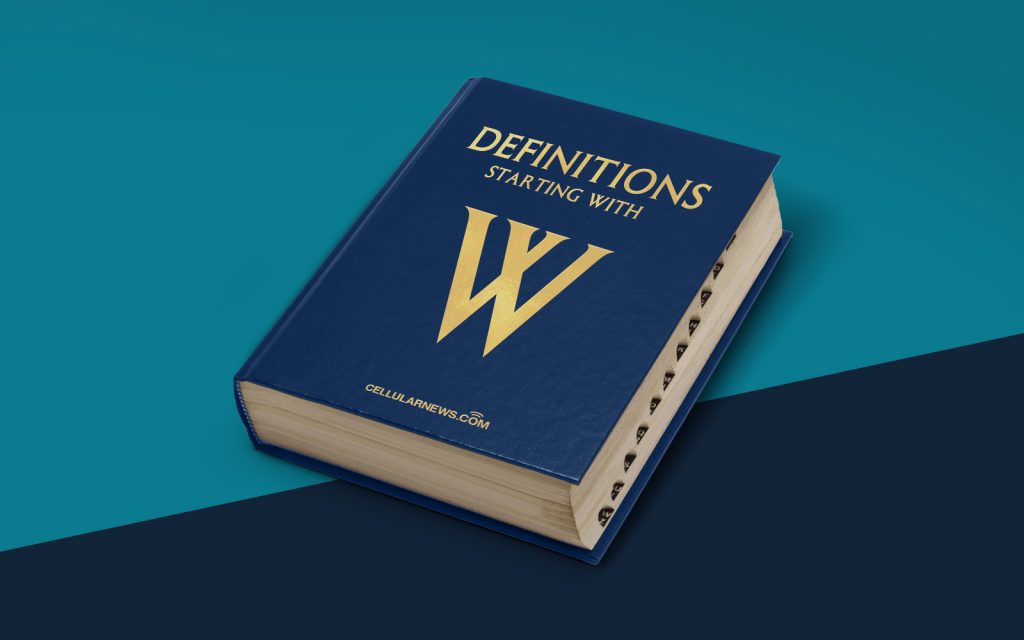
Introducing the Wasserstein GAN (WGAN): A Marvel of Generative Adversarial Networks
GANs, or Generative Adversarial Networks, have taken the field of deep learning by storm. These powerful algorithms have revolutionized the way we generate realistic images, videos, and even text. In this blog post, we will explore one particular category of GANs called “Wasserstein GANs” or WGANs.
So, what exactly is a Wasserstein GAN? Let’s dive in and find out!
The Definition of Wasserstein GAN
Wasserstein GAN is a variant of the traditional GAN that incorporates the Wasserstein distance metric to enhance the stability and performance of the network. It was introduced by Martin Arjovsky, Soumith Chintala, and Léon Bottou in 2017, and has since gained significant attention in the machine learning community.
A WGAN consists of two key components: the generator and the discriminator. The generator is responsible for creating new samples that resemble a particular dataset, such as images of faces or handwritten digits. On the other hand, the discriminator’s role is to differentiate between real and fake samples.
Key Takeaways
- Wasserstein GAN (WGAN) is a variant of Generative Adversarial Networks (GANs) that incorporates the Wasserstein distance metric.
- WGANs offer improved stability and performance compared to traditional GANs.
The Advantages of Wasserstein GAN
Wasserstein GANs offer several advantages over traditional GANs, making them a popular choice in the field of generative modeling. Here are a few notable benefits:
- Improved Training Stability: One of the main issues with traditional GANs is the instability during training. WGANs address this problem by using the Wasserstein distance, which provides a smoother and more meaningful measure of distance between probability distributions. This leads to more stable training dynamics and mitigates problems like mode collapsing.
- Better Gradient Estimation: Estimating gradients accurately is crucial for training deep learning models. WGANs employ a technique called “gradient penalty” to ensure more reliable gradient estimation. This approach helps overcome the problem of vanishing gradients, allowing the network to learn more effectively.
- Enhanced Output Quality: WGANs are known to produce high-quality samples with better image sharpness and clearer object definition. This improvement is attributed to the stable training process and the utilization of Wasserstein distance, which encourages the generator to generate meaningful samples that are more consistent with the real data.
In Conclusion
The Wasserstein GAN (WGAN) is a breakthrough in the field of generative modeling, offering improved stability, better gradient estimation, and higher output quality compared to traditional GANs. Its utilization of the Wasserstein distance metric has paved the way for more reliable and effective training of generative models.
As the field of deep learning continues to evolve, WGANs prove to be a valuable tool for various tasks, including image generation, text synthesis, and data augmentation. By harnessing the power of WGANs, researchers and practitioners can push the boundaries of artificial intelligence, creating new and exciting possibilities for the future.