including:
- Human error during data entry or manipulation
- Software or hardware failures
- Data migration or integration challenges
- Security breaches or unauthorized access
- Data corruption or loss
Recognizing these potential causes can help organizations establish preventive measures and proactive data management strategies.
5. How can organizations ensure data integrity?
To ensure data integrity, organizations should implement the following best practices:
- Establish data governance policies and procedures
- Implement data validation rules and quality checks
- Regularly monitor and audit data for inconsistencies
- Invest in robust data backup and recovery systems
- Train employees on data handling and security protocols
- Adopt encryption and access control measures
By adopting these practices, organizations can maintain data integrity and minimize the risk of data-related issues.