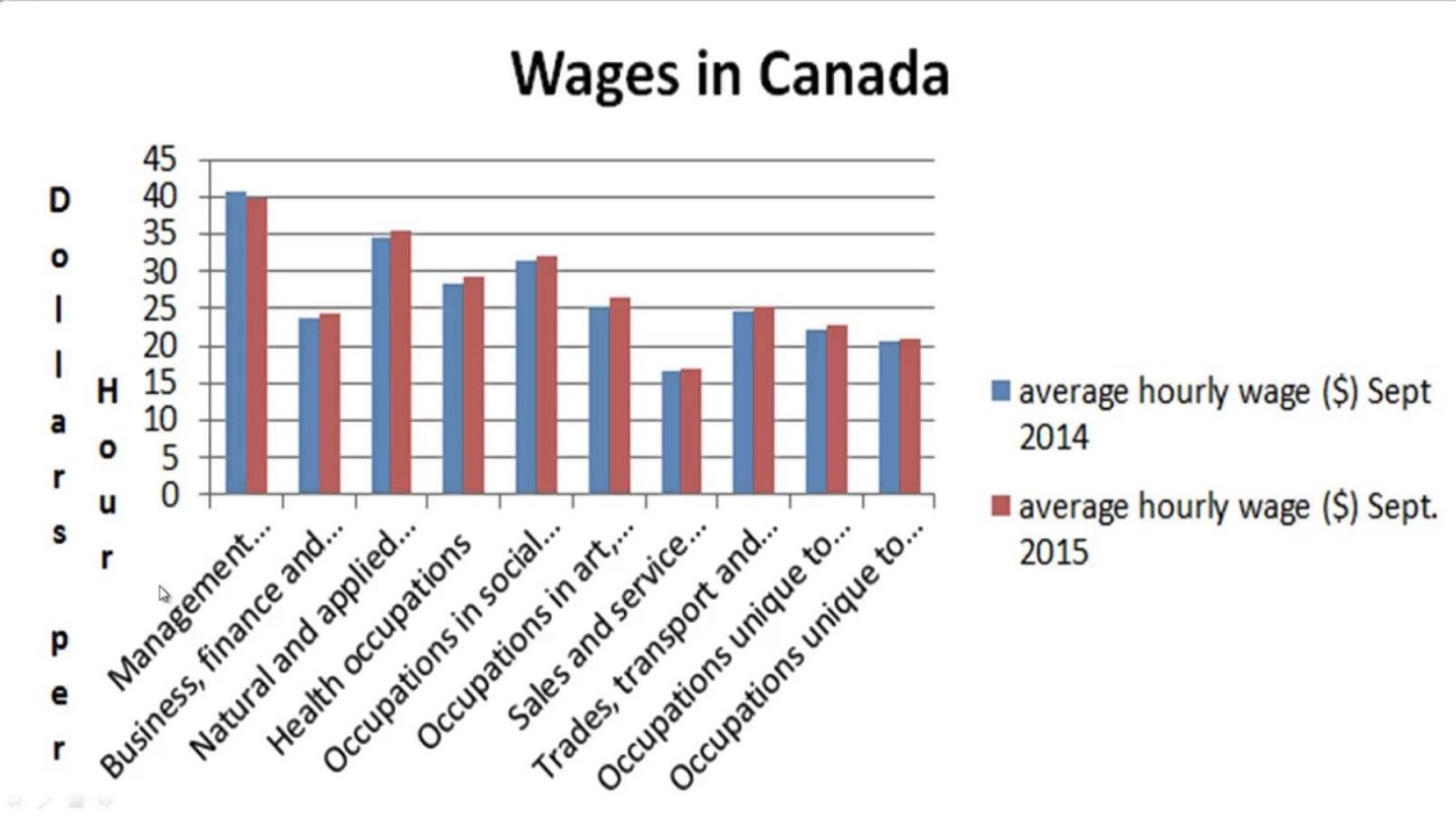
Interpreting data is a crucial skill in today’s data-driven world. Whether you’re a business owner analyzing sales numbers or a student looking at research data, knowing how to interpret data is essential. It involves understanding the patterns, trends, and insights hidden within the numbers to make informed decisions and gain a deeper understanding of the subject at hand. In this article, we will explore different techniques and strategies for interpreting data effectively. From understanding statistical measures to visualizing data through graphs and charts, we will cover everything you need to know to unlock the power of data analysis. So, let’s dive in and learn how to interpret data like a pro!
Inside This Article
- Importance of Data Interpretation
- Steps for Data Interpretation
- Common Data Interpretation Techniques
- Challenges in Data Interpretation
- Conclusion
- FAQs
Importance of Data Interpretation
Data interpretation is a crucial aspect of any data-driven field. It involves analyzing and making sense of data to uncover valuable insights, patterns, and trends. By interpreting data, professionals can make informed decisions, identify potential problems, and optimize processes. Whether you work in finance, marketing, healthcare, or any other industry, data interpretation is essential for driving success.
Here are the key reasons why data interpretation is important:
- Data-driven decision making: In today’s digital age, businesses accumulate vast amounts of data. However, without proper interpretation, this data is just noise. By interpreting data, businesses can make evidence-based decisions, leading to improved efficiency, cost savings, and better customer experiences.
- Trend identification: Data interpretation allows you to identify trends and patterns over time. This can be essential for understanding market behavior, customer preferences, or identifying emerging opportunities. By recognizing these trends, you can stay ahead of the competition and make strategic decisions.
- Problem detection: Through data interpretation, you can identify potential problems or anomalies in your data. This could be anything from identifying fraudulent activities to detecting product defects. By spotting these issues early on, you can take corrective action and prevent further complications.
- Performance evaluation: Data interpretation helps in evaluating performance and monitoring key metrics. By analyzing data, you can assess the effectiveness of your strategies, determine the success of your marketing campaigns, and measure the ROI of your investments. This information is essential for making improvements and optimizing future efforts.
- Enhanced forecasting: Interpreting historical data enables you to make accurate predictions and forecasts. With the help of data models and statistical techniques, professionals can anticipate market trends, demand patterns, and customer behavior. This information is invaluable for resource planning, inventory management, and financial forecasting.
Ultimately, data interpretation is crucial for unlocking the true potential of data and turning it into actionable insights. Without interpretation, data remains a collection of raw numbers and statistics, providing limited value. By leveraging data interpretation techniques and tools, professionals can gain a deeper understanding of their business and make informed decisions that drive success.
Steps for Data Interpretation
Data interpretation is a crucial process that involves analyzing and making sense of the data collected. To ensure accurate and meaningful interpretations, following a systematic approach can be beneficial. Here are the steps for effective data interpretation:
- Define the research question: Start by clearly defining the objective or research question that you want to address through the data. This will help guide your interpretation process.
- Review the data: Take the time to thoroughly review the data that you have collected. Understand the variables, their values, and the overall structure of the data set.
- Organize the data: Arrange the data in a structured manner that facilitates analysis. This may involve sorting, filtering, or grouping the data based on relevant criteria.
- Explore the data: Dive into the data by performing exploratory analysis. Look for patterns, trends, and outliers. Use visualizations such as charts and graphs to gain insights.
- Apply statistical techniques: Utilize statistical techniques to analyze the data and extract meaningful information. This may include calculating measures of central tendency, dispersion, correlation, or conducting hypothesis tests.
- Interpret the findings: Once the analysis is complete, interpret the findings by connecting them to the research question or objective. Clearly articulate the implications and significance of the results.
- Validate the interpretations: It is important to validate your interpretations by considering potential biases, limitations, or alternative explanations for the findings. Seek feedback from peers or experts in the field.
- Communicate the results: Finally, communicate your interpretations and findings effectively. This may involve creating reports, presentations, or visualizations that convey the insights in a clear and concise manner.
By following these steps, you can enhance the accuracy and reliability of your data interpretations. Remember, data interpretation is an iterative process, and it is essential to continuously refine your understanding as new insights emerge.
Common Data Interpretation Techniques
Data interpretation is a crucial skill in analyzing and understanding the information presented in datasets. Here are some commonly used techniques that can help you make sense of the data:
- Trend Analysis: This technique involves examining the data over a period of time to identify any patterns or trends. By plotting the data points on a graph or chart, you can visually assess the direction and magnitude of any changes.
- Correlation Analysis: Correlation analysis helps you understand the relationship between two or more variables in a dataset. By calculating correlation coefficients, you can determine if there is a positive, negative, or no correlation between the variables. This technique is particularly useful in identifying potential cause-and-effect relationships.
- Regression Analysis: Regression analysis is used to predict the value of a dependent variable based on the values of one or more independent variables. By fitting a regression line to the data, you can estimate the relationship and make predictions.
- Cluster Analysis: Cluster analysis is used to group similar data points together based on their characteristics. This technique is helpful when you want to identify different segments or patterns within a large dataset.
- Descriptive Statistics: Descriptive statistics provide summary measures that describe the main characteristics of a dataset. These measures include mean, median, mode, standard deviation, and percentiles. They help you understand the central tendency, dispersion, and distribution of the data.
- Probability Analysis: Probability analysis is used to assess the likelihood of an event occurring. By calculating probabilities, you can make informed decisions and assess the risk associated with certain outcomes.
- Histograms: Histograms are graphical representations that show the frequency distribution of a dataset. By dividing the data into intervals or bins, you can visualize how the values are distributed across the range.
- Pie Charts: Pie charts are circular diagrams that represent the relative proportions of different categories within a dataset. They are useful for visualizing the composition or distribution of data in a concise and easy-to-understand manner.
These are just a few of the common data interpretation techniques that can help you extract valuable insights from your datasets. Remember to choose the appropriate technique based on the nature of your data and the specific questions you want to answer.
Challenges in Data Interpretation
Data interpretation plays a crucial role in deriving meaningful insights from data. However, it is not without its challenges. Here are some of the common challenges encountered in data interpretation:
-
Data Quality: One of the primary challenges is ensuring the quality of the data being interpreted. Data may contain errors, inconsistencies, or missing values, leading to inaccurate interpretations. Therefore, it is important to thoroughly validate and clean the data before interpretation.
-
Data Complexity: Another challenge lies in dealing with complex and multidimensional data. In many cases, data may involve multiple variables and relationships, making it difficult to extract meaningful patterns or insights. Advanced techniques such as data mining or machine learning may be required to handle such complexity.
-
Data Volume: With the exponential growth of data, handling large volumes of data poses a significant challenge. As the volume of data increases, it becomes time-consuming and resource-intensive to process and interpret it. Implementing scalable and efficient data processing systems is necessary to address this challenge.
-
Data Integration: Organizations often have data scattered across various sources and systems. Integrating data from different sources, such as databases, spreadsheets, or external APIs, can be challenging. Inconsistent data formats, incompatible data structures, and data governance issues make the process of data integration complex.
-
Data Interpretation Bias: Human bias can also pose a challenge in data interpretation. Researchers or analysts may have preconceived notions or unconscious bias, which can lead to biased interpretations. It is important to be aware of such biases and strive for objectivity in data interpretation.
-
Data Visualization: Communicating the insights derived from data is another challenge in data interpretation. Presenting data in a visually appealing and easily understandable format is crucial for effective communication. Choosing the right visualization techniques and tools becomes essential to overcome this challenge.
Overcoming these challenges in data interpretation requires a combination of technical expertise, domain knowledge, and critical thinking. By being aware of these challenges and adopting appropriate strategies, organizations can successfully interpret data and make informed decisions.
Understanding how to interpret data is essential in today’s data-driven world. It allows us to make informed decisions, identify trends, and uncover valuable insights. By analyzing and deciphering data, we gain a deeper understanding of various aspects of our lives, whether it’s business, education, healthcare, or personal growth.
Interpreting data involves more than just looking at numbers. It requires critical thinking, attention to detail, and an understanding of the context in which data is collected and presented. It is important to consider factors such as data quality, sources, and potential biases to ensure accurate interpretations.
Furthermore, data interpretation is not a one-size-fits-all approach. Different industries and fields may require different techniques and methodologies. However, the fundamental principles of data interpretation, such as identifying patterns, making comparisons, and drawing logical conclusions, remain universal.
By becoming proficient in data interpretation, individuals and organizations can harness the power of data to drive innovation, improve decision-making processes, and enhance overall performance. So, whether you are a business professional, a student, or simply a curious individual, developing the skill to interpret data effectively is a valuable asset in today’s data-driven world.
FAQs
1. What is data interpretation?
Data interpretation is the process of analyzing and making sense of data to extract meaningful insights and draw conclusions. It involves organizing, cleaning, and transforming raw data into a format that can be easily understood and used to inform decision-making.
2. Why is data interpretation important?
Data interpretation is important because it allows businesses and individuals to make informed decisions based on reliable and accurate information. By analyzing data, patterns, trends, and relationships can be uncovered, leading to insights that can drive improvements, optimize processes, and identify opportunities for growth.
3. What are the steps involved in data interpretation?
The steps involved in data interpretation typically include:
- Defining the problem or objective
- Collecting relevant data
- Cleaning and preprocessing the data
- Exploring and visualizing the data
- Applying statistical techniques and models
- Interpreting and communicating the findings
4. What tools and techniques can be used for data interpretation?
There are a variety of tools and techniques that can be used for data interpretation, depending on the specific needs and goals. These may include statistical analysis methods, data visualization software, machine learning algorithms, and spreadsheet applications such as Microsoft Excel.
5. How can data interpretation be improved?
Data interpretation can be improved by:
- Ensuring data quality and accuracy
- Using appropriate data visualization techniques
- Employing statistical analysis methods
- Considering different perspectives and variables
- Seeking feedback and collaboration