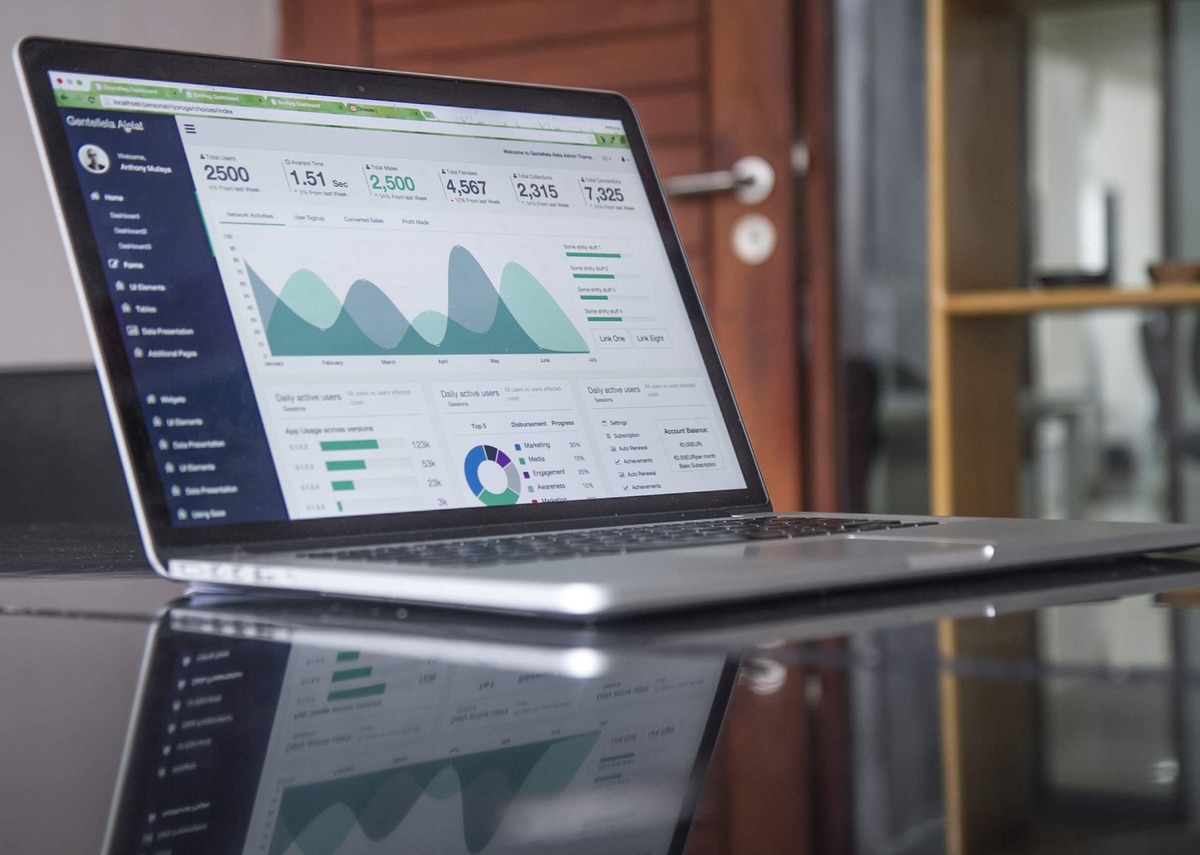
Are you struggling to aggregate data from multiple sources and make sense of it all? Look no further! In this article, we will walk you through the ins and outs of data aggregation, helping you understand how to bring together data from diverse sources into a single, comprehensive view.
Data aggregation is the process of collecting and combining data from various sources to gain insights and make informed decisions. Whether you are a business owner, a data analyst, or a researcher, effective data aggregation is crucial for extracting meaningful information.
Throughout this article, we will explain the importance of data aggregation, discuss different techniques and tools to aggregate data, and provide you with practical tips to maximize the efficiency and accuracy of your aggregation process. So let’s dive in and discover how to aggregate data like a pro!
Inside This Article
- Overview
- Step 1: Identifying Data Sources
- Step 2: Data Collection and Extraction
- Step 3: Data Cleansing and Validation
- Step 4: Data Integration and Transformation
- Step 5: Data Storage and Organization
- Step 6: Data Analysis and Interpretation
- Step 7: Data Visualization and Reporting
- Tips for Successful Data Aggregation
- Common Challenges in Data Aggregation
- Best Practices for Data Aggregation
- Tools and Software for Data Aggregation
- Case Studies: Successful Data Aggregation Examples
- Conclusion
- FAQs
Overview
Data aggregation, a fundamental process in data analysis and management, involves the collection, organization, and analysis of various sources of data to derive meaningful insights. It is the process of combining data from different sources or systems to create a unified view for analysis and reporting purposes.
In today’s data-driven world, organizations across industries are facing the challenge of managing large volumes of data coming from diverse sources such as databases, spreadsheets, social media, and IoT devices. Aggregating this data allows businesses to gain a holistic understanding of their operations, customers, and market trends, enabling them to make informed decisions and drive growth.
Aggregating data involves a series of steps, including identifying data sources, collecting and extracting data, cleansing and validating the data, integrating and transforming it, storing and organizing it, and finally analyzing and interpreting the data to derive insights.
By aggregating data, organizations can uncover hidden patterns, identify correlations, and make data-driven decisions. It helps in spotting trends, understanding customer behavior, optimizing processes, and improving overall business performance.
However, data aggregation can be a complex task, requiring a combination of technical expertise, data management skills, and the right tools. It is important to follow best practices, overcome challenges, and utilize appropriate software and tools to ensure the accuracy and reliability of the aggregated data.
In this article, we will delve into the steps involved in data aggregation, discuss common challenges and best practices, and explore some tools and case studies to illustrate successful data aggregation examples.
Step 1: Identifying Data Sources
When it comes to data aggregation, the first and most crucial step is to identify the data sources. Before you can begin collecting and analyzing data, you need to determine where the data is coming from and what specific sources you will be working with.
Identifying data sources can vary depending on the purpose of your data aggregation project. It could involve gathering data from various internal systems within your organization or external sources such as API feeds, public databases, or even web scraping.
To begin the process of identifying data sources, start by considering the specific goals and objectives of your project. What kind of data do you need? What questions are you trying to answer? Understanding your end goals will help you narrow down the relevant data sources.
Next, conduct thorough research to explore potential data sources that align with your project requirements. This can involve consulting with subject matter experts, exploring industry-specific databases, or even reaching out to relevant stakeholders to inquire about available data.
During this step, it is important to keep in mind the quality and reliability of the data sources. Ensure that the data you are collecting is accurate, up-to-date, and relevant to your project’s objectives. Scrutinize the credibility of the source and verify the data’s integrity to minimize potential errors or biases.
Consider the scalability of the data sources as well. As your project progresses, will you be able to access an adequate amount of data to generate meaningful insights? It is essential to assess whether the chosen sources can provide the volume and variety of data necessary for your analysis.
Finally, document and organize the identified data sources for easy reference and future use. Create a data source inventory that includes details such as the source name, description, access method, data format, and any relevant terms or conditions associated with data usage.
By diligently identifying and documenting your data sources, you set a solid foundation for your data aggregation project. This step ensures that you have a clear understanding of where your data is coming from and establishes the groundwork for the subsequent steps of the aggregation process.
Step 2: Data Collection and Extraction
Once you have identified the data sources that you need for your aggregation process, the next step is to collect and extract the data. This step is crucial as it lays the foundation for the accuracy and integrity of the aggregated data. Here are some key considerations to keep in mind during the data collection and extraction process:
1. Determine the Data Collection Method: Depending on the nature of the data you are collecting, you may use various methods such as web scraping, API calls, data feeds, or manual data entry. Each method has its own advantages and limitations, so choose the one that best suits your needs.
2. Ensure Data Quality: It is essential to validate and verify the collected data to ensure its authenticity and accuracy. Perform data cleansing techniques like removing duplicates, correcting errors, and ensuring consistency in formats and values. This step is crucial to avoid biased or flawed aggregated data.
3. Establish Data Extraction Schedule: Determine how frequently you will collect and update the data. Depending on the data source and its update frequency, you may need to set up automated data extraction processes to keep your aggregated data up to date. Consider scheduling daily, weekly, or monthly extractions, depending on your requirements.
4. Use Data Extraction Tools: There are various tools and software available that can assist in automating the data extraction process. These tools can help extract data from websites, APIs, databases, or other sources efficiently and accurately. Research and choose the tools that align with your data extraction needs.
5. Ensure Data Security and Privacy: When collecting data, it is important to ensure its security and protect the privacy of individuals or organizations whose data you are aggregating. Adhere to privacy regulations and implement secure data handling practices to maintain data integrity and safeguard sensitive information.
By following these steps, you can effectively collect and extract data for your aggregation process. This will lay the foundation for accurate and reliable aggregated data that can be utilized for further analysis and decision-making.
Step 3: Data Cleansing and Validation
Once you have identified and collected the data from various sources, the next crucial step in the data aggregation process is data cleansing and validation. This step involves cleaning up the collected data to ensure its accuracy, consistency, and reliability.
Data cleansing involves the identification and correction of any errors, inconsistencies, or inaccuracies in the data. This can include removing duplicate entries, correcting misspellings, standardizing data formats, and resolving any missing or incomplete data.
Data validation, on the other hand, ensures that the collected data meets specific criteria or business rules. This process involves checking the data for completeness, correctness, and adherence to predefined rules. It helps in identifying any outliers or anomalies that may affect the quality of the aggregated data.
During the data cleansing and validation phase, you can use various techniques and tools to ensure the accuracy of the data. These may include automated data cleansing software, data profiling, data matching, and data profiling algorithms.
One commonly used technique is outlier detection, which helps in identifying and handling data points that deviate significantly from the expected values. By identifying and addressing outliers, you can improve the overall quality and reliability of the aggregated data.
Another important aspect of data cleansing and validation is data normalization. This process involves transforming the collected data into a consistent format, eliminating any redundancies, and ensuring data integrity. Normalization helps in enhancing data quality and enables efficient analysis and interpretation.
Additionally, it is crucial to involve domain experts or subject matter experts during the data cleansing and validation process. Their expertise and knowledge can help in identifying any errors or inconsistencies in the data and provide valuable insights to ensure accurate and reliable aggregation.
Remember that data cleansing and validation is an ongoing process. As new data is collected and added to the aggregation, it is essential to continuously monitor and validate the data to maintain its quality and integrity.
By following a comprehensive data cleansing and validation process, you can ensure that the aggregated data is accurate, consistent, and reliable, setting a strong foundation for further analysis and decision-making.
Step 4: Data Integration and Transformation
Once you have collected and cleansed the data, the next step in the data aggregation process is data integration and transformation. This step involves combining data from multiple sources and converting it into a unified format for further analysis and interpretation.
Data integration is the process of merging data from different sources into a single, coherent dataset. It involves identifying common data elements and linking them together based on unique identifiers or key fields. This ensures that data from diverse sources can be combined to provide a comprehensive view of the information.
In addition to integrating the data, it is also important to transform it into a standardized format. This includes converting data types, normalizing values, and resolving any inconsistencies or discrepancies. Transformation ensures that the data is uniform and can be effectively analyzed without any biases or errors.
During the integration and transformation process, it is common to encounter data quality issues or inconsistencies. These could include missing values, duplicate records, or conflicting data formats. It is crucial to address these issues through data cleansing techniques, such as data deduplication, data validation, and data imputation.
Data integration and transformation can be achieved using various tools and techniques. This may involve using ETL (Extract, Transform, Load) processes, data integration platforms, or custom scripts. The choice of method depends on the complexity and volume of the data, as well as the specific requirements of the analysis.
Once the data has been integrated and transformed, it is ready for further analysis and interpretation. This can include performing statistical analysis, generating insights, and uncovering patterns or trends. The transformed data can also be used for reporting, visualization, or feeding into machine learning algorithms for predictive modeling.
Step 5: Data Storage and Organization
Once you have collected and cleansed your data, the next crucial step in the data aggregation process is storing and organizing the data. Effective data storage and organization are essential to ensure easy accessibility, efficient retrieval, and accurate analysis of the aggregated data.
Here are some key considerations for data storage and organization:
- Choose the Right Storage Solution: Selecting the appropriate storage solution is vital to accommodate the size and type of data you are aggregating. Cloud-based storage options, such as Amazon S3, Google Cloud Storage, or Microsoft Azure, provide scalable and cost-effective solutions for storing large volumes of data.
- Design a Logical Data Structure: Establishing a logical and well-defined data structure is crucial for efficient data organization. This involves designing a schema that accurately represents the relationships between different data elements and entities. Relational databases, document-oriented databases, or data warehouses can be utilized based on the nature of the data and analysis requirements.
- Implement Data Security Measures: Data security is of utmost importance when storing and organizing aggregated data. Implementing appropriate access controls, encryption, and backup mechanisms ensures the confidentiality, integrity, and availability of the aggregated data. Regular audits and monitoring can help identify any potential security breaches or vulnerabilities.
- Establish Data Governance Policies: Data governance policies define the rules and guidelines for managing and maintaining the aggregated data. This includes establishing data ownership, defining data retention periods, and ensuring compliance with data privacy regulations and industry standards. Clear data governance policies facilitate consistent and reliable data storage and organization.
- Implement Metadata Management: Metadata plays a crucial role in categorizing and organizing aggregated data. By capturing and managing metadata, such as data source, data type, data quality, and data lineage, you can enhance the discoverability and usability of the aggregated data. Metadata management tools and solutions can automate the process of capturing and maintaining metadata.
- Consider Scalability and Performance: As your data grows, it is important to plan for scalability and performance. Ensure that the storage solution and data organization approach can handle increasing data volumes and processing requirements. Distributed database systems or data lakes can be explored to effectively handle large-scale data aggregation.
- Enable Data Backup and Recovery: Implementing regular data backup and recovery mechanisms is crucial to ensure data resilience. In case of any data loss or system failure, having reliable backup copies of the aggregated data allows for easy recovery and minimizes the risk of data loss or disruption. Automated backup schedules and redundant storage systems help protect the aggregated data.
By following these best practices for data storage and organization, you can lay a strong foundation for successful data aggregation. It will enable you to efficiently store and access the aggregated data, facilitating seamless data analysis and interpretation.
Step 6: Data Analysis and Interpretation
Data analysis and interpretation is a crucial step in the process of data aggregation. Once you have collected and cleansed your data, it’s time to delve into the insights and meaning hidden within the numbers. This step involves applying statistical methods, data modeling techniques, and analytical tools to derive valuable insights and make informed decisions based on the aggregated data.
Here are some key aspects to consider during the data analysis and interpretation stage:
- Identify Key Performance Indicators (KPIs): Before diving into the analysis, it’s important to define the KPIs that align with your specific goals. These indicators will help you measure the success or effectiveness of your data aggregation efforts.
- Data Exploration: Take the time to explore and understand your data. This can involve examining patterns, trends, and relationships between variables. Visualization tools, such as charts and graphs, can be immensely helpful in gaining a deeper understanding of the data.
- Statistical Analysis: Apply statistical techniques to analyze the data and uncover meaningful insights. This can include measures of central tendency, variability, correlation, regression analysis, and hypothesis testing. Statistical software packages like R or Python can assist in conducting these analyses.
- Data Modeling: Use data modeling techniques to create predictive or descriptive models that can provide further insights into the data. These models can help identify patterns, forecast future trends, and make data-driven predictions or decisions.
- Interpretation and Insights: Once the analysis is complete, it’s time to interpret the findings and extract actionable insights. This involves recognizing patterns, anomalies, trends, and any other relevant information that can contribute to decision-making or problem-solving.
- Communicate the Results: Presenting the findings in a clear and concise manner is crucial for effective data interpretation. Use data visualization techniques, such as charts, graphs, and dashboards, to communicate the insights to stakeholders, enabling them to understand and act upon the aggregated data.
Remember, data analysis and interpretation are iterative processes. It’s important to continuously refine and adjust your analysis as you dive deeper into the data and discover new insights. By effectively analyzing and interpreting the aggregated data, you can unlock valuable knowledge that can lead to improved decision-making, optimized processes, and ultimately, better outcomes.
Step 7: Data Visualization and Reporting
Data visualization and reporting are crucial components of the data aggregation process. Once you have collected, cleansed, integrated, and stored your data, it’s essential to present it in a meaningful and easily understandable way. Data visualization allows you to transform complex datasets into visual representations such as charts, graphs, and maps, making it easier for stakeholders to identify patterns, trends, and insights.
There are various techniques and tools available for data visualization, ranging from basic spreadsheets and presentation software to specialized data visualization software. The choice of tool will depend on the complexity of your data and the level of interactivity and customization you require.
When creating visualizations, it’s important to consider the target audience and the purpose of the report. Are you presenting to executives who need a high-level overview, or analysts who require detailed insights? Understanding your audience will help determine the level of granularity and the types of visualizations to include.
Effective data reporting goes beyond just creating charts and graphs. It involves interpreting the data, extracting meaningful insights, and presenting them in a compelling narrative. This requires clear and concise communication, highlighting the key findings, and providing actionable recommendations.
To enhance the effectiveness of your data visualizations and reports, consider the following tips:
- Keep it simple: Avoid cluttering your visualizations with unnecessary details or complex designs. Keep the focus on the data and the insights you want to convey.
- Use appropriate visualizations: Choose the right chart or graph type to best represent your data. Bar graphs, line charts, and pie charts are commonly used for different purposes.
- Add context: Provide contextual information such as labels, legends, and annotations to help the audience understand the meaning behind the data.
- Highlight key findings: Use colors, emphasis, or callouts to draw attention to the most important insights or trends in your data.
- Make it interactive: If possible, allow users to interact with the visualizations by filtering or drilling down into the data for deeper analysis.
Furthermore, it’s essential to regularly update and refresh your data visualizations and reports as new data becomes available. This ensures that your insights stay relevant and up-to-date, enabling decision-makers to make informed choices based on the latest information.
Tips for Successful Data Aggregation
When it comes to data aggregation, there are several key tips that can help ensure your process is successful and yields accurate and valuable insights. Here are some important considerations to keep in mind:
1. Define your objectives: Before you begin aggregating data, clearly define the objectives and goals you want to achieve. Whether it’s understanding customer behavior or analyzing market trends, having a clear purpose will guide your data collection and analysis.
2. Identify relevant data sources: Take the time to identify the data sources that are most relevant to your objectives. This could include internal databases, third-party APIs, public datasets, or even social media platforms. Choosing the right sources will ensure that you are collecting the most accurate and comprehensive data.
3. Ensure data quality: Data quality is crucial for meaningful analysis. Cleaning and validating the data before aggregation is essential to eliminate any errors or inconsistencies that could impact your results. Use data cleansing techniques and validation checks to ensure the accuracy and integrity of the collected data.
4. Use appropriate data integration methods: Data integration involves combining data from different sources into a unified format. Depending on the complexity of your data sources, you may need to employ different integration techniques such as ETL (Extract, Transform, Load) processes or API integrations. Choose the method that suits your needs and ensures seamless data integration.
5. Optimize data storage and organization: Efficient data storage and organization are critical for easy access and analysis. Choose a scalable and secure storage solution, such as cloud-based databases, that can handle large volumes of data. Create a logical and structured organization system to make data retrieval and analysis more efficient.
6. Analyze and interpret the data: Once the data is aggregated, it’s time to analyze and interpret the insights. Utilize data analytics tools and techniques to uncover patterns, correlations, and trends. This will help you gain actionable insights and make informed decisions based on the aggregated data.
7. Visualize and report your findings: Presenting your findings in a clear and visually appealing manner is crucial for effective communication. Use data visualization tools to create charts, graphs, and dashboards that effectively convey the insights derived from the aggregated data. Prepare comprehensive reports that highlight key findings and recommendations.
By following these tips and best practices, you can ensure a successful data aggregation process that leads to valuable insights and informed decision-making.
Common Challenges in Data Aggregation
Data aggregation is a complex process that involves collecting, cleaning, integrating, and analyzing data from various sources. While it offers many benefits, there are also common challenges that organizations face when aggregating data. Understanding these challenges can help you anticipate and address them effectively. Let’s explore some of the most common challenges in data aggregation:
1. Data Quality: One of the biggest challenges in data aggregation is ensuring the quality of the data. Incomplete, inaccurate, or inconsistent data can have a significant impact on the overall analysis and decision-making. It is crucial to have robust data validation and cleansing processes in place to minimize errors and ensure the reliability of the aggregated data.
2. Data Integration: Integrating data from different sources can be a complex task. Each data source may have its own format, structure, and naming conventions, making it challenging to merge and consolidate the data. Data integration solutions and tools can help streamline this process, but it requires careful planning and mapping of data elements across sources.
3. Data Security: Aggregated data may contain sensitive and confidential information, making data security a critical concern. Ensuring the privacy and protection of the aggregated data is essential to prevent unauthorized access or breaches. Implementing robust security measures, such as data encryption and access controls, is necessary to maintain data integrity and confidentiality.
4. Scalability: As the volume of data grows, scalability becomes a significant challenge in data aggregation. Processing and analyzing large datasets require robust infrastructure and computational resources. Organizations need to invest in scalable solutions that can handle increasing data volumes without compromising performance or causing delays in data aggregation processes.
5. Data Governance: Effective data governance is essential for successful data aggregation. Organizations need to establish clear data governance policies and procedures to ensure data accuracy, compliance with regulations, and ethical use of the aggregated data. Maintaining data consistency, data lineage, and addressing data ownership and accountability issues are key aspects of data governance in the context of data aggregation.
6. Data Timeliness: In some cases, the timeliness of data can be a challenge in data aggregation. Real-time data aggregation requires a robust infrastructure and efficient data collection processes. Delayed or outdated data can impact the accuracy and relevance of the analysis, especially in fast-paced industries where real-time insights are crucial.
7. Data Variety: Data aggregation often involves combining data from different sources, including structured and unstructured data, text, audio, video, and more. Managing and analyzing data of various formats and types can be a challenge, as different tools and techniques may be required to extract insights from different data formats. Organizations need to have flexible data processing capabilities to handle diverse data sources effectively.
By anticipating and addressing these common challenges, organizations can ensure the success and reliability of their data aggregation efforts. Implementing robust data quality measures, investing in scalable infrastructure, and adopting a comprehensive data governance framework are some of the key strategies to overcome these challenges in data aggregation.
Best Practices for Data Aggregation
Data aggregation plays a crucial role in deriving meaningful insights and making informed business decisions. To ensure accurate and reliable results, it is important to follow best practices throughout the data aggregation process. Here are some key practices to consider:
1. Define clear objectives: Before starting the data aggregation process, clearly define your objectives and identify the specific data requirements. This will help ensure that you collect and aggregate the right data to meet your goals.
2. Choose reliable data sources: Identify and select reliable, trustworthy data sources that provide accurate and up-to-date information. Consider factors such as data quality, credibility of the source, and relevance to your objectives.
3. Standardize data formats: To facilitate seamless data integration and analysis, it is important to standardize the formats of the data from various sources. This includes consistent date formats, unit measurements, and naming conventions.
4. Implement data quality checks: Data quality is paramount in data aggregation. Implement data cleansing and validation processes to ensure the accuracy, completeness, and consistency of the aggregated data. This includes identifying and rectifying any outliers, duplications, or missing values.
5. Regularly update data: Keep your aggregated data up to date by establishing a regular update schedule. This ensures that you are working with the most current and relevant information for analysis and decision-making.
6. Maintain data security: Data aggregation often involves handling sensitive and confidential information. Implement robust security protocols to protect the integrity and confidentiality of the aggregated data. This includes encryption, access controls, and regular data backups.
7. Document your processes: Maintain a comprehensive documentation of the data aggregation process, including the steps taken, data sources used, and any transformations or manipulations applied. This documentation ensures transparency, repeatability, and facilitates future analysis or audits.
8. Validate results: After aggregating the data, validate the results to ensure they align with your objectives and expectations. Cross-check the aggregated data against known benchmarks or perform statistical analyses to verify the accuracy and reliability of the results.
9. Continuously refine and optimize: As you gain insights from the aggregated data, seek opportunities to refine and optimize your data aggregation process. This includes incorporating feedback, exploring new data sources, and evaluating the effectiveness of your data aggregation methods.
By following these best practices for data aggregation, you can enhance the quality, reliability, and usefulness of the aggregated data, enabling you to make well-informed decisions and drive business success.
Tools and Software for Data Aggregation
When it comes to data aggregation, having the right tools and software is crucial to streamline the process and ensure accurate results. The market offers a wide range of options, each with its own set of features and capabilities. Let’s explore some of the most popular tools and software for data aggregation:
1. Python: Python, a versatile programming language, is widely used for data aggregation. Its extensive libraries, such as Pandas and NumPy, provide powerful data manipulation capabilities, making it an excellent choice for extracting, cleaning, and analyzing data.
2. R: R is another popular programming language for data aggregation and analysis. It offers advanced statistical capabilities, allowing users to perform complex data transformations and modeling. The tidyverse package in R provides a comprehensive suite of tools specifically designed for data wrangling and visualization.
3. SQL: Structured Query Language (SQL) is a standard language for managing relational databases. It enables users to query, manipulate, and aggregate data effectively. SQL is a widely adopted tool for data aggregation, especially in organizations with large datasets stored in relational databases.
4. Talend: Talend is an open-source data integration platform that offers a wide variety of features for data aggregation. It allows users to extract data from multiple sources, transform it, and load it into a centralized repository. Talend supports both batch and real-time data processing, making it suitable for various use cases.
5. Tableau: Tableau is a powerful data visualization tool that also provides data aggregation capabilities. Users can connect to multiple data sources, combine and blend data, and create interactive visualizations and dashboards. Tableau makes it easy to explore and analyze data from different angles.
6. Alteryx: Alteryx is a comprehensive platform that combines data integration, data preparation, and analytics. It allows users to aggregate and cleanse data from various sources before performing in-depth analysis. With its intuitive interface, Alteryx makes complex data aggregation tasks more accessible to non-technical users.
7. Apache Spark: Apache Spark is a fast and distributed computing framework that offers built-in data aggregation capabilities. It can process large-scale datasets in memory, enabling efficient and scalable data aggregation. Spark provides APIs in various programming languages, making it flexible and versatile.
8. Microsoft Excel: Excel, a widely used spreadsheet software, can also be utilized for simple data aggregation tasks. With its sorting, filtering, and formula functions, users can organize and summarize data easily. Excel is a great option for smaller datasets and quick analysis.
These are just a few examples of the numerous tools and software available for data aggregation. The best choice depends on the specific requirements, complexity of the data, and user expertise. It is recommended to evaluate the features, compatibility, and scalability of each tool before making a decision. Additionally, considering the budget and support options is essential for a successful data aggregation implementation.
Case Studies: Successful Data Aggregation Examples
Data aggregation is a crucial process for businesses seeking to gain valuable insights from multiple sources of information. Let’s explore some real-life case studies where successful data aggregation played a key role in driving business success.
1. Company XYZ: Improving Customer Experience
Company XYZ, a leading e-commerce retailer, wanted to enhance its customer experience by understanding customer preferences and behaviors. By aggregating data from various touchpoints such as website interactions, social media, and call center logs, they were able to create a comprehensive customer profile. This helped them personalize product recommendations, improve targeting of marketing campaigns, and provide tailored customer support, ultimately leading to increased customer satisfaction and loyalty.
2. HealthTech Startup: Optimizing Healthcare Services
A HealthTech startup aimed to optimize healthcare services by aggregating data from electronic health records, wearable devices, and real-time patient monitoring systems. By combining this data, they were able to analyze patient health trends, identify high-risk individuals, and create preventive care strategies. This led to improved patient outcomes, reduced healthcare costs, and greater efficiency in delivering personalized healthcare solutions.
3. Financial Institution: Fraud Detection
A financial institution faced a significant challenge with detecting and preventing fraudulent transactions. By aggregating data from various sources such as transaction logs, customer behavior patterns, and external fraud databases, they developed a robust fraud detection system. This allowed them to identify anomalies, patterns, and suspicious activities, enabling them to take immediate action and mitigate potential financial losses.
4. Transportation Company: Optimizing Route Efficiency
A transportation company aimed to optimize its route efficiency to reduce costs and improve delivery times. By aggregating data from GPS devices, traffic reports, and weather forecasts, they were able to identify the most efficient routes for their drivers. This resulted in reduced fuel consumption, minimized travel time, and improved overall customer satisfaction.
5. Retail Chain: Demand Forecasting
A retail chain wanted to improve their inventory management and demand forecasting capabilities. By aggregating data from their point-of-sale systems, online sales platforms, and external market data, they were able to accurately predict customer demand and optimize their inventory levels. This led to reduced stockouts, decreased waste, and improved profitability.
These case studies demonstrate the power of data aggregation in improving decision-making, enhancing customer experiences, optimizing operations, and driving business growth. By harnessing the potential of data aggregation, businesses can gain a competitive edge in today’s data-driven world.
Conclusion
Aggregating data is a powerful technique that allows us to gather and consolidate information from multiple sources into a single, comprehensive view. Whether you are a business looking to analyze sales data, a researcher gathering data for a study, or simply someone trying to gain insights from various sources, data aggregation can simplify and streamline your process.
By using the right tools and approaches, you can save time and effort in manually collecting and organizing data. With the ability to bring together data from different formats and locations, you can uncover trends, patterns, and correlations that may otherwise go unnoticed.
Remember to plan your data aggregation strategy carefully, considering factors such as data sources, frequency of updates, and the desired output format. Utilizing APIs, web scraping, or database queries can help automate the data collection process and ensure accuracy and consistency.
So, the next time you find yourself drowning in a sea of data, take advantage of data aggregation techniques to rise above the waves and gain clarity and valuable insights.
FAQs
1. What is data aggregation?
Data aggregation is the process of collecting and organizing large sets of data from various sources into a unified format. It involves combining and summarizing data to gain insights and make informed decisions.
2. Why is data aggregation important?
Data aggregation allows businesses and organizations to analyze and understand information from multiple sources, helping them identify patterns, trends, and correlations that may not be evident when analyzing individual datasets. It enables better decision-making, improved efficiency, and the ability to extract meaningful insights.
3. How is data aggregation performed?
Data aggregation can be performed using various methods and tools. Common techniques include manual data entry, data integration through APIs (Application Programming Interfaces), data extraction and transformation using ETL (Extract, Transform, Load) processes, and data warehousing.
4. What are the benefits of data aggregation?
By aggregating data, businesses can gain a comprehensive understanding of their operations, customer behavior, market trends, and more. Some benefits of data aggregation include improved data accuracy, time savings, enhanced reporting capabilities, better decision-making, and the ability to identify new opportunities.
5. What are some applications of data aggregation?
Data aggregation has diverse applications across various industries. It is commonly used in finance and banking for risk analysis, fraud detection, and portfolio management. In healthcare, data aggregation helps monitor patient populations, analyze clinical outcomes, and identify treatment patterns. E-commerce businesses use data aggregation to understand customer behavior, personalize recommendations, and optimize pricing strategies.