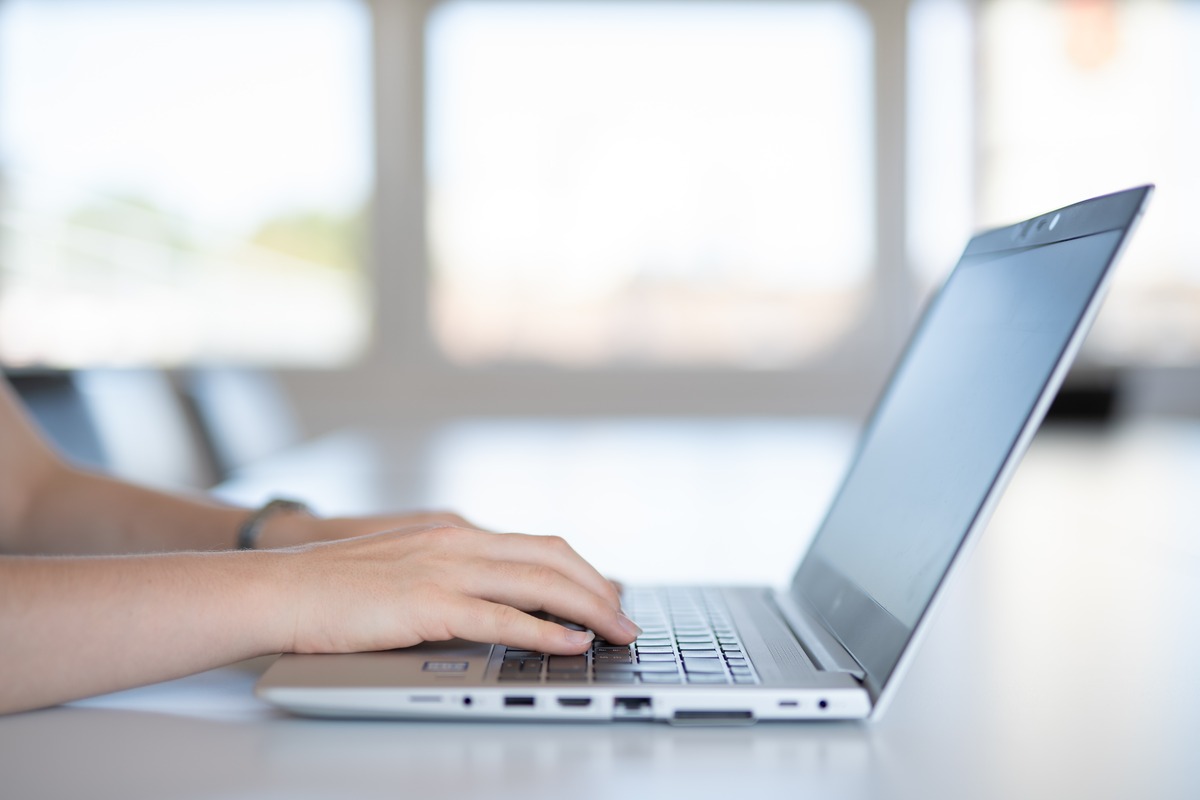
When it comes to analyzing data, one key aspect that often arises is the need to assign weights to different data points. Weighting data allows you to give more importance or significance to certain observations, thereby influencing the overall analysis and conclusions drawn from the data. Understanding how to weight data correctly is crucial to ensure accurate results and make informed decisions.
In this article, we will delve into the concept of weighting data and explore different methods and techniques that can be used to effectively assign weights to data points. Whether you are working with financial data, conducting market research, or analyzing scientific data, mastering the art of data weighting will undoubtedly enhance the quality of your analysis and help you derive meaningful insights. So, let’s dive in and discover the strategies behind weighting data!
Inside This Article
- Overview of Weighting Data
- Importance of Weighting Data
- Different Methods of Weighting Data
- Considerations and Challenges in Weighting Data
- Conclusion
- FAQs
Overview of Weighting Data
In the world of data analysis, weighting data is a crucial technique used to adjust the influence of different data points based on their relative importance. It involves assigning weights or factors to each data point based on certain criteria. These weights are then used to calculate aggregate statistics or make predictions.
Weighting data is commonly employed in various fields, including market research, social sciences, and opinion polling. It allows researchers to compensate for sample biases and ensure that their analysis accurately represents the population under study.
At its core, weighting data is about creating a representative sample. In some cases, certain segments of the population may be overrepresented or underrepresented in a dataset. Weighting helps correct these imbalances by giving more weight to data points from underrepresented groups and less weight to data points from overrepresented groups.
There are different methods of weighting data, and the choice of method depends on the specific objectives and characteristics of the dataset. Common methods include post-stratification, raking, and propensity score weighting.
In post-stratification, weights are assigned based on known population totals for specific characteristics, such as age, gender, or region. This ensures that the sample matches the population distribution for these variables. Raking, on the other hand, involves iteratively adjusting the weights until the sample distribution matches the population distribution for multiple variables simultaneously.
Propensity score weighting is often used in observational studies to account for the likelihood of selection bias. It involves estimating the probability of a particular data point being in the sample based on observed characteristics, and assigning weights accordingly.
While weighting data can improve the accuracy and representativeness of analysis, there are also considerations and challenges involved. It is essential to carefully determine the appropriate weighting factors and ensure that they are based on valid and reliable criteria. Additionally, weighting can increase the complexity of data analysis and interpretation.
Weighting data is a valuable tool for researchers and analysts seeking to draw meaningful insights from their datasets. By accounting for sample biases and ensuring representativeness, weighting data enhances the validity and reliability of statistical analysis.
Importance of Weighting Data
When conducting data analysis, the process of weighting data is of utmost importance. It involves assigning different weights to different data points or individuals in a dataset to reflect their relative importance or representativeness within a larger population. Weighting data allows researchers to account for biases or imbalances that may exist in the dataset and ensure that the findings accurately represent the target population.
There are several reasons why weighting data is crucial in research and analysis:
- Representativeness: Weighting data helps achieve greater representativeness by accounting for underrepresented or overrepresented groups within a sample. For instance, if a survey has a higher proportion of males than females, weighting the data can adjust for the discrepancy, ensuring that the results are more reflective of the actual population distribution.
- Equalizing Response Rates: In some cases, certain subgroups may have higher or lower response rates in surveys or studies. Weighting data allows for the adjustment of response rates, ensuring that the findings do not disproportionately favor or disadvantage any particular group.
- Correcting Bias: Weighting data can help correct for biases that may exist due to non-random sampling or non-response. By assigning appropriate weights to the data, researchers can minimize the impact of these biases and obtain more accurate results.
- Combining Multiple Data Sources: When combining data from different sources, such as surveys or administrative records, weighting data ensures that each source is appropriately represented and contributes proportionately to the overall analysis. This helps maintain the integrity and validity of the combined dataset.
- Statistical Analysis: Weighting data is essential for accurate statistical analysis. The weights assigned to each data point enable the calculation of reliable estimates, standard errors, and confidence intervals. Without proper weighting, the results of statistical analysis may be biased or misleading.
Overall, weighting data plays a crucial role in ensuring the accuracy, reliability, and validity of research findings. By accounting for various factors and adjusting for potential biases, researchers can obtain more representative and meaningful insights from their data. It is important to carefully consider the weighting methodology to ensure that it aligns with the research objectives and accurately reflects the population of interest.
Different Methods of Weighting Data
Weighting data is an essential process in statistical analysis, allowing us to account for the varying importance or representativeness of different observations within a dataset. There are several commonly used methods for weighting data, each suited for different situations and objectives. Let’s explore some of these methods:
1. Equal Weighting: This method assigns equal weight to each observation in the dataset. It assumes that every data point is equally important and representative. While this approach is simple and easy to implement, it may not accurately reflect the true characteristics of the population.
2. Probability Weighting: In this method, each observation is assigned a weight based on the probability of its occurrence in the population. This approach is commonly used when the sample is not representative of the population, and the weights help adjust for the biases introduced by the sampling process.
3. Inverse Weighting: Inverse weighting assigns higher weights to observations that are less frequent in the dataset. This method is useful when rare events or outliers need to be given more importance. For example, if studying customer satisfaction, weighting dissatisfied customers more heavily can provide a more accurate representation of the overall sentiment.
4. Post-stratification: Post-stratification involves dividing the population into subgroups or strata and assigning weights to observations based on the characteristics of these subgroups. This method is useful when the sample size within each stratum is small and does not accurately represent the population distribution.
5. Calibration Weighting: Calibration weighting is often used in survey research to adjust the sample weights to match known population totals for specific demographics or variables. This method ensures that the sample is representative and reduces potential biases introduced during the sampling process.
6. Propensity Score Weighting: This method is commonly used in observational studies and matching techniques. It assigns weights to observations based on their propensity scores, which measure the likelihood of being assigned to a particular group or treatment. This helps account for confounding variables and ensures that the weighted sample is more comparable and representative.
Each of these methods has its strengths and limitations, and the choice of weighting method depends on the specific research objectives and the characteristics of the dataset. It is essential to carefully consider the assumptions and requirements of each method before applying it to your data analysis.
Considerations and Challenges in Weighting Data
While weighting data can be an effective way to account for biases and inaccuracies in research or survey results, it is not without its considerations and challenges. Here are some key points to keep in mind when undertaking data weighting:
1. Sample Representativeness: One of the primary considerations is ensuring that the weighted data accurately represents the target population. It is essential to have a clear understanding of the characteristics of the population being studied and how well the sample used in the research aligns with those characteristics. If the sample is not representative, weighting may not yield accurate results.
2. Variable Selection: Another challenge in weighting data is determining which variables should be considered in the weighting process. It is important to select variables that are relevant to the research objective and have a significant impact on the distribution of the population. Choosing the wrong variables or omitting crucial ones can result in biased results.
3. Weighting Methodology: There are various methods for weighting data, such as post-stratification weighting, raking, or propensity score weighting. Each method has its own strengths and weaknesses, and selecting the appropriate methodology depends on the specific research context and data characteristics. It is crucial to understand the pros and cons of different weighting methods to ensure accurate results.
4. Data Quality and Missing Values: The quality of the data used for weighting is paramount. Inaccurate or unreliable data can lead to biased results, even if the weighting process itself is robust. Additionally, missing values can pose a challenge, as they need to be appropriately handled during the weighting process. Imputation techniques or other strategies may be necessary to address missing data and maintain the integrity of the weighted dataset.
5. Interpretation and Communication: It is essential to consider the interpretation and communication of weighted data. Weighted results might differ from unweighted results, and understanding these differences is crucial when communicating findings to stakeholders or making informed decisions based on the research. Clear and transparent reporting of the weighting process and its implications can help ensure that others correctly interpret the study findings.
6. Statistical Assumptions: Weighting data is based on certain statistical assumptions, such as linearity, homoscedasticity, and independence. These assumptions need to be carefully evaluated to ensure that they hold true for the data being weighted. Violations of these assumptions can undermine the validity of the weighting process and the resulting analysis.
7. Sensitivity Analysis: Performing sensitivity analysis is crucial when weighting data. It involves testing the robustness of the weighted results by examining the impact of different weighting procedures or variable selections on the overall findings. Sensitivity analysis helps determine the stability and reliability of the weighted data and provides insights into the potential effects of different weighting decisions.
Overall, while weighting data can address biases and improve the accuracy of research findings, it is essential to consider these challenges and limitations. A thoughtful and well-executed weighting process, combined with a thorough understanding of the data and its context, can lead to more reliable and meaningful results.
Conclusion
In conclusion, understanding how to weight data is essential in many fields, from statistics to machine learning and beyond. By assigning appropriate weights to different data points, we can improve the accuracy and reliability of our analyses and predictions.
Weighting data allows us to account for the significance or importance of different observations or variables in our analysis. Whether it be giving more weight to recent data or focusing on specific categories within a dataset, weighting helps us uncover patterns and make more informed decisions.
By using techniques such as inverse weighting, normalization, or using expert opinions, we can ensure that our data analysis is robust and unbiased. Weighting data also helps us deal with issues like imbalanced datasets and outliers, leading to more accurate results and insights.
So, if you want to make the most out of your data, remember the importance of weighting. It can unlock hidden insights, enhance the accuracy of your analysis, and ultimately empower you to make informed decisions based on reliable information.
FAQs
1. Why is data weight important?
Data weight is important because it allows you to assign relative importance or significance to different data points. By assigning weights to data, you can prioritize certain factors over others when making decisions or analyzing information. This helps to ensure that the most relevant and impactful data is given the appropriate level of attention and consideration.
2. How can I determine the weight of my data?
Determining the weight of your data depends on the specific context and objectives of your analysis. There are several approaches you can take, such as assigning weights based on expert judgment, using statistical techniques like regression analysis, or leveraging machine learning algorithms to automatically assign weights based on patterns in the data. The method you choose will depend on the complexity of your data and the level of accuracy and precision required.
3. What factors should I consider when deciding data weights?
When deciding data weights, it’s important to consider the relevance, reliability, and impact of each data point. Relevance refers to how directly the data relates to your objectives or research question. Reliability pertains to the quality and accuracy of the data source. Impact refers to the potential influence or significance a data point has on your analysis or decision-making process. By evaluating these factors, you can make informed decisions about how to assign weights to your data.
4. Can I change data weights over time?
Yes, you can change data weights over time. As new information becomes available or your objectives shift, it may be necessary to reassess the relevance and impact of certain data points. Changes in the external environment or emerging trends can also impact the relative importance of different factors. It’s important to regularly review and update data weights to ensure they remain aligned with your evolving needs and objectives.
5. Are there any tools or software available to help with data weighting?
Yes, there are tools and software available to assist with data weighting. These tools can range from simple spreadsheets or calculators that allow you to manually assign weights, to more advanced statistical software packages or machine learning algorithms. Some popular data analysis software, like Excel or R, provide functions for calculating weights based on different methodologies. Additionally, there are specialized software packages designed specifically for data weighting and analysis that offer more advanced features and capabilities.