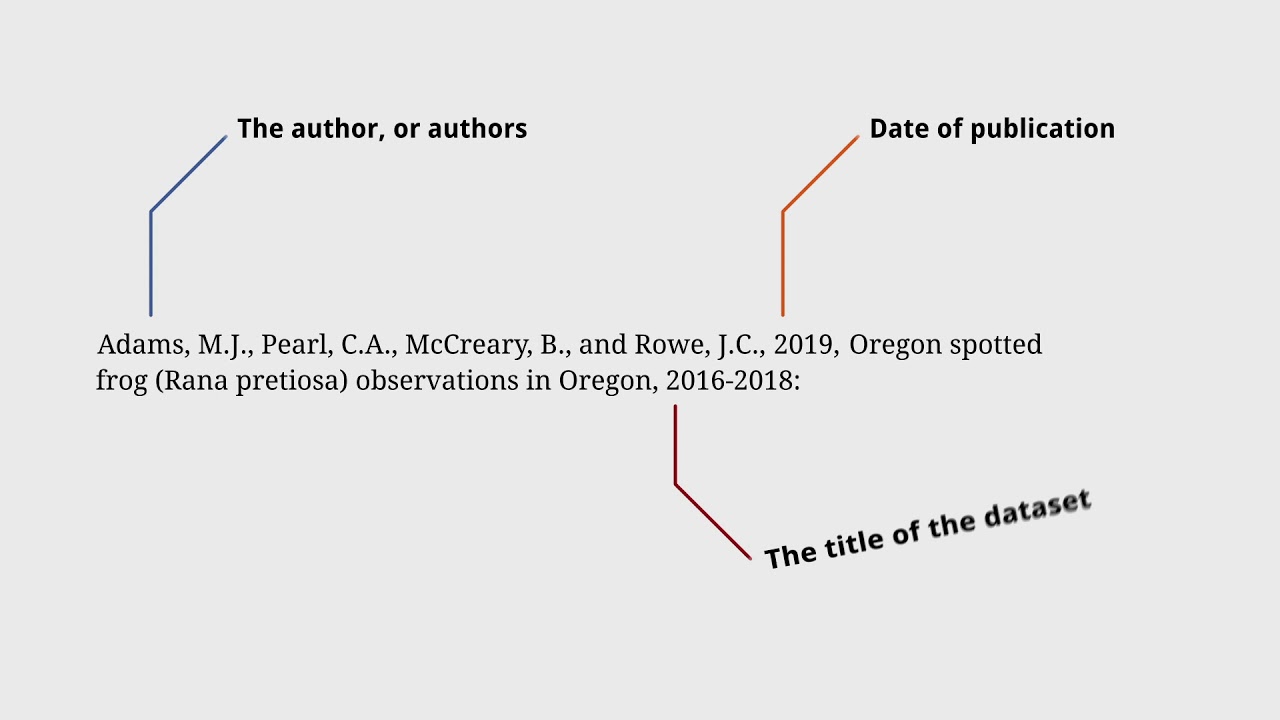
In the age of technology and information, data plays a crucial role in shaping our understanding of the world. Whether you are writing a research paper, conducting a study, or simply wanting to provide proper credit to the data you are referencing, knowing how to cite data is essential. Citing data not only enhances the credibility and reliability of your work, but it also allows others to easily find and verify the information you have used. In this article, we will explore the importance of citing data, the different citation styles, and step-by-step instructions on how to properly cite data in your research. So, let’s dive in and learn how to effectively cite data to enhance the quality of your work.
Inside This Article
- Overview
- Types of Data Citation
- Guidelines for Data Citation
- Examples of Data Citation Styles
- Conclusion
- FAQs
Overview
Data citation is the process of attributing credit to the creators of a dataset by referencing it in a standardized and structured manner. It plays a crucial role in acknowledging the contributions of researchers and promoting the transparency, reproducibility, and accountability of scientific work.
Properly citing data is essential for several reasons. Firstly, it allows other researchers to easily access and verify the data used in a study. This facilitates collaboration, encourages reproducibility, and enables the advancement of knowledge in the field. Secondly, data citation provides a way to give credit to the original data creators, recognizing their efforts and contributions to the scientific community. Lastly, data citation is important for establishing the intellectual property rights and ownership of datasets, ensuring fair use and proper acknowledgement of authors’ work.
In recent years, the importance of data citation has gained recognition, and various organizations and institutions have developed guidelines and standards to facilitate the proper citation of data. These guidelines typically include information such as the dataset creator’s name, title of the dataset, publication or release date, version number, and persistent identifier such as a DOI (Digital Object Identifier) or a URL.
Understanding how to cite data is crucial for researchers, especially in disciplines that heavily rely on data-driven research. By properly citing datasets, researchers contribute to the integrity of the research community and help build a robust knowledge base for future scientific endeavors.
Types of Data Citation
Data citation is a crucial element in research and scholarly publishing, as it allows for the proper attribution and recognition of the datasets used in a study. There are different types of data citation styles that researchers and scholars can utilize, depending on the specific requirements of their field or publication.
1. Author-Date Style: This type of data citation style follows the format used in the social sciences and humanities, where the author’s last name and the publication year are included in the citation. For example, (Smith, 2021).
2. Numeric Style: Numeric data citation is commonly used in the sciences, particularly in fields such as biology, chemistry, and physics. In this style, numerical references are used within the text, and a corresponding number is listed in the reference section. For example, [1].
3. Name-Year Style: Name-year data citation is similar to author-date style, but instead of using parentheses, the author’s last name and the publication year are listed within the text. For example, Smith (2021).
4. Institutional Style: In some cases, an institution or organization may have its own specific data citation style. This style is often used when citing data that has been produced or collected by a specific institution, such as a government agency or research organization. It may include additional information, such as the department or division responsible for the data.
5. URL Style: With the increasing availability of online databases and repositories, data can often be accessed through a URL. This type of data citation includes the URL of the dataset, allowing readers to directly access the data source. It is commonly used in online publications and websites.
6. Dataset Identifier Style: Some fields, such as genomics or linguistics, have specific formats for citing datasets. These styles often involve the use of unique identifiers assigned to each dataset, such as accession numbers or persistent identifiers (PIDs).
It is important to note that the appropriate data citation style to use may vary depending on the requirements of the publication or journal. Researchers should consult the specific guidelines provided by the publisher or refer to established citation style manuals, such as APA or MLA, for guidance on how to cite data in their respective fields.
Guidelines for Data Citation
Data citation is an essential practice in the field of research and academia. It allows researchers to properly credit and acknowledge the sources of data they have used in their work. Here are some guidelines to follow when citing data:
1. Provide a descriptive title: When citing a dataset, it is important to provide a clear and concise title that accurately reflects the content of the data. This helps other researchers easily identify and locate the dataset.
2. Include the author or creator: Just like citing a scholarly article or book, it is crucial to attribute the data to its author or creator. This provides proper recognition to the individual or organization responsible for collecting or generating the dataset.
3. Include the publication or release date: When citing a dataset, it is important to include the date when the data was published or released. This helps establish the credibility and currency of the data.
4. Provide a version or edition number: If multiple versions or editions of the dataset exist, it is advisable to include the specific version or edition number in the citation. This helps ensure accuracy and allows other researchers to access the exact version used in the study.
5. Include the data repository or source: Citing the data repository or source where the dataset is located is crucial for facilitating access to the data. It enables other researchers to locate and verify the dataset used in the study.
6. Use a persistent identifier: When citing data, it is recommended to use a persistent identifier, such as a DOI (Digital Object Identifier) or a URL. These identifiers ensure that the dataset remains accessible even if the location or URL changes over time.
7. Provide a direct link to the dataset: Including a direct link to the dataset in the citation allows other researchers to easily access and retrieve the data. This enhances transparency and reproducibility in research.
8. Follow a recognized data citation style: There are various data citation styles available, such as the DataCite Metadata Schema, the DataCite Citation Style, or the Digital Curation Centre (DCC) Recommended Data Citation Metadata. It is important to follow a recognized style to ensure consistency and standardization.
9. Include any necessary additional information: Depending on the specific dataset, there may be additional information that needs to be included in the citation, such as the geographic location of the data collection, the methodology used, or any licensing or usage restrictions. Including such details provides comprehensive information to other researchers.
10. Verify the accuracy of the citation: Before finalizing the citation, it is important to double-check and verify the accuracy of the information provided. This includes ensuring that all the necessary elements are included and that the citation follows the chosen data citation style.
By following these guidelines, researchers can ensure that they cite data correctly and ethically, giving proper credit to the sources of their data and contributing to the integrity and transparency of the scientific community.
Examples of Data Citation Styles
Data citation styles provide a standardized format for citing different types of data sources. Here are some examples of commonly used data citation styles:
- APA Style: The American Psychological Association (APA) style is widely used in the social sciences. When citing data in APA style, include the author’s name, publication date, title of the dataset, the version (if applicable), and the URL or DOI (Digital Object Identifier) if available.
- MLA Style: The Modern Language Association (MLA) style is commonly used in the humanities. In MLA style, include the author’s name, title of the dataset, version (if applicable), publishing organization or repository, publication date, and the URL or DOI.
- Chicago Style: The Chicago Manual of Style is a comprehensive style guide used in many disciplines. When citing data in Chicago style, include the author’s name, title of the dataset, version (if applicable), publishing organization or repository, publication date, and the URL or DOI.
- Harvard Style: The Harvard referencing style is popular in various academic disciplines. In Harvard style, cite data by including the author’s name, year of publication, title of the dataset, version (if applicable), publishing organization or repository, and the URL or DOI.
- Vancouver Style: The Vancouver style is commonly used in the medical and scientific fields. When citing data in Vancouver style, include the author’s name, title of the dataset, version (if applicable), publishing organization or repository, publication date, and the URL or DOI.
It’s important to note that different disciplines and publishers may have specific guidelines for data citation. Always consult the relevant style guide or instructions provided by the publisher when citing data.
Remember to prioritize consistency, accuracy, and completeness when citing data sources. This helps ensure that others can locate and verify the data you have used in your research.
Conclusion
Citing data is a crucial aspect of research and academic writing, as it ensures transparency, credibility, and integrity in scholarly work. By properly citing data, researchers provide a means for others to verify and build upon their findings.
Throughout this guide, we have explored different methods and formats for citing data, including the use of in-text citations and reference lists. We have also discussed the importance of providing comprehensive and accurate information about the data source.
Remember that each citation style may have specific guidelines for citing data, so it is essential to consult the respective style guide or consult with your instructor or librarian for guidance.
By following the principles of proper data citation, you contribute to the advancement of knowledge and the fostering of a scholarly community that values transparency, reproducibility, and accuracy in research.
FAQs
-
What is data citation?
Data citation is the practice of providing a bibliographic reference to data sets or individual data files. It allows researchers to properly acknowledge and cite the data they have used in their research, similar to how they would cite an article or a book. -
Why is data citation important?
Data citation is important for several reasons. Firstly, it acknowledges the efforts of data creators and encourages them to continue making data available for others to use. Additionally, it helps researchers build upon and validate previous work, promotes transparency and reproducibility, and facilitates collaboration within the scientific community. -
How should I format a data citation?
The format of a data citation depends on the citation style you are using. Generally, a data citation should include the author or creator, the title of the dataset, the publication or release date, the version number (if applicable), and a persistent identifier such as a DOI (Digital Object Identifier) or a URL. Make sure to consult the specific guidelines of your citation style for detailed formatting instructions. -
Where can I find the necessary information to cite a dataset?
The necessary information to cite a dataset can usually be found in the dataset’s metadata. Metadata is structured information that describes the dataset, including details about its creation, contents, and usage. It is often provided along with the dataset or can be accessed through data repositories or publishers’ websites. Look for information such as authors, dataset titles, publication dates, versions, and persistent identifiers. -
Are there any tools or resources available to help with data citation?
Yes, there are several tools and resources available to assist with data citation. Some popular options include citation management software like Zotero or Mendeley, which can help you organize and format your citations. Additionally, data repositories and publishers often provide guidance on how to properly cite datasets within their specific platforms. It’s always a good idea to consult the documentation and resources provided by the repository or publisher where you accessed the dataset.