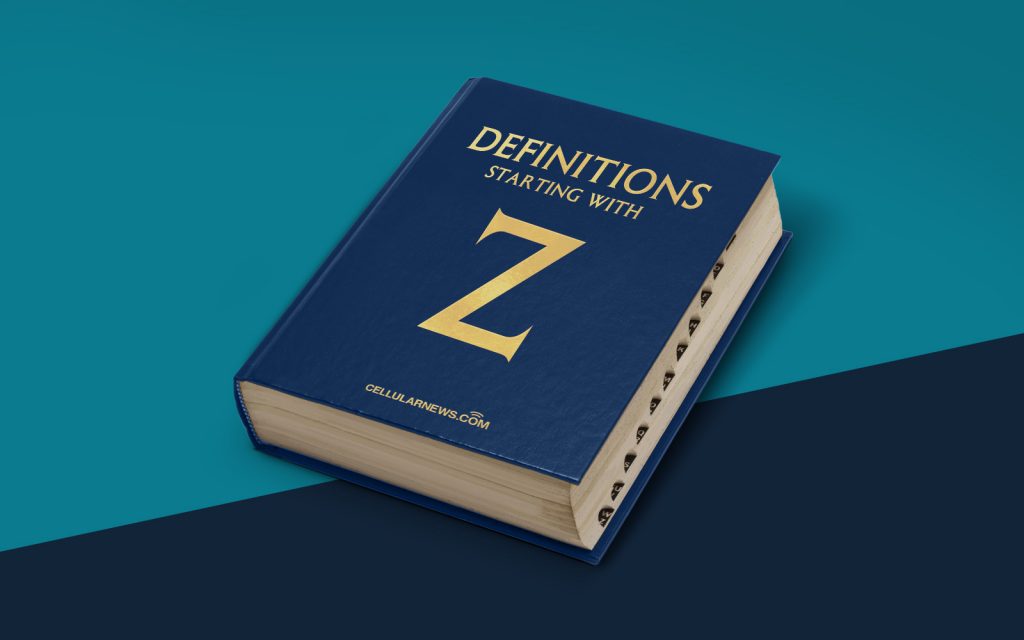
Understanding Zero-Shot, One-Shot, and Few-Shot Learning
Welcome to our “Definitions” blog series! Today, we are diving into the intriguing world of Zero-Shot, One-Shot, and Few-Shot Learning. Whether you’re a tech enthusiast, a data scientist, or just curious about cutting-edge AI algorithms, you’ve come to the right place. In this article, we’ll demystify these three fascinating concepts and explore how they are revolutionizing the field of machine learning.
Key Takeaways:
- Zero-Shot Learning enables machines to learn and generalize concepts without specific training examples.
- One-Shot Learning refers to the ability of machines to recognize and classify objects after seeing just a single example.
Zero-Shot Learning: Expanding Machine Learning Boundaries
Zero-Shot Learning is an exciting branch of machine learning that pushes the boundaries in training models without explicit supervision. Traditionally, machine learning algorithms require a vast amount of labeled data to perform accurate predictions. However, zero-shot learning takes a different approach. It allows machines to understand and generate predictions for classes or concepts they have never encountered before.
The key concept behind zero-shot learning is the utilization of semantic information or attributes associated with the classes. By leveraging these semantic representations, machines can infer how each class is related to others, leading to the ability to make predictions on unseen data. This innovative approach augments the power of machine learning, as it enables systems to tackle new and unforeseen scenarios with minimal human intervention.
One-Shot Learning: Recognizing the Unseen
One-Shot Learning takes the idea of generalizing from limited data and brings it to the extreme. It involves the training of algorithms to recognize and classify objects based on just one piece of information or a single example. While it may seem mind-boggling, one-shot learning exemplifies the remarkable pattern recognition capabilities of artificial intelligence.
By employing techniques such as Siamese neural networks or metric learning, one-shot learning enables machines to learn meaningful representations from a single instance. This ability mimics the human cognitive process, where we can effortlessly identify new objects or faces despite having seen them only once.
Few-Shot Learning: Bridging the Gap
Few-Shot Learning acts as the bridge between zero-shot and one-shot learning approaches. It acknowledges that in real-world scenarios, complete absence of training examples for a new class is rare. Rather, a limited number of instances may be available. Few-shot learning caters to this scenario and aims to train models capable of recognizing and classifying concepts with only a small amount of data.
To achieve this, few-shot learning relies on techniques like transfer learning and meta-learning. These methods enable models to leverage knowledge acquired from related classes or tasks during training, allowing them to quickly adapt and generalize to unseen concepts. Few-shot learning has a wide range of applications, from image recognition to natural language understanding, where data scarcity is often a challenge.
The Future of Adaptive AI
Zero-shot, one-shot, and few-shot learning techniques are at the forefront of driving artificial intelligence towards more adaptable and intuitive systems. By reducing the need for large labeled datasets, these approaches open doors to new possibilities. They enable AI systems to generalize and make predictions on unseen data, unlock novel applications, and reduce human reliance in the training process.
As the field of AI continues to evolve, we can expect further advancements in zero-shot, one-shot, and few-shot learning, ultimately leading us to smarter, more autonomous AI agents capable of understanding and adapting to the world around us.
Thank you for joining us on this exploration of zero-shot, one-shot, and few-shot learning. We hope this article has demystified these fascinating concepts, allowing you to grasp their potential and get a glimpse into the future of AI. Stay tuned for more enlightening definitions on our blog!