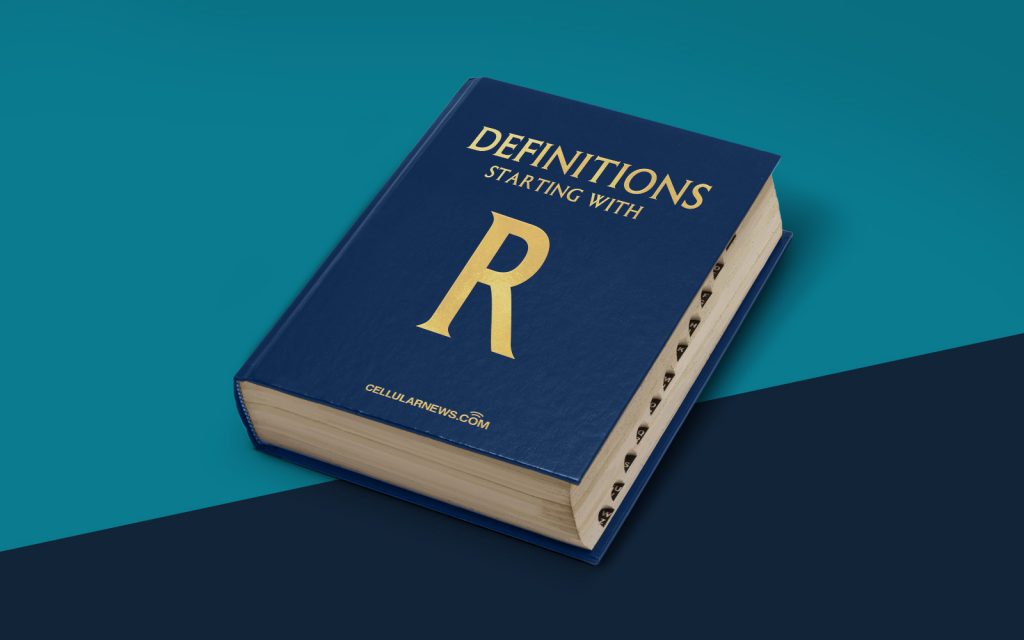
What is a Recommendation Engine?
Welcome to the “Definitions” category of our page! In this section, we break down complex terms and concepts to help you gain a better understanding of them. Today, we will dive into the world of recommendation engines.
Do you ever wonder how online platforms like Amazon, Netflix, or Spotify seem to magically know what you want to buy, watch, or listen to next? The secret lies in the powerful algorithms behind recommendation engines.
Key Takeaways:
- Recommendation engines are sophisticated algorithms that analyze user data to provide personalized suggestions.
- These engines are widely used in various industries, including e-commerce, streaming services, and social media platforms.
Now let’s dig deeper and demystify the concept of recommendation engines. At its core, a recommendation engine is a software system that collects and analyzes vast amounts of data about users’ preferences, behaviors, and interactions. By leveraging this data, the engine generates personalized recommendations tailored to each user’s unique taste and interests.
There are primarily two types of recommendation engines: collaborative filtering and content-based filtering. Collaborative filtering relies on the collective wisdom of the crowd by comparing users’ behaviors and preferences to predict their future choices. On the other hand, content-based filtering examines the characteristics and attributes of items to recommend similar ones to users.
So, how do recommendation engines work? Here’s a simplified step-by-step process:
- Data Collection: The engine gathers data from various sources, such as user profiles, browsing history, purchase history, ratings, and social media interactions.
- Data Preprocessing: The collected data is cleaned, transformed, and prepared for analysis. This step ensures that the data is accurate and relevant.
- Feature Extraction: The engine identifies important features or characteristics of items and users. It could be genre, artist, price range, or demographic information.
- Algorithm Selection: Based on the type of recommendation engine, an appropriate algorithm is chosen to generate recommendations.
- Training the Model: The algorithm is trained using historical user data, learning patterns, and associations between user preferences and item attributes.
- Generating Recommendations: Once the model is trained, the engine can generate personalized recommendations for each user based on their data.
Recommendation engines offer several benefits both for businesses and users. For businesses, they can boost customer engagement, increase sales, and improve customer satisfaction by offering relevant and personalized recommendations. Users, on the other hand, benefit from a curated selection of items, saving time and discovering new products or content that align with their interests.
In conclusion, recommendation engines are powerful tools that help businesses deliver personalized experiences to their users. By analyzing user data and leveraging sophisticated algorithms, these engines have become essential in the digital landscape. From suggesting your next favorite book to finding the perfect movie or song, recommendation engines enhance our online experiences and make our lives a little bit easier.
Key Takeaways:
- Recommendation engines analyze user data to provide personalized recommendations.
- There are two main types of recommendation engines: collaborative filtering and content-based filtering.
We hope this article has clarified what a recommendation engine is and how it works. If there are any other terms or concepts you’d like us to explain, feel free to reach out. Happy exploring!