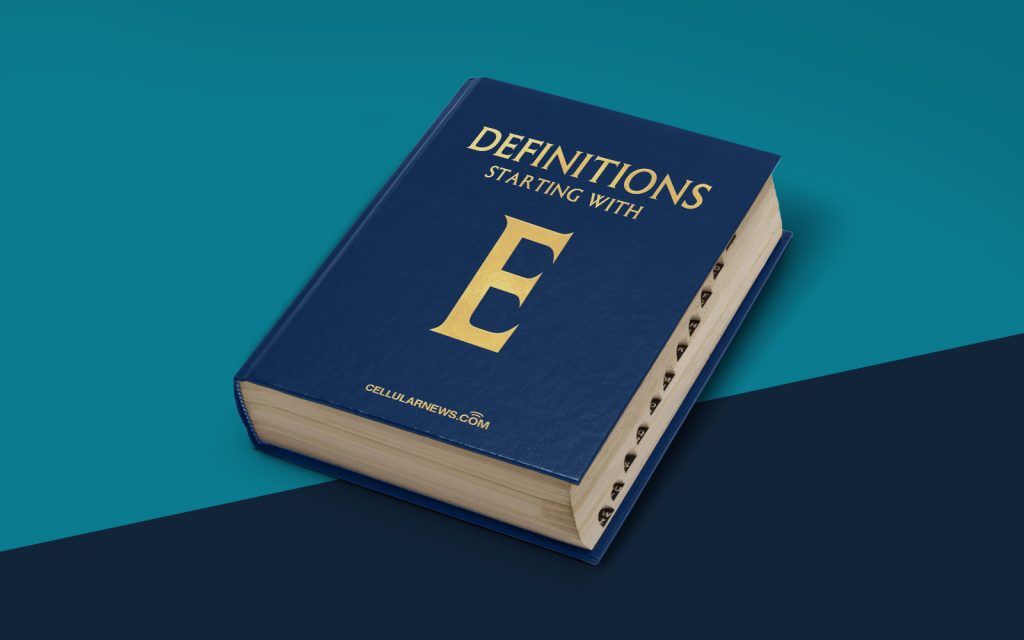
Unveiling the Secrets of Exploratory Data Analysis (EDA)
Imagine stepping into a room filled with scattered puzzle pieces. You have no idea how they fit together or what the completed image looks like. What would you do? Would you start piecing them together randomly, hoping that eventually, something coherent would emerge? Or would you take a closer look at each piece, examining its shape, color, and pattern, to gain insights into the bigger picture?
Key Takeaways:
- EDA is a powerful data analysis technique that helps in understanding the structure and properties of a dataset.
- It involves visualizing and summarizing the data to unveil interesting trends, detect anomalies, and identify potential relationships between variables.
When facing a dataset, we often find ourselves in a similar situation. We are presented with a vast amount of information, but without guidance, the data may appear disjointed and overwhelming. This is where Exploratory Data Analysis (EDA) comes into play.
But what exactly is Exploratory Data Analysis (EDA)?
At its core, Exploratory Data Analysis (EDA) is a strategy used by data scientists and analysts to unravel the mysteries hiding within datasets. It enables us to observe, understand, and extract meaningful insights from the data, helping us make informed decisions and gain a deeper understanding of the underlying patterns and relationships.
So, how does Exploratory Data Analysis work its magic? Let’s find out:
- Understanding the data: EDA begins by gaining a holistic understanding of the dataset. We examine the variables, their types, and the overall structure of the data. This step helps us identify potential issues, such as missing values or outliers, which may affect the quality of our analysis.
- Visualizing the data: Visualization plays a crucial role in exploring and interpreting the data effectively. By plotting charts, histograms, scatter plots, and other visual representations, we can gain valuable insights into the distribution of the variables, identify trends, and detect patterns and outliers that might have gone unnoticed otherwise.
- Summarizing the data: EDA involves summarizing the data through various statistical measures such as mean, median, standard deviation, and percentiles. These summaries provide a snapshot of the dataset, allowing us to understand its central tendencies, variability, and the presence of any significant outliers or skewness.
- Identifying relationships: Exploratory Data Analysis helps us uncover relationships between variables. By visualizing and analyzing correlations, we can better understand how variables interact with one another. This knowledge can be crucial in building predictive models or making data-driven decisions.
- Driving insights: EDA explorations often lead us to unexpected discoveries and insights about the data. It can help us formulate new questions or hypotheses, guiding further analysis and informing decision-making processes.
Exploratory Data Analysis (EDA) is not just a tool, but an art that allows us to dive deep into the world of data. It empowers us to transform complex and intricate datasets into understandable and actionable information. By leveraging EDA, we can unlock the potential hidden within the data and embrace a data-driven approach in our decision-making processes.
So, the next time you encounter a puzzling dataset, remember the power of EDA. Embrace the scattered pieces, observe their details, and unravel the hidden patterns. In doing so, you’ll unlock a world of insights and embark on an exciting journey of data exploration.