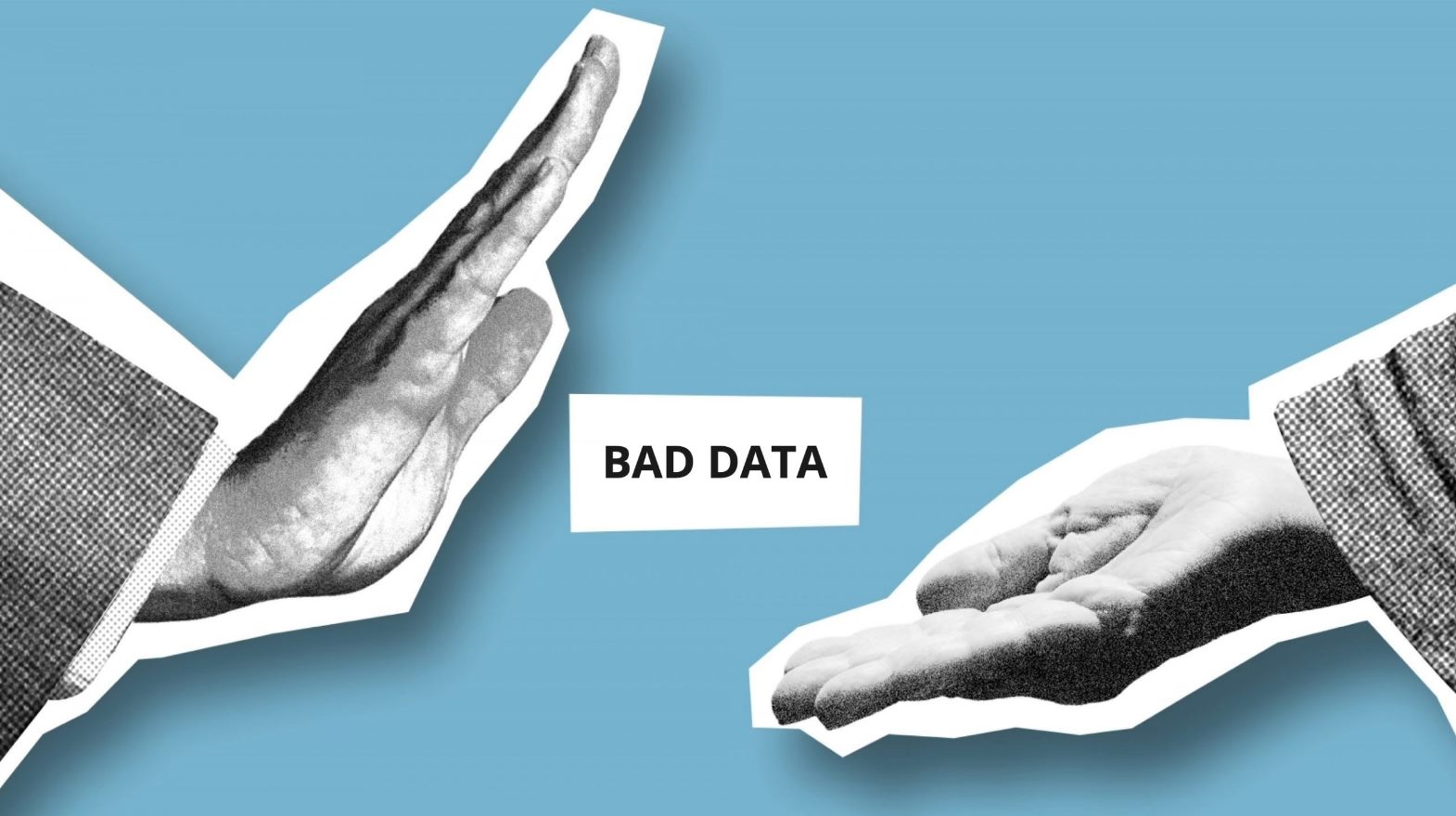
Data quality is a crucial metric that businesses need to monitor and address regularly. It refers to the accuracy, completeness, consistency, and reliability of data. In today’s data-driven world, where decisions are based on the insights derived from data, ensuring high-quality data is paramount.
But how can you measure data quality? In this article, we’ll dive into the various methods and metrics used to assess data quality. Whether you’re a data analyst, a data scientist, or a business owner, understanding how to measure data quality will enable you to make informed decisions, identify data issues, and improve the overall reliability of your data.
Inside This Article
- What is Data Quality?
- Importance of Measuring Data Quality
- Common Methods for Measuring Data Quality
- Key Metrics Used to Evaluate Data Quality
- Conclusion
- FAQs
What is Data Quality?
Data quality refers to the overall accuracy, completeness, consistency, and reliability of data. In simple terms, it is the degree to which data can be trusted and relied upon to make informed business decisions. Data quality encompasses various aspects, including the completeness of data, the absence of errors or inconsistencies, and the timeliness of data.
Accurate data is free from errors, ensuring that the information reflects the reality it is intended to represent. Completeness refers to the presence of all required data elements, leaving no gaps or missing values. Consistency pertains to the uniformity and coherence of data across different sources or databases. Reliable data is dependable and can be consistently used to support decision-making.
Quality data is crucial for organizations to effectively analyze and interpret information, identify trends, and make data-driven decisions. It ensures that organizations have a solid foundation to determine strategies, measure performance, and improve operational efficiency. Without reliable data, organizations run the risk of making flawed decisions, ineffective plans, and inefficient processes.
Measuring data quality is a critical step in ensuring the reliability and usefulness of data. By assessing data quality, organizations can identify areas for improvement, prioritize data management efforts, and establish data governance processes. With accurate and high-quality data, businesses can gain a competitive edge, enhance customer satisfaction, and drive better outcomes.
Importance of Measuring Data Quality
Measuring data quality is crucial for businesses in the digital age. In today’s data-driven world, companies rely heavily on accurate and reliable data to make informed decisions, drive business strategies, and gain a competitive edge. Without high-quality data, organizations risk making flawed decisions that can have far-reaching consequences.
One of the main reasons why measuring data quality is important is because it helps establish trust and credibility. When data is accurate, complete, and consistent, it instills confidence among stakeholders, including customers, suppliers, and business partners. This, in turn, strengthens relationships and enhances the reputation of the company.
Another key reason is that measuring data quality enables organizations to identify and rectify any issues or errors in the data. By monitoring data quality regularly, companies can spot inaccuracies, duplication, missing values, and other issues that can impact decision-making. This proactive approach ensures that data remains reliable and actionable.
Measuring data quality also plays a vital role in improving operational efficiency. When data is of high quality, processes can run smoothly, and there is less room for errors and delays. This leads to better productivity, cost savings, and improved customer satisfaction. On the other hand, poor data quality can lead to wastage of resources, inefficient workflows, and tarnished customer experiences.
Furthermore, measuring data quality helps organizations meet regulatory and compliance requirements. In many industries, such as finance, healthcare, and government, strict regulations govern the handling and protection of data. By ensuring data quality, companies can demonstrate compliance with these regulations and mitigate the risk of penalties, lawsuits, and reputational damage.
Lastly, measuring data quality enables businesses to unlock the full potential of their data. Quality data serves as a foundation for data analysis, predictive modeling, machine learning, and other advanced analytics techniques. By measuring data quality, organizations can optimize these processes, gain deeper insights, and make data-driven decisions that bring meaningful results.
Common Methods for Measuring Data Quality
Measuring data quality is crucial for any organization that relies on accurate and reliable data for decision-making. Without proper measurement and assessment, data quality issues can go unnoticed, leading to faulty analysis and incorrect business decisions. In this section, we will explore some common methods used to measure data quality.
1. Data Profiling: Data profiling involves analyzing the structure, content, and quality of the data. It helps to identify inconsistencies, duplicates, missing values, and other data quality issues. By profiling the data, organizations can gain insights into the overall quality of their datasets.
2. Data Cleansing: Data cleansing is the process of correcting or removing errors, inconsistencies, and inaccuracies from the dataset. It involves techniques such as deduplication, standardization, and validation to ensure data accuracy. By cleansing the data, organizations can enhance its quality and reliability.
3. Data Monitoring: Data monitoring involves continuously monitoring the quality of data in real-time. This method uses automated tools and processes to track data quality metrics, identify anomalies, and generate alerts for potential issues. By monitoring data quality, organizations can quickly detect and resolve any data-related problems.
4. Data Quality Scorecards: Data quality scorecards provide a visual representation of the overall quality of the data. These scorecards use key performance indicators (KPIs) and metrics to measure data quality against predefined benchmarks. By using scorecards, organizations can easily evaluate and track data quality over time.
5. User Feedback: User feedback is an important method for measuring data quality. By actively seeking feedback from users who interact with the data, organizations can identify any issues or discrepancies. This feedback helps to validate the accuracy and relevance of the data, ensuring its quality.
6. Data Audits: Data audits involve a comprehensive examination of the data to assess its quality and compliance with regulations and standards. Audits can be conducted internally or by third-party experts to ensure data integrity, security, and privacy. By regularly auditing the data, organizations can maintain high-quality datasets.
7. Data Validation: Data validation is the process of ensuring that the data meets specific criteria, including accuracy, consistency, and completeness. Validation techniques can include data profiling, rule-based checks, and statistical analysis. By validating the data, organizations can have confidence in its quality and reliability.
These are some common methods used to measure data quality. Every organization should adopt a combination of these methods depending on their specific data requirements and business goals. By implementing robust data quality measurement practices, organizations can maximize the value of their data and make informed decisions.
Key Metrics Used to Evaluate Data Quality
Data quality plays a crucial role in the success of any organization’s data-driven initiatives. To ensure the accuracy, completeness, and integrity of data, it’s important to measure its quality using various metrics. Here are some key metrics commonly used to evaluate data quality:
1. Accuracy: This metric assesses the correctness of data. It measures the extent to which data values align with the actual values in the source or reference systems. Accuracy is often evaluated by comparing data against trusted sources or conducting manual data validation.
2. Completeness: This metric evaluates the extent to which data is complete. It measures the presence of all required data elements and ensures that there are no missing values or fields in the dataset. Completeness can be assessed by comparing the expected data elements with the actual ones.
3. Consistency: Consistency measures the uniformity and coherence of data across different sources or systems. It ensures that data values and formats are standardized and aligned throughout the dataset. Consistency can be evaluated by comparing data across various systems or conducting data mapping exercises.
4. Timeliness: Timeliness assesses the freshness and currency of data. It measures how quickly data is captured, processed, and made available for analysis. Timeliness is crucial in decision-making processes where up-to-date information is vital. This metric can be evaluated by tracking data capture and update timestamps.
5. Validity: Validity measures the conformity of data to predefined rules and constraints. It ensures that data adheres to the defined data formats, data types, and business rules. Validity can be evaluated by applying validation rules and checks against the dataset to identify any non-conformities.
6. Integrity: Integrity evaluates the accuracy, consistency, and reliability of relationships or associations between data elements. It ensures the logical coherence and interdependencies between different data points. Integrity can be assessed by examining data relationships, conducting referential integrity checks, and identifying any inconsistencies.
7. Duplication: Duplication measures the presence of redundant or duplicate data records in the dataset. It helps identify and eliminate duplicate entries, reducing data redundancy and improving data quality. Duplication can be evaluated by conducting matching and deduplication exercises to identify similar or identical records.
8. Precision: Precision measures the level of detail and specificity in the recorded data values. It ensures that data is accurately represented without any rounding or truncation errors. Precision can be evaluated by examining the decimal places or significant figures in numerical data.
9. Relevance: Relevance measures the usefulness and applicability of data in meeting the intended objectives and requirements. It ensures that data is relevant to the specific business context or analytical needs. Relevance can be evaluated based on predefined criteria and user feedback.
By measuring these key metrics, organizations can gain insights into the quality of their data and identify areas where improvements are needed. This enables them to make informed decisions, improve operational efficiency, and drive successful data-driven initiatives.
Conclusion
Measuring data quality is a critical aspect of any successful data-driven strategy. By ensuring the accuracy, completeness, consistency, and timeliness of your data, you can make informed business decisions and drive better outcomes. Whether you are analyzing customer data, market trends, or operational metrics, a strong data quality measurement process is essential.
Start by defining clear criteria for data quality and aligning them with your business objectives. Implement data cleansing and validation techniques to identify and rectify errors and inconsistencies. Regularly monitor and evaluate the quality of your data using appropriate metrics and indicators. Continuously strive to improve data quality by integrating data governance and quality control practices into your organization’s culture.
Remember, data quality is not a one-time effort, but an ongoing process that requires dedication and commitment. By investing in measuring and improving data quality, you can unlock the full potential of your data and gain a competitive advantage in today’s data-driven world.
FAQs
Q: What is data quality?
A: Data quality refers to the overall accuracy, completeness, consistency, and relevance of data. It is crucial for businesses to have high-quality data to make informed decisions and achieve their objectives.
Q: Why is data quality important?
A: Data quality is important because it directly impacts the reliability of business insights and analytics. Poor data quality can lead to inaccurate analysis, flawed decision-making, and wasted resources. On the other hand, high-quality data ensures accurate insights and helps organizations gain a competitive edge.
Q: How can I measure data quality?
A: Measuring data quality involves evaluating various dimensions, such as accuracy, completeness, validity, consistency, and timeliness. This can be done through data profiling, data cleansing, data monitoring, data quality assessments, and data quality scorecards.
Q: What are some common metrics used to measure data quality?
A: Some common metrics used to measure data quality include:
- Accuracy: Measures how close the data values are to their true or expected values.
- Completeness: Evaluates the extent to which data is missing or incomplete.
- Consistency: Assesses the coherence and conformity of data across different sources or within a dataset.
- Timeliness: Determines how up-to-date the data is and whether it is aligned with business requirements.
Q: What are some challenges in measuring data quality?
A: Measuring data quality can be challenging due to various factors, including:
- Data complexity: Data can be complex, heterogeneous, and stored in different formats, making it difficult to assess quality consistently.
- Missing metadata: Lack of proper documentation and understanding about data sources can hinder accurate measurement of data quality.
- Changing data requirements: Business needs and data requirements evolve over time, making it necessary to continuously redefine measurement criteria.
- Incomplete data integration: Data may come from multiple sources, making it necessary to ensure proper integration and quality assessment across those sources.