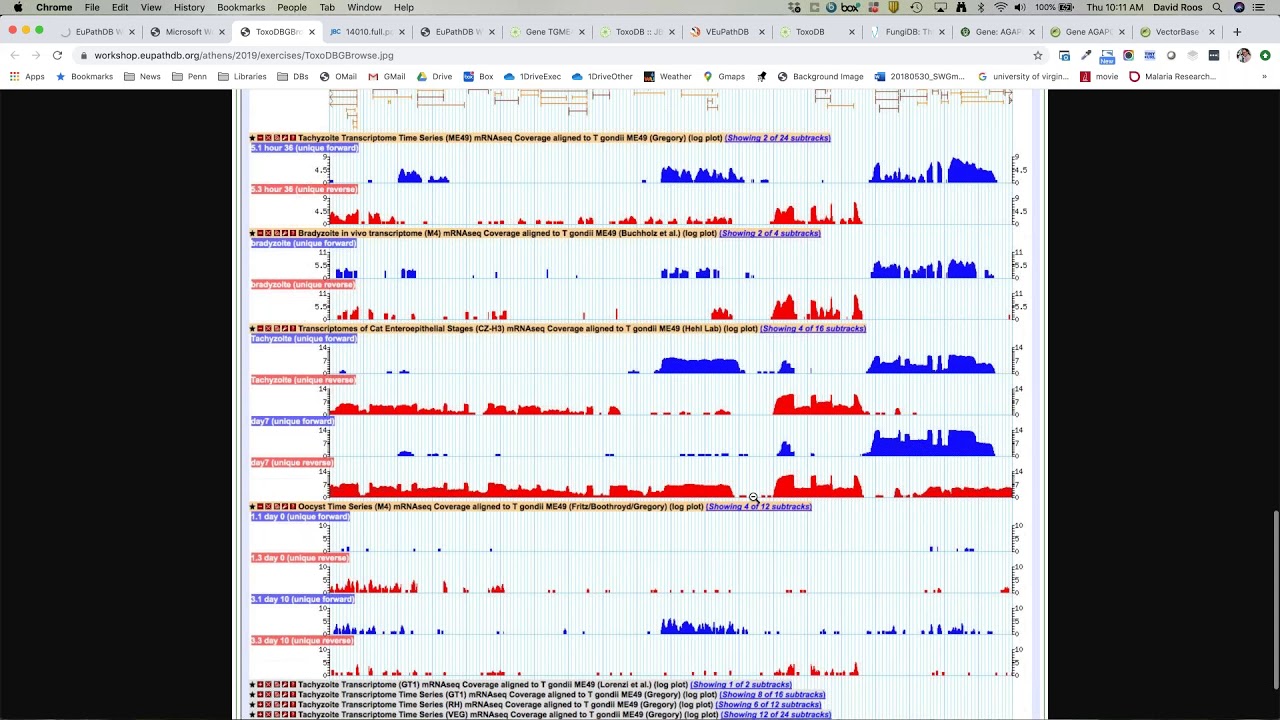
Interpreting RNA-Seq data is a crucial task in the field of genomics. This high-throughput sequencing method allows scientists to gain valuable insights into the transcriptome – the complete set of RNA molecules in a cell or organism at a given time. By analyzing RNA-Seq data, researchers can decipher gene expression levels, identify novel transcripts, and detect alternative splicing events.
However, interpreting RNA-Seq data can be complex and challenging. It requires understanding the experimental design, quality control measures, data preprocessing, and advanced statistical analysis methods. Additionally, knowledge of bioinformatics tools and databases is essential to extract meaningful information from the vast amount of sequencing data.
In this article, we will guide you through the process of interpreting RNA-Seq data step-by-step. From data preprocessing to differential expression analysis, we will cover the essential concepts and techniques necessary to make sense of your RNA-Seq experiment’s results.
Inside This Article
- Overview of RNA Sequencing
- Pre-processing and Quality Control of RNA-Seq Data
- Alignment and Mapping of RNA-Seq Reads
- Quantification and Differential Expression Analysis of RNA-Seq Data
- Conclusion
- FAQs
Overview of RNA Sequencing
RNA sequencing, also known as RNA-Seq, has revolutionized the field of genomics. It is a powerful technique that allows researchers to study the entire transcriptome of an organism, providing insights into gene expression, alternative splicing, and identification of novel transcripts.
RNA-Seq begins with the extraction of RNA molecules from the biological sample of interest. These RNA molecules represent the active genes in the cells and tissues being studied. The extracted RNA is then converted into complementary DNA (cDNA), which serves as a template for the sequencing process.
Next-generation sequencing (NGS) platforms are commonly used for RNA-Seq, as they offer high-throughput sequencing and allow the generation of millions of short reads simultaneously. This enables comprehensive profiling of gene expression and the identification of rare or low-abundance transcripts.
During the sequencing process, the cDNA molecules are fragmented and attached to sequencer-specific adapters. These adapters contain short DNA sequences that are necessary for the attachment of the cDNA to the sequencing platform. The fragmented cDNA molecules are then amplified and undergo high-throughput sequencing.
Once the sequencing is complete, the generated reads are subjected to a series of computational analyses. These analyses include pre-processing and quality control, alignment and mapping to a reference genome, and quantification and differential expression analysis of the sequenced transcripts.
RNA-Seq has numerous applications in various fields of biology and medicine. It is commonly used to study gene expression changes in different tissues, developmental stages, or disease conditions. It can also help in identifying novel transcripts, detecting alternative splicing events, and understanding the regulation of gene expression.
Overall, RNA sequencing provides a comprehensive view of the transcriptome and offers valuable insights into the dynamic nature of gene expression. It has transformed the field of genomics and continues to shape our understanding of complex biological processes.
Pre-processing and Quality Control of RNA-Seq Data
Before diving into the analysis of RNA-Seq data, it is crucial to properly preprocess and perform quality control on the raw data to ensure reliable and accurate results. Preprocessing involves a series of steps to transform the raw sequencing reads into a format suitable for downstream analysis.
The first step in pre-processing is the removal of any adapter sequences or low-quality bases from the reads. Adapters are short DNA sequences added to the ends of fragments during library preparation, and their removal is necessary to obtain accurate read alignments. Additionally, low-quality bases, which are caused by sequencing errors or degradation during library preparation, can introduce noise and inaccuracies into the data.
After adapter removal and trimming, the next step is to perform quality control checks. Quality control assesses the overall quality of the sequencing data and detects any potential issues, such as poor sequencing depth or high levels of sequencing errors. Common quality control metrics include per base sequence quality scores, per base sequence content, GC distribution, and duplication levels.
Quality control can be performed using various tools and software packages, such as FastQC. These tools generate graphical plots and summary statistics that help identify any problematic samples or technical issues. If any issues are detected, appropriate corrective measures can be taken, such as re-sequencing or reprocessing the samples.
Once the data has undergone quality control, the reads need to be aligned or mapped to a reference genome or transcriptome. Alignment involves assigning each read to its corresponding position in the reference genome or transcriptome. The alignment step is crucial as it determines the accuracy of downstream analyses such as gene expression quantification and differential expression analysis.
There are several alignment algorithms available, including Bowtie, HISAT2, and STAR, each with its strengths and limitations. The choice of alignment algorithm depends on the specific research question and the characteristics of the data, such as read length and sequencing depth.
After alignment, it is essential to quantify gene expression levels from the aligned reads. This step involves assigning reads to specific genes or transcripts and estimating their abundance. Various methods for quantification, such as featureCounts and HTSeq, are available, each with its unique approach.
Finally, once the gene expression levels have been quantified, the data can be further analyzed for differential expression. Differential expression analysis compares the gene expression levels between different conditions or treatments to identify genes that are significantly upregulated or downregulated. This analysis provides insights into the biological processes and genetic mechanisms at play.
Alignment and Mapping of RNA-Seq Reads
Alignment and mapping of RNA-Seq reads is a crucial step in the analysis of RNA sequencing data. This process involves aligning the short sequencing reads obtained from the RNA-Seq experiment to a reference genome or transcriptome. The goal is to determine the origin and position of each read in the genome or transcriptome, allowing for further analysis such as quantification and identification of differentially expressed genes.
To perform alignment and mapping, specialized software tools are used. These tools utilize algorithms and indexes to efficiently match the sequencing reads to the reference sequence. One commonly used tool for this task is the Burrows-Wheeler Aligner (BWA). BWA employs the Burrows-Wheeler transform to quickly align the reads to a given reference genome.
During the alignment process, the software takes into account factors such as sequencing errors, base quality scores, and the presence of genomic variations (like single-nucleotide polymorphisms) to accurately map each read. The alignment algorithm generates a mapping file, which records the alignment information for each read, including the aligned position, alignment quality, and any mismatches or gaps.
Once the reads are aligned and mapped, researchers can perform various downstream analyses. One of the primary applications is the quantification of gene expression levels. By counting the number of reads mapped to each gene, researchers can determine the relative abundance of transcripts and identify genes that are upregulated or downregulated under specific conditions.
In addition to gene expression analysis, the mapped reads can also be used for other analyses such as splice variant detection, fusion gene identification, and genomic variant calling. These analyses provide valuable insights into the structure and function of the transcriptome and can help uncover novel biomarkers or potential therapeutic targets.
It is worth noting that the accuracy and reliability of the alignment and mapping step greatly influence the downstream analysis results. Different alignment algorithms and parameter settings may yield slightly varying results, so it is important to carefully consider the choice of alignment tool and optimize the parameters based on the specific characteristics of the data and the research question.
Quantification and Differential Expression Analysis of RNA-Seq Data
Once the RNA-Seq reads have been aligned and mapped, the next step is to quantify the expression levels of genes and identify any differences in expression between different experimental conditions. This process is known as quantification and differential expression analysis of RNA-Seq data.
Quantification involves estimating the abundance of each transcript or gene in the sample based on the number of RNA-Seq reads that align to it. This can be done using various algorithms, such as the widely used method known as the reads per kilobase per million mapped reads (RPKM) or its updated version, called transcripts per million (TPM). These methods take into account both the length of the gene and the total number of reads in the sample to calculate the expression level.
Once the expression levels have been quantified, the next step is to identify genes that are differentially expressed between different conditions or groups. This is typically done using statistical tests, such as the popular edgeR or DESeq2 packages. These methods take into account the variation in gene expression between replicates and calculate the statistical significance of the differences in expression between the groups.
The results of the differential expression analysis typically include a list of genes that are significantly upregulated or downregulated, along with their corresponding fold change and p-value. The fold change represents the magnitude of the change in expression between the groups, while the p-value indicates the statistical confidence in the difference.
It is important to note that the interpretation of the results of the differential expression analysis should be done with caution. Other factors, such as biological variability and the experimental design, should be taken into consideration to ensure the validity of the findings. It is also recommended to perform additional analyses, such as pathway enrichment analysis or gene ontology analysis, to gain a better understanding of the biological implications of the differentially expressed genes.
The field of RNA sequencing (RNA-seq) has revolutionized the way we study and understand gene expression patterns. By analyzing the transcriptome at a large scale, RNA-seq provides a comprehensive and detailed view of the genes and their expression levels in a biological sample. Throughout this article, we have explored the various steps involved in interpreting RNA-seq data, from data generation to analysis and visualization.
Having a solid understanding of the different bioinformatics tools and techniques used in RNA-seq analysis is key to unlocking valuable insights and discoveries. By leveraging the power of statistical algorithms, differential gene expression analysis, and functional enrichment analysis, researchers can identify differentially expressed genes and gain insights into biological processes and pathways that may be altered under different conditions.
Moreover, the emergence of advanced technologies, such as single-cell RNA-seq, has paved the way for uncovering cell heterogeneity and exploring the nuances of gene expression at a single-cell level. This provides a deeper understanding of cellular identities and dynamics.
In conclusion, RNA-seq data analysis allows scientists to delve deeper into the intricacies of gene expression and unravel the mysteries of biology. By combining computational approaches with biological knowledge, researchers can unlock new insights, leading to breakthrough discoveries in fields such as genomics, precision medicine, and developmental biology.
FAQs
1. What is RNA-seq data?
RNA-seq data refers to the data generated from RNA sequencing experiments. It provides comprehensive information about the complete set of RNA molecules present in a sample, allowing researchers to study gene expression levels and identify differentially expressed genes.
2. How is the quality of RNA-seq data assessed?
The quality of RNA-seq data is assessed through various metrics such as read quality, sequencing depth, and alignment rates. Quality checks involve examining sequence read quality scores, assessing the percentage of reads that align to a reference genome, and evaluating the coverage and uniformity of mapped reads across genes.
3. What is the purpose of RNA-seq data analysis?
RNA-seq data analysis enables researchers to gain insights into biological processes, pathways, and gene expression patterns. It can help identify differentially expressed genes between different conditions, discover new transcript variants, annotate gene structures, and study alternative splicing events.
4. What software tools are commonly used for analyzing RNA-seq data?
There are several popular software tools used for analyzing RNA-seq data, including DESeq2, edgeR, and limma, which are commonly used for differential gene expression analysis. Other tools like Cufflinks, StringTie, and Salmon are utilized for transcript assembly and quantification.
5. What are the challenges in analyzing RNA-seq data?
Analyzing RNA-seq data can be challenging due to various factors such as data heterogeneity, bias, and normalization issues. Additionally, issues related to batch effects, multiple testing, and the need for appropriate statistical methods pose challenges in accurately interpreting RNA-seq data.