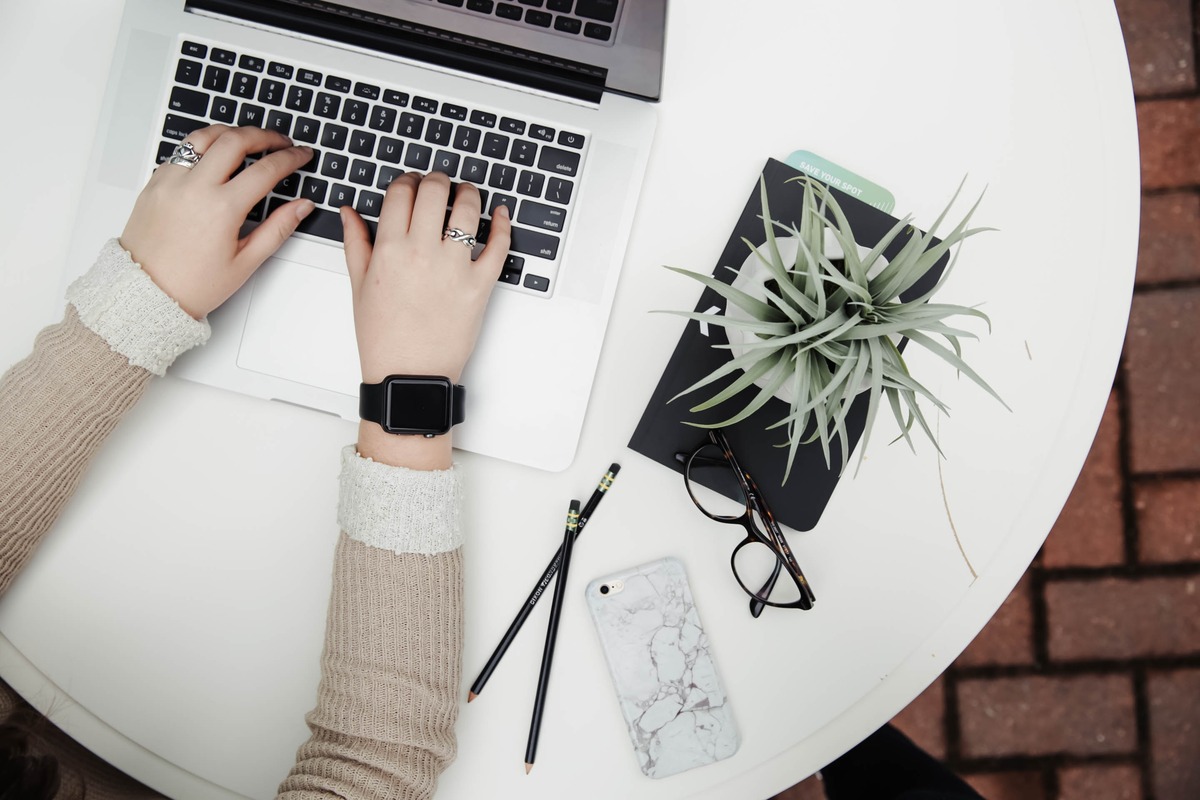
Privacy has become a major concern in today’s digital age, with the increasing amount of personal data being collected and stored by companies and organizations. De-identifying data has emerged as a crucial process to safeguard individual privacy while still allowing for the analysis and use of data for various purposes. By removing or obscuring personally identifiable information from datasets, de-identification aims to protect individuals’ identities and sensitive information.
In this article, we will delve into the concept of de-identifying data, exploring its importance and the methods commonly used for de-identification. Whether you’re a business owner, a data scientist, or simply someone interested in understanding how privacy is protected, this article will provide you with the guidance and insights you need to effectively de-identify data and ensure the privacy of individuals while still benefiting from the power of data analysis.
Inside This Article
- Overview of De-Identifying Data
- Benefits of De-Identifying Data
- Common Techniques for De-Identifying Data
- Legal and Ethical Considerations in Data De-Identification
- Conclusion
- FAQs
Overview of De-Identifying Data
Data de-identification is the process of removing or altering personal identifiers from a dataset, making it difficult to identify individuals. It is an essential step in ensuring privacy and protecting sensitive information when sharing or analyzing data. By de-identifying data, organizations can minimize the risk of unauthorized access or data breaches while still providing valuable insights.
The process of de-identifying data involves several techniques and considerations. It goes beyond simply removing names or other explicit identifiers and requires a thorough understanding of the data and potential disclosure risks. De-identification should be done in a way that minimizes the chances of re-identification while preserving the utility and value of the data being shared.
De-identifying data is particularly important in industries that handle sensitive information, such as healthcare, finance, and research. It helps organizations comply with data protection regulations while still allowing for data analysis and research purposes. By de-identifying data, organizations can strike a balance between privacy and data utility.
It is important to note that de-identifying data is not a foolproof method to guarantee anonymity. Advances in data science and technology make re-identification methods more sophisticated. Therefore, organizations must continually assess and update their de-identification techniques to stay ahead of potential risks.
The goal of de-identifying data is to make it difficult, if not impossible, to link the data back to specific individuals. This is typically achieved by removing or modifying personal identifiers such as names, addresses, social security numbers, or any other identifying information. However, it is crucial to ensure that other indirect identifiers or combinations of data cannot be used to identify individuals.
Overall, de-identifying data is a critical step in maintaining privacy and protecting sensitive information. It allows organizations to share or analyze data without compromising individuals’ privacy and addresses legal and ethical concerns surrounding data usage. By implementing appropriate de-identification techniques, organizations can mitigate privacy risks while still harnessing the power of data-driven insights.
Benefits of De-Identifying Data
De-identifying data is the process of removing or modifying personally identifiable information (PII) from datasets. This helps protect individual privacy while still allowing organizations to analyze and share data for various purposes. Here are some key benefits of de-identifying data:
1. Privacy Protection: De-identifying data ensures that sensitive personal information such as names, social security numbers, and addresses are removed or altered, reducing the risk of unauthorized access or misuse. It helps organizations comply with privacy regulations and builds trust with individuals whose data is being collected.
2. Enhanced Security: By de-identifying data, the risk of a data breach or security incident is significantly reduced. When personal identifiers are removed, the dataset becomes less valuable to potential attackers or hackers, as they cannot link the data back to specific individuals.
3. Facilitates Data Sharing: De-identifying data enables organizations to share datasets without compromising individual privacy. This is particularly useful in collaborative research, healthcare, and other industries where the exchange of data is important for advancements but must be done with privacy in mind.
4. Supports Research and Analysis: De-identified data can be used for research and analysis purposes while maintaining individual privacy. It allows researchers to study population trends, identify patterns, and derive insights without violating privacy regulations or ethical considerations.
5. Compliance with Regulations: De-identifying data helps organizations comply with data protection regulations, such as the General Data Protection Regulation (GDPR) and the Health Insurance Portability and Accountability Act (HIPAA). By removing identifiable information, organizations reduce the risk of non-compliance and potential legal consequences.
6. Ethical Data Use: De-identifying data ensures that organizations handle data in an ethical manner. It demonstrates a commitment to protecting individual privacy rights and promotes responsible data stewardship practices.
7. Improves Data Quality: De-identifying data often involves cleaning and standardizing the dataset, which can lead to improved data quality. By removing errors and inconsistencies, organizations can have more accurate and reliable data for their analysis and decision-making processes.
8. Public Perception and Trust: When organizations adopt measures to de-identify data, it enhances their reputation in the eyes of the public and establishes trust. Individuals are more likely to participate in data collection initiatives and share their information if they feel confident that their privacy is being safeguarded.
Overall, de-identifying data offers several significant benefits, including privacy protection, enhanced security, support for research and analysis, regulatory compliance, and improved public perception. Implementing data de-identification practices should be a priority for organizations to ensure responsible data handling and maintain individual privacy.
Common Techniques for De-Identifying Data
When it comes to safeguarding sensitive information, de-identifying data is an essential practice. By removing or altering identifiable attributes, businesses and organizations can protect privacy and comply with regulations. There are several common techniques for de-identifying data:
1. Anonymization: Anonymization involves removing all personally identifiable information (PII) from a dataset. This can include names, social security numbers, addresses, and other identifying factors. Anonymization ensures that the data cannot be linked back to an individual.
2. Masking: Masking is a technique that replaces sensitive data with fictitious or obfuscated values. Common masking methods include replacing part of a social security number with “X” or “Y” or replacing names with generic placeholders like “John Doe” or “Jane Smith.”
3. Aggregation: Aggregation involves combining multiple individual data points into summarized groups. For example, instead of recording the exact age of individuals, data can be aggregated into age ranges, such as “18-24”, “25-34”, and so on. This helps to preserve privacy while still allowing analysis at a high level.
4. Generalization: Generalization involves rounding or truncating data values to make them less specific. For example, instead of recording an exact salary, it can be rounded to the nearest thousand. This reduces the risk of re-identification while still providing useful insights.
5. Randomization: Randomization involves introducing random noise or perturbations into the data. This can be achieved by adding random values or modifying existing data slightly. Randomization helps to prevent the identification of individuals while maintaining the overall statistical properties of the dataset.
6. Data Encryption: Data encryption involves transforming the data into an unreadable format using encryption algorithms. Only authorized parties with the decryption key can access the original data. Encryption provides a high level of security and privacy for sensitive information.
7. Data Minimization: Data minimization focuses on reducing the overall amount of data collected and stored. By limiting the collection of unnecessary data, the risk of exposure is minimized. Data minimization is achieved by collecting and retaining only the minimum amount of data required for a specific purpose.
Each of these techniques plays a crucial role in protecting privacy and ensuring the security of data. The choice of method depends on the specific requirements and regulations outlined by the industry and jurisdiction. By implementing these techniques effectively, organizations can strike a balance between data utility and privacy protection.
Legal and Ethical Considerations in Data De-Identification
De-identifying data involves the removal or modification of personally identifiable information (PII) from datasets to protect individual privacy and comply with data protection regulations. While de-identification is an essential practice, there are legal and ethical considerations to keep in mind when performing this process.
One of the key legal considerations is ensuring compliance with data privacy laws and regulations. Different regions and countries may have specific legislation, such as the General Data Protection Regulation (GDPR) in the European Union or the Health Insurance Portability and Accountability Act (HIPAA) in the United States. It is crucial to understand and adhere to these regulations to avoid legal consequences.
Another essential legal consideration is maintaining the usability and analyzability of the de-identified data. While the primary goal is to remove personally identifiable information, it is essential to ensure that the data remains useful for research, analysis, or other legitimate purposes. Striking a balance between privacy protection and data utility is crucial to meet legal requirements.
Ethical considerations also play a vital role in data de-identification. Respecting individual privacy is paramount, and data controllers must take appropriate measures to safeguard sensitive information. This includes implementing strict access controls, encryption, and data protection protocols to prevent unauthorized access or breaches.
Transparency and informed consent are ethical principles that must be upheld during the de-identification process. Individuals whose data is being de-identified should be fully aware of how their information is being handled and have the opportunity to provide informed consent. Clearly communicating the purpose, scope, and potential risks associated with data de-identification ensures that individuals can make informed decisions about their personal data.
Additionally, it is essential to consider the potential re-identification risks associated with de-identified data. Even without explicit personally identifiable information, there is a possibility that data can be re-identified through various techniques, such as data linkage or inference attacks. Evaluating and mitigating these risks is crucial to protecting individual privacy and upholding ethical standards.
Furthermore, the principles of data minimization and purpose limitation should be followed when de-identifying data. Data controllers should only collect and retain the minimum necessary data for the intended purpose and ensure that the de-identified data is not used for any other unauthorized purposes.
Overall, legal and ethical considerations are essential when performing data de-identification. Adhering to data protection laws, respecting individual privacy, maintaining data utility, and being transparent and ethical in the process are crucial to ensure the responsible handling of data and protect the rights and privacy of individuals.
Conclusion
In conclusion, de-identifying data is a critical process for organizations that handle sensitive information. By removing personally identifiable information, businesses can protect the privacy and confidentiality of their customers and clients. This not only helps in compliance with data protection regulations but also fosters trust and loyalty among stakeholders. De-identification techniques such as data anonymization, pseudonymization, and aggregation play a crucial role in safeguarding data while still retaining its utility for analysis and research purposes.
It is important for organizations to follow best practices and industry standards when de-identifying data to ensure the effectiveness of the process. By implementing robust security measures, conducting regular audits, and providing training to employees, businesses can minimize the risk of re-identification and potential data breaches. Additionally, staying updated with the latest advancements in data de-identification technologies is essential to keep up with evolving privacy regulations and protect sensitive information.
Overall, de-identifying data is an important aspect of data privacy and security. By implementing proper techniques and safeguards, organizations can strike a balance between utilizing data for insights and preserving the privacy and confidentiality of individuals. Embracing de-identification practices not only enables compliance but also demonstrates a commitment to ethical data handling and builds trust among customers and stakeholders.
FAQs
1. What is data de-identification?
Data de-identification is the process of removing or transforming personal information from datasets to protect individuals’ privacy. It involves modifying or removing identifiable elements such as names, addresses, social security numbers, or any other information that could be used to identify an individual.
2. Why is data de-identification important?
Data de-identification is crucial in ensuring the privacy and security of individuals’ information. By de-identifying data, organizations can minimize the risk of unauthorized access to personally identifiable information (PII) and comply with privacy regulations. It allows for the safe sharing of datasets for research, analytics, and other purposes without compromising individuals’ privacy.
3. What are common methods used for data de-identification?
There are several methods used for data de-identification, including:
– Anonymization: Removing direct identifiers such as names, addresses, and social security numbers from the dataset.
– Masking: Replacing sensitive information with pseudonyms or symbols.
– Generalization: Aggregating or rounding data to broader categories to prevent identification.
– Tokenization: Replacing identifying data with randomly generated unique tokens.
– Data perturbation: Adding random noise or altering the values of data to make identification difficult.
4. Are there any risks associated with data de-identification?
While data de-identification can significantly reduce the risk of privacy breaches, it is not foolproof. There is always a possibility of re-identification if additional information is combined with the de-identified dataset. This is known as a re-identification risk. Organizations must carefully evaluate and assess the potential risks involved in sharing de-identified data and take necessary precautions to mitigate these risks.
5. What are some best practices for data de-identification?
To ensure effective data de-identification and minimize re-identification risks, it is recommended to follow these best practices:
– Use a combination of de-identification techniques to maximize privacy protection.
– Conduct thorough risk assessments and evaluate the potential re-identification risks.
– Implement data governance policies and procedures to ensure proper handling of de-identified data.
– Regularly review and update de-identification processes to stay up-to-date with evolving privacy standards and regulations.
– Educate employees about the importance of data de-identification and the proper handling of de-identified datasets.
– Consider obtaining external expertise or consulting privacy professionals to ensure compliance with privacy laws and regulations.