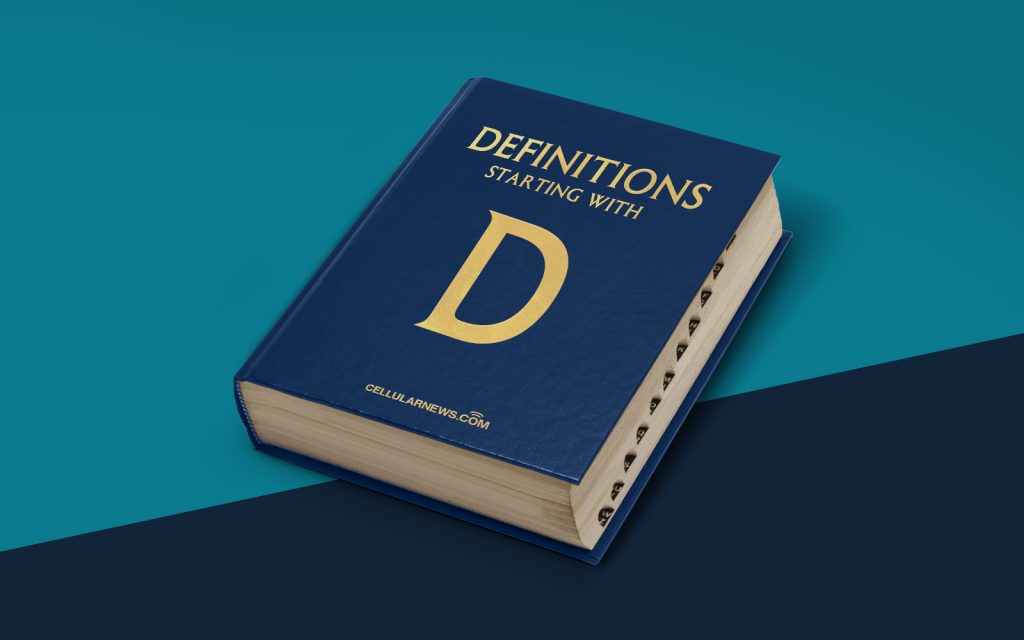
What is Deep Analytics?
Welcome to our “DEFINITIONS” category! In this post, we’ll delve into the meaning and significance of Deep Analytics. Whether you’re new to the term or looking to expand your knowledge, we’ve got you covered.
Deep Analytics refers to the profound exploration and examination of data to uncover valuable insights and patterns. It involves the use of sophisticated algorithms, machine learning techniques, and statistical models to gain a comprehensive understanding of complex datasets. By extracting this meaningful information, organizations can make informed decisions, optimize processes, and drive growth.
Key Takeaways:
- Deep Analytics involves exploring and analyzing data to uncover valuable insights.
- It utilizes advanced algorithms and statistical models to gain a deep understanding of complex datasets.
Now that we have a basic understanding, let’s dive deeper into the essential aspects of Deep Analytics:
1. Data Collection and Integration
Deep Analytics starts with the collection and integration of relevant data from diverse sources. These sources may include structured data from databases, unstructured data from social media, or even the Internet of Things (IoT) devices. Gathering and organizing this data is crucial for carrying out effective analysis.
2. Advanced Analytics Techniques
In Deep Analytics, traditional analytical techniques are enhanced with advanced algorithms, machine learning, and statistical models. These techniques allow for the extraction of deep, meaningful insights from the data. By applying machine learning algorithms, patterns and trends can be identified, correlations can be established, and predictions can be made.
Furthermore, deep learning techniques such as neural networks can be employed to uncover complex relationships within the data. This enables organizations to reveal insights that were previously inaccessible.
3. Visualization and Communication
Deep Analytics is not just about crunching numbers and generating reports; it’s also about effectively communicating the findings to key stakeholders. Data visualization plays a vital role in this process, as it transforms complex data into easy-to-understand visuals such as charts, graphs, and dashboards.
Visualizations help decision-makers grasp the insights at a glance, facilitating faster and more accurate decision-making. Effective communication of the analytics results drives actions and fosters a data-driven culture within organizations.
4. Data Privacy and Security
As organizations deal with ever-increasing volumes of data, ensuring data privacy and security is paramount. Deep Analytics involves handling sensitive information, and thus, robust measures must be in place to protect data from unauthorized access, breaches, or misuse.
Organizations complying with data privacy regulations, such as the General Data Protection Regulation (GDPR), must ensure that deep analysis is carried out while safeguarding individuals’ privacy rights and adhering to legal requirements.
5. Real-Time Analytics
In today’s fast-paced environment, real-time analytics have become crucial for organizations seeking to gain a competitive edge. Deep Analytics enables organizations to process and analyze data in real-time, allowing for immediate insights and actions.
Real-time analytics can be particularly beneficial for industries such as e-commerce, finance, and logistics, where quick decision-making can significantly impact business outcomes. By leveraging the power of Deep Analytics, organizations can respond swiftly to changing market dynamics and make data-driven decisions in the moment.
Deep Analytics is revolutionizing the way organizations leverage data. By going beyond surface-level analysis, organizations can unlock hidden insights, make informed decisions, and stay ahead of the competition. Embracing Deep Analytics can empower businesses to unleash the true value of their data and drive sustainable growth.