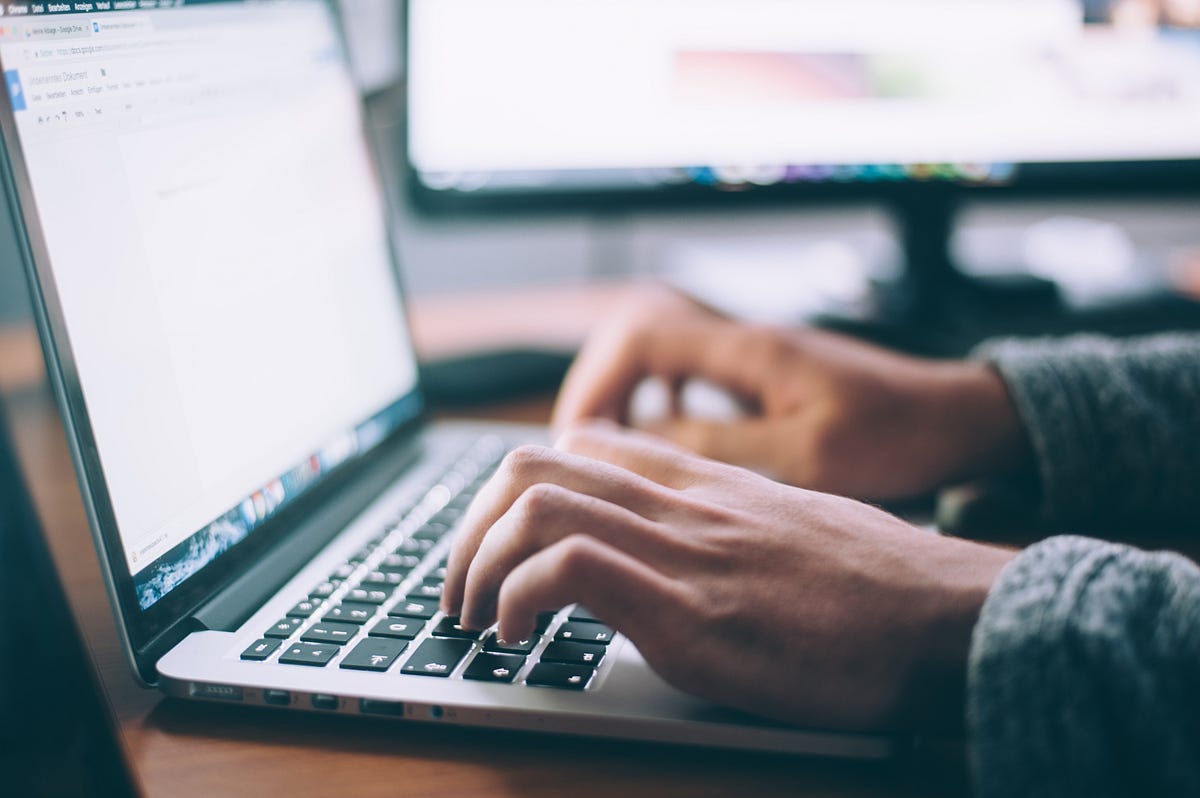
Data quality is a crucial aspect of any organization’s success. The accuracy, completeness, consistency, and reliability of data can significantly impact business operations and decision-making processes. With the increasing volume and complexity of data, ensuring high-quality data has become an even greater challenge. However, implementing effective strategies and practices can help improve data quality and ultimately drive better business outcomes. In this article, we will explore various methods and techniques to enhance data quality, from data cleansing and standardization to data governance and quality control. Whether you are a business owner, a data analyst, or an IT professional, understanding how to improve data quality is essential for maximizing the value of your data and gaining a competitive edge in today’s data-driven world.
Inside This Article
- Overview
- Importance of Data Quality
- Common Challenges in Data Quality Management
- Strategies to Improve Data Quality
- Conclusion
- FAQs
Overview
Data quality refers to the accuracy, completeness, reliability, and consistency of data. In today’s digital age, data has become a valuable asset for businesses of all sizes. However, the usefulness of data relies heavily on its quality. Poor data quality can lead to inaccurate insights, unreliable analysis, and inefficient decision-making. Therefore, improving data quality is crucial for organizations to maximize the value of their data and make informed business decisions.
Improving data quality involves various processes and techniques that aim to enhance the overall integrity of data. These processes include data cleansing, data validation, data profiling, and data governance. By implementing effective data quality management practices, businesses can ensure that their data is accurate, relevant, and reliable.
Furthermore, with the rise of big data and the increasing integration of data from multiple sources, ensuring data quality becomes even more challenging. Organizations need to deal with diverse data formats, large volumes of data, and real-time data streams. This necessitates the adoption of advanced technologies and tools that can efficiently manage and improve data quality.
Overall, improving data quality is not just a one-time task but an ongoing process. It requires a combination of data governance, data management practices, and the right technology infrastructure. By investing in data quality improvement initiatives, businesses can unlock the full potential of their data, gain valuable insights, and stay ahead in today’s competitive business landscape.
Importance of Data Quality
Data quality is of utmost importance in today’s data-driven world. It refers to the accuracy, completeness, reliability, and consistency of data. High-quality data ensures that organizations can make informed decisions, improve their operations, and achieve their business goals effectively. Let’s take a closer look at the key reasons why data quality is so essential:
1. Improved Decision Making: High-quality data acts as a reliable foundation for making informed decisions. When data is accurate, complete, and consistent, it enables organizations to analyze trends, identify patterns, and derive actionable insights. These insights can drive strategic business decisions and help in anticipating market trends.
2. Enhanced Customer Experience: Quality data is crucial for understanding customers and providing them with an exceptional experience. By having accurate customer information, organizations can personalize their offerings, address customer pain points, and deliver targeted marketing campaigns. This, in turn, enhances customer satisfaction and loyalty.
3. Better Operational Efficiency: Data quality plays a vital role in enhancing operational efficiency. Organizations rely on data for various processes, such as resource planning, inventory management, and supply chain optimization. When data is accurate and up-to-date, it enables streamlined operations, reduces errors, and improves overall productivity.
4. Regulatory Compliance: Many industries are subject to strict regulatory requirements regarding data management. High-quality data ensures that organizations comply with these regulations, avoid penalties, and build trust with customers and stakeholders. It helps in maintaining data privacy, security, and integrity.
5. Cost Savings: Poor data quality can result in significant financial losses. Data errors and inconsistencies can lead to incorrect invoicing, failed transactions, and inefficient resource allocation. By investing in data quality management, organizations can reduce costs associated with data errors, rework, and customer dissatisfaction.
6. Improved Business Performance: Ultimately, high-quality data drives improved business performance. It enables organizations to track key performance indicators, measure their success, and identify areas for improvement. With accurate and reliable data, businesses can optimize their processes, identify growth opportunities, and stay ahead of the competition.
Common Challenges in Data Quality Management
Data quality management is crucial for any organization that relies on accurate and reliable data to drive decision-making and operations. However, there are several common challenges that organizations face when it comes to managing data quality. Let’s explore some of these challenges:
1. Incomplete or missing data: One of the major challenges in data quality management is dealing with incomplete or missing data. This can occur due to various reasons, such as human error, system glitches, or data integration issues. Incomplete or missing data can lead to inaccurate analysis and flawed decision-making.
2. Data duplication: Another common challenge is the presence of duplicate data within the organization’s databases. Duplicate data can be caused by multiple data entry points or inadequate data consolidation processes. It can lead to confusion, inefficiency, and inconsistencies in reporting and analysis.
3. Inconsistent data formats: Data can be stored in various formats across different systems and departments within an organization. This inconsistency in data formats can make it difficult to integrate and analyze the data effectively. It can result in data errors and inconsistencies in reporting.
4. Data entry errors: Human errors during data entry can significantly impact data quality. Simple mistakes like typos, incorrect formatting, or incorrect data mapping can compromise the accuracy and reliability of the data. Proper training and data validation processes can help reduce these errors.
5. Lack of data governance: Data governance refers to the overall management of data within an organization, including data quality standards, policies, and procedures. Many organizations struggle with establishing robust data governance practices, which can result in inconsistent data quality across different departments and systems.
6. Data integration challenges: Integrating data from multiple sources can be a complex task. Incompatible data formats, data mapping issues, and data transformation challenges can hinder the data integration process and compromise data quality.
7. Data security and privacy concerns: Ensuring data security and privacy is another significant challenge in data quality management. Organizations need to comply with relevant data protection regulations and implement stringent security measures to safeguard sensitive data. Failure to address these concerns can not only compromise data quality but also expose the organization to legal and reputational risks.
8. Lack of data quality awareness: Lastly, a common challenge is the lack of awareness and understanding of the importance of data quality among stakeholders. This can lead to a lack of investment in data quality improvement initiatives and a lack of accountability for data quality issues.
Addressing these common challenges requires a proactive approach to data quality management. Organizations need to invest in robust data governance frameworks, implement data validation processes, and utilize data quality tools and technologies to ensure accurate and reliable data for decision-making.
Strategies to Improve Data Quality
Data quality plays a crucial role in the success of any organization. It ensures that the information being used for decision-making is accurate, reliable, and up-to-date. However, maintaining high-quality data can be challenging, especially with the increasing volume and complexity of data. To help you improve data quality, here are some effective strategies to implement:
1. Establish Data Governance:
Data governance involves creating a framework to manage data quality across the organization. This includes defining data standards, roles, and responsibilities, as well as establishing processes for data validation and verification. By implementing a robust data governance strategy, you can ensure that data quality is a priority and that everyone understands their role in maintaining it.
2. Conduct Regular Data Audits:
A data audit involves reviewing and analyzing the quality of your data. By conducting regular audits, you can identify any inconsistencies, errors, or inaccuracies in your data. This allows you to take corrective actions and implement preventive measures to improve data accuracy and completeness.
3. Implement Data Validation:
Data validation involves checking the accuracy and integrity of data during input and processing. By implementing validation rules and procedures, you can ensure that only valid and reliable data enters your systems. This can include checks for data type, range, format, and logical consistency.
4. Invest in Data Cleansing Tools:
Data cleansing tools help identify and correct errors, duplicates, and inconsistencies in your data. These tools employ algorithms and techniques to clean and standardize data, ensuring its accuracy and consistency. Investing in such tools can save time and effort while significantly improving the quality of your data.
5. Improve Data Entry Processes:
Data entry errors are a common cause of poor data quality. By improving data entry processes, such as implementing validation checks, providing training to data entry operators, and using automation tools, you can minimize errors at the point of entry, resulting in higher data quality.
6. Enhance Data Integration and Migration:
Data integration and migration projects often introduce data quality issues. It is essential to thoroughly validate and cleanse data before integrating or migrating it to new systems or databases. This ensures that the new environment is not contaminated with poor-quality data.
7. Foster Data Quality Culture:
Improving data quality requires a cultural shift within the organization. It is crucial to educate and train employees on the importance of data quality and their responsibility in maintaining it. Encourage a data-driven mindset and promote accountability for data quality across all levels of the organization.
By implementing these strategies, you can significantly improve the quality of your data, leading to better decision-making, improved operational efficiency, and enhanced customer experiences.
Conclusion
In conclusion, improving data quality is crucial for businesses in order to ensure accurate, reliable, and meaningful insights. By implementing the best practices and strategies mentioned in this article, businesses can effectively address data quality issues and optimize their decision-making processes. It is essential to establish a data governance framework, clean and validate data regularly, invest in advanced technologies and tools, and prioritize data quality throughout the organization. Moreover, fostering a culture of data quality and providing adequate training to employees can contribute to maintaining high standards in data management. Remember, data is the foundation for successful business operations and future growth, so it is worth investing time, resources, and efforts in enhancing data quality. By doing so, businesses can unlock the full potential of their data and gain a competitive edge in today’s data-driven world.
FAQs
Q: How can I improve data quality?
Improving data quality can be achieved through various means such as data cleansing, data validation, and regular data audits. Implementing robust data governance practices and investing in advanced data management tools can also greatly enhance data quality.
Q: What is data cleansing?
Data cleansing is the process of identifying and correcting or removing inaccurate, incomplete, or inconsistent data from a database. This involves tasks such as deduplication, data standardization, and data enrichment to ensure that data is accurate, consistent, and up to date.
Q: How does data validation contribute to data quality?
Data validation involves the verification of data to ensure that it adheres to predetermined standards and rules. Validating data helps identify and rectify any errors or inconsistencies, ensuring that only reliable and accurate data is stored in the system, thus improving overall data quality.
Q: Why are data audits important for maintaining data quality?
Data audits involve conducting a comprehensive examination of data sets to assess their accuracy, completeness, and compliance with regulations or standards. Regular data audits help identify and correct any anomalies, errors, or gaps in data, ensuring that data quality is consistently maintained over time.
Q: How can data governance practices improve data quality?
Data governance refers to the framework and processes that govern the management of data within an organization. By establishing clear ownership, accountability, and standardization of data management practices, data governance ensures that data quality is a priority. It helps define roles and responsibilities, promotes data stewardship, and establishes guidelines for data quality monitoring and improvement.