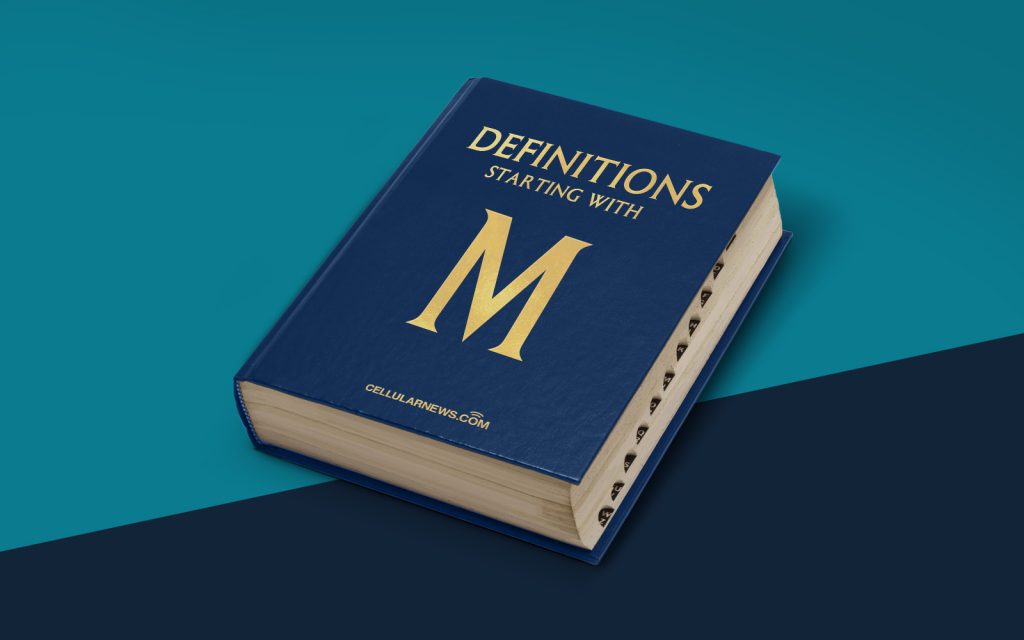
What is Multimodal AI?
Have you ever wondered what Multimodal AI is? Well, you’ve come to the right place! In this article, we will explore the definition, uses, challenges, and applications of Multimodal AI. So, let’s dive in!
Key Takeaways:
- Multimodal AI refers to the field of artificial intelligence that focuses on understanding and processing information from multiple modalities, such as text, images, speech, and videos.
- It combines various machine learning techniques to analyze and interpret data from different sources, enabling systems to have a more comprehensive understanding of the world.
Multimodal AI is an interdisciplinary field that combines computer vision, natural language processing, speech recognition, and other AI techniques to build intelligent systems that can perceive and understand information from multiple sources. It aims to bridge the gap between different modes of communication and provide more human-like interactions between machines and humans.
Uses of Multimodal AI:
Multimodal AI has a wide range of applications across various industries. Here are some of its key uses:
- Image and Scene Understanding: Multimodal AI can analyze images and scenes to understand their content and context. This can be utilized in applications like object recognition, scene understanding, and visual search.
- Speech and Text Understanding: Multimodal AI can process both speech and text data to extract meanings and sentiments, enabling applications such as voice assistants, chatbots, and sentiment analysis.
- Human-Computer Interaction: Multimodal AI can enhance the interaction between humans and computers by enabling systems to understand gestures, facial expressions, and other non-verbal cues.
- Translation and Transcription: Multimodal AI can facilitate translation and transcription services by combining speech recognition and language processing techniques.
Challenges in Multimodal AI:
While Multimodal AI offers exciting possibilities, it also comes with its own set of challenges. Some of the key challenges include:
- Data Integration: Integrating and processing data from multiple modalities can be complex and resource-intensive.
- Alignment and Fusion: Aligning and fusing information from different modalities can be a challenge due to differences in data representation and context.
- Scalability: Scaling multimodal AI systems to handle large volumes of data in real-time can be a computational challenge.
- Evaluation Metrics: Defining evaluation metrics to assess the performance of multimodal AI systems is still an ongoing research topic.
In conclusion, Multimodal AI is an exciting field that combines different AI techniques to process and understand information from multiple modalities. It has a wide range of applications across various industries and holds great potential for enhancing human-computer interactions. However, there are also challenges to address, such as data integration, alignment, scalability, and evaluation metrics. As technology advances, we can expect Multimodal AI to continue to evolve and contribute to the development of more intelligent and interactive systems.