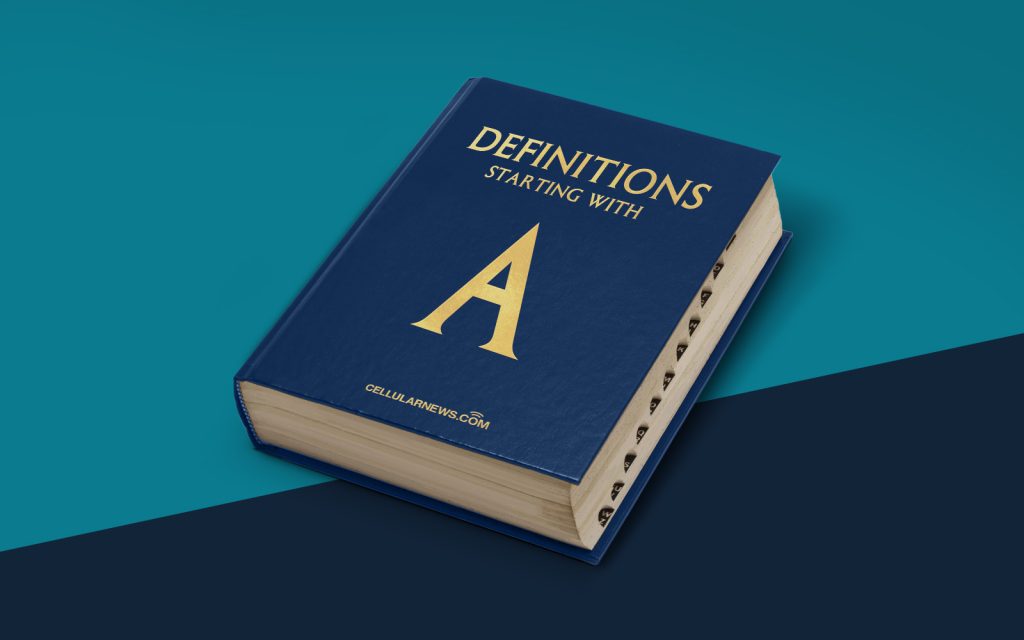
Unveiling the Curious Phenomenon of AI Hallucination
Have you ever wondered how artificial intelligence (AI) can sometimes produce bizarre, nonsensical outputs? This intriguing occurrence is known as AI hallucination. In this blog post, we will delve into the world of AI hallucination, exploring its definition, providing examples, discussing its causes, and offering tips on how to spot it. So, let’s embark on this fascinating journey!
Key Takeaways:
- AI hallucination refers to the phenomenon where artificial intelligence systems generate strange and unexpected outputs.
- It can occur in various AI applications such as language processing, image recognition, and even chatbots.
What is AI Hallucination?
AI hallucination, also known as AI-generated hallucination or AI bias, is a puzzling occurrence where an AI system produces seemingly illogical or nonsensical outputs. These outputs may deviate significantly from what we would expect given the input or context. While AI is designed to learn patterns and generate accurate results, hallucination can occur as a side effect of the learning process.
AI hallucination is not a deliberate act of the AI, but rather a reflection of the limitations and biases within the training data or algorithms. Just as humans can experience illusions or see patterns that don’t exist in reality, AI can “hallucinate” based on flawed or incomplete information.
Examples of AI Hallucination
The realm of AI hallucination is vast, and there are numerous examples that highlight its peculiarities. Here are a few notable instances:
- An AI-powered language processing system consistently generates nonsensical sentences that have no coherent meaning, despite having been trained on vast amounts of text data.
- An autonomous vehicle’s object recognition system misidentifies everyday objects, labeling a stop sign as a speed limit sign or a pedestrian as a lamppost.
- A chatbot, designed to assist customers, responds to queries with irrelevant or nonsensical answers, failing to grasp the context or intention behind the questions.
These examples demonstrate the potential for AI hallucination across various domains and highlight the challenges AI developers face in ensuring accurate and coherent outputs.
Causes of AI Hallucination
AI hallucination can be attributed to several causes, including:
- Training on biased or incomplete data: If an AI system learns from data that contains biases or lacks diversity, it may generate outputs that mirror those biases or overlook certain details.
- Overfitting: When an AI system becomes too focused on the training data and fails to generalize well to new inputs, it can lead to hallucination.
- Flawed algorithms or models: Errors or limitations in the design of AI algorithms or models can contribute to hallucination. These flaws can be related to how the AI processes and interprets information.
How to Spot AI Hallucination?
Recognizing AI hallucination can be a challenging task, especially since it is often detected only after the system has been deployed. However, here are some tips to help you spot potential cases of AI hallucination:
- Compare outputs against ground truth: Assess the outputs of an AI system against known ground truth or human-created benchmarks to identify any inconsistencies or nonsensical results.
- Conduct extensive testing: Thoroughly test the AI system during the development phase to identify any warning signs of unusual or unexpected outputs.
- Engage human oversight: Implement human review or monitoring to catch and correct any instances of hallucination that the AI system may produce.
By employing these strategies, developers can gain a deeper understanding of AI hallucination and develop effective techniques to mitigate its impact.
Conclusion
As AI becomes increasingly prevalent in our lives, it is crucial to understand and address the nuances of AI hallucination. By recognizing its causes and learning to spot its occurrence, we can take steps towards minimizing its impact and ensuring that AI systems produce accurate and reliable outputs. While AI hallucination may be intriguing, it is essential to develop robust safeguards and oversight mechanisms to harness AI’s true potential for the benefit of humankind.