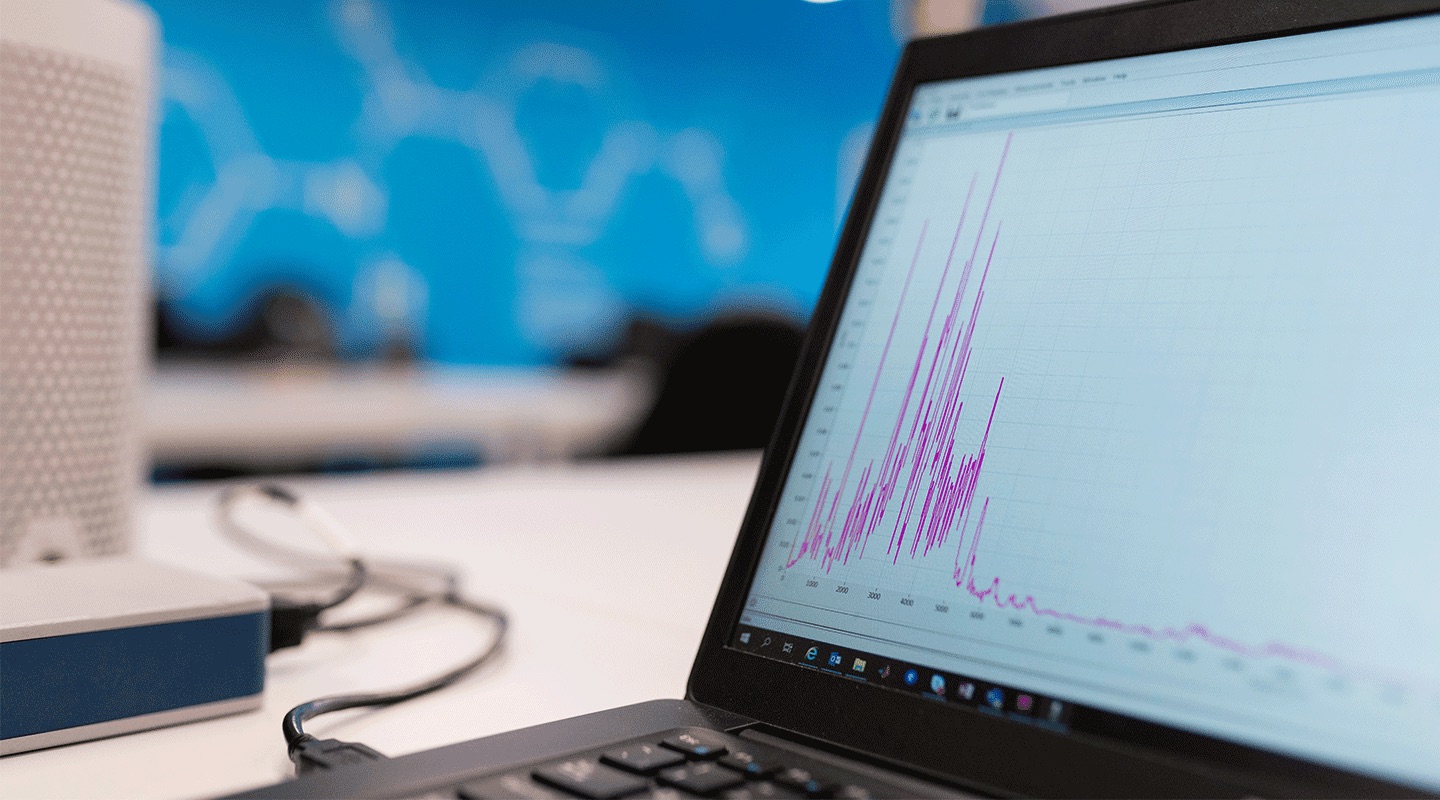
Data quality is an essential aspect of any business operation, ensuring that the information used for decision-making and analysis is accurate, reliable, and up-to-date. In today’s digital era, where data is generated and consumed at an unprecedented rate, maintaining data quality has become increasingly challenging. However, it is crucial for businesses to invest time and resources in establishing strong data governance practices to ensure the integrity of their data.
In this article, we will explore the importance of data quality and provide valuable insights and strategies on how to maintain it. From establishing data quality frameworks to implementing data validation processes, we will delve into the best practices that businesses can adopt to enhance data accuracy, completeness, consistency, and reliability. By implementing these strategies, businesses can make confident decisions, improve efficiencies, and gain a competitive edge in today’s data-driven world.
Inside This Article
- Understanding the Importance of Data Quality
- Establishing Data Quality Standards
- Implementing Data Validation Techniques
- Regular Data Cleaning and Maintenance
- Conclusion
- FAQs
Understanding the Importance of Data Quality
Data quality is the backbone of any successful business. It refers to the accuracy, completeness, consistency, and reliability of the data collected and stored by an organization. In today’s digital age, where companies rely heavily on data-driven decision making, maintaining high-quality data is crucial for making accurate and informed business decisions.
Poor data quality can have severe consequences for an organization. Inaccurate or incomplete data can lead to flawed analysis, misleading insights, and ultimately, poor decision making. It can also result in wasted resources, inefficient processes, and missed opportunities.
On the other hand, when data quality is high, organizations can benefit in several ways. High-quality data ensures that the information used for analysis and decision making is accurate and reliable, leading to more effective strategies and better outcomes. It helps identify trends, patterns, and customer behavior, allowing businesses to tailor their products and services to meet customer demands.
Furthermore, data quality is also essential for regulatory compliance. Many industries, such as healthcare and finance, have strict regulations regarding data security and privacy. Poor data quality can result in non-compliance with these regulations, potentially leading to legal issues and financial penalties.
Overall, understanding and prioritizing data quality is vital for organizations in today’s data-driven business landscape. By investing in data quality management, implementing data validation techniques, and regularly cleaning and maintaining data, businesses can ensure that they have accurate, reliable, and actionable information to drive their decision-making processes.
Establishing Data Quality Standards
In the world of data analytics, the importance of data quality cannot be emphasized enough. High-quality data is essential for making informed decisions, identifying trends, and gaining valuable insights. To ensure the integrity and reliability of your data, it is crucial to establish data quality standards. Let’s explore some key steps in this process.
1. Define the objectives: Start by clearly defining the objectives of your data quality initiative. Identify the specific goals you want to achieve, such as improving data accuracy, completeness, consistency, and timeliness.
2. Determine data quality metrics: Once your objectives are established, you need to define the metrics that will be used to measure the quality of your data. These metrics can vary depending on your organization’s specific requirements. Common metrics include data accuracy, completeness, validity, consistency, relevance, and uniqueness.
3. Set data quality thresholds: Once you have chosen your data quality metrics, it is important to set realistic thresholds. These thresholds will define the acceptable levels for each metric. For example, you may decide that a data accuracy threshold of 95% is acceptable for your organization.
4. Establish data quality rules: Data quality rules are guidelines that ensure data consistency and accuracy. These rules define how data should be structured, formatted, and validated. They can range from simple rules, such as ensuring that a phone number field contains only numbers, to more complex rules involving data relationships and dependencies.
5. Implement data profiling and monitoring: Data profiling involves analyzing the content and structure of your data to identify any anomalies or issues. By conducting regular data profiling, you can identify data quality issues and take corrective actions. Data monitoring involves ongoing monitoring of your data to ensure that it meets the defined quality standards.
6. Establish data governance processes: Data governance is the process of managing the availability, usability, integrity, and security of data in your organization. Establishing data governance processes ensures that data quality standards are maintained consistently across the organization. This includes defining roles and responsibilities, creating data quality policies, and implementing data stewardship practices.
7. Educate and train stakeholders: Data quality is a collective responsibility that involves various stakeholders within an organization. It is important to educate and train stakeholders on the importance of data quality and their role in maintaining it. This can include providing training sessions, creating awareness campaigns, and developing documentation and guidelines.
Implementing Data Validation Techniques
Implementing data validation techniques is crucial to ensure the accuracy, integrity, and reliability of your data. By validating your data, you can identify and eliminate any errors, inconsistencies, or discrepancies that may exist. Here are some key techniques to consider:
1. Field Validation: This technique involves checking individual fields within your data for accuracy and completeness. You can apply checks such as data type validation, range validation, and format validation to ensure that the data entered meets the specified criteria.
2. Cross-Field Validation: Cross-field validation involves validating the relationship between multiple fields in your data. This technique helps to identify any inconsistencies or conflicts that may exist between different data elements. For example, if you have a database that records customer addresses, you can cross-validate the postal code, city, and state fields to ensure that they match with each other.
3. Code Validation: Code validation involves verifying the accuracy and validity of codes used in your data. For example, if you have a dataset that includes product codes, you can validate these codes against a predefined set of valid codes to ensure that they are correctly entered.
4. Range Validation: Range validation involves checking if the values in your data fall within a specified range or set of values. For instance, if you have a dataset of ages, you can validate that the ages recorded are within a certain range, such as 18 to 65 years old.
5. Format Validation: Format validation focuses on ensuring that the data follows a specific format or pattern. For example, if you have a dataset containing phone numbers, you can validate that the phone numbers are entered in the correct format with the appropriate number of digits or separators.
6. Duplicate Data Checking: Duplicate data checking involves detecting and removing duplicate entries within your dataset. This technique is essential to maintain data integrity and avoid data redundancy. By identifying and removing duplicates, you can ensure that your data is accurate and reliable.
7. Real-Time Validation: Real-time validation is performed at the point of data entry to prevent incorrect or incomplete data from being entered into the system. By validating the data in real-time, you can prompt users to correct any errors or inconsistencies immediately, ensuring that only high-quality data is added to your database.
By implementing these data validation techniques, you can significantly improve the quality of your data and increase its trustworthiness. This, in turn, will enhance decision-making processes and drive better business outcomes.
Regular Data Cleaning and Maintenance
Regular data cleaning and maintenance is essential to ensure the accuracy and integrity of your data. Here are some best practices to follow:
1. Review Data on a Regular Basis: Set a schedule to review your data at regular intervals. This will help you identify any errors, inconsistencies, or outdated information that may affect the quality of your data.
2. Remove Duplicate Records: Duplicate records can lead to confusion and inaccuracies. Utilize data cleansing tools or write custom scripts to identify and remove duplicate entries from your database.
3. Standardize Data Formats: Inconsistent data formats can make it difficult to analyze and use data effectively. Standardize formats for fields such as phone numbers, addresses, and dates to ensure consistency across your data sets.
4. Validate Data: Implement data validation techniques to ensure that the data entered into your system meets specified criteria. This can include checking for valid email addresses, phone numbers, or zip codes.
5. Update Outdated Information: Keep a close eye on outdated data, such as contact information or product details. Regularly update this information to maintain accuracy and relevancy.
6. Back Up Your Data: Regularly back up your data to prevent loss and ensure data integrity. This will help you recover in case of accidental deletion or system failures.
7. Train Your Staff: Educate your staff on the importance of data quality and provide training on data entry and maintenance processes. This will help ensure that everyone follows best practices and contributes to maintaining data quality.
8. Monitor Data Quality: Develop metrics and monitor data quality regularly. Establish key performance indicators (KPIs) to track the accuracy, completeness, and consistency of your data.
9. Seek Feedback from Users: Encourage your users to provide feedback on data quality issues they encounter. This feedback can help identify areas for improvement and enhance the overall quality of your data.
10. Stay Updated on Industry Standards: Keep yourself informed about the latest best practices and industry standards in data cleaning and maintenance. Subscribe to industry publications and attend relevant conferences or webinars to stay up to date.
By following these regular data cleaning and maintenance practices, you can ensure that your data remains accurate, reliable, and up to date. Incorporating these practices into your data management strategy will lead to better decision-making and more successful outcomes for your business.
Conclusion
Ensuring data quality is vital for any organization that wants to operate efficiently and make informed decisions. By implementing the right practices, such as data cleansing, validation, and regular monitoring, companies can minimize errors and maximize the value of their data.
Effective data quality management requires a combination of tools, processes, and dedicated teams. The use of advanced technologies like artificial intelligence and machine learning can further enhance data quality efforts.
Remember, data quality is an ongoing process that requires continuous attention and improvement. It’s crucial to establish clear data quality standards, involve stakeholders, and regularly assess the effectiveness of your data quality initiatives.
By maintaining high-quality data, organizations can gain a competitive edge, make better decisions, and drive growth and success in today’s data-driven world.
FAQs
Q: Why is data quality important?
Data quality is important because it ensures that the data being used for analysis, decision-making, and other business processes is accurate, reliable, and up-to-date. Poor data quality can lead to incorrect insights, inefficient processes, and costly errors.
Q: How can I assess the quality of my data?
There are several ways to assess data quality. One common approach is to evaluate the data based on its accuracy, completeness, consistency, timeliness, and relevance. This can be done through data profiling, data cleansing, and data validation techniques.
Q: What are some common causes of poor data quality?
Poor data quality can be caused by various factors, including human error during data entry, system glitches, outdated data integration processes, lack of data governance, and insufficient data validation checks. It’s important to identify and address these root causes to improve data quality.
Q: How can I improve data quality?
Improving data quality requires a systematic approach. This includes establishing data governance practices, implementing data quality tools and processes, conducting regular data audits, training data users on data entry best practices, and continuously monitoring data quality.
Q: What are the benefits of maintaining good data quality?
Maintaining good data quality has several benefits. It improves decision-making accuracy, enhances operational efficiency, increases customer satisfaction, enables regulatory compliance, and boosts overall business performance. High-quality data is a valuable asset that can drive success in today’s data-driven world.