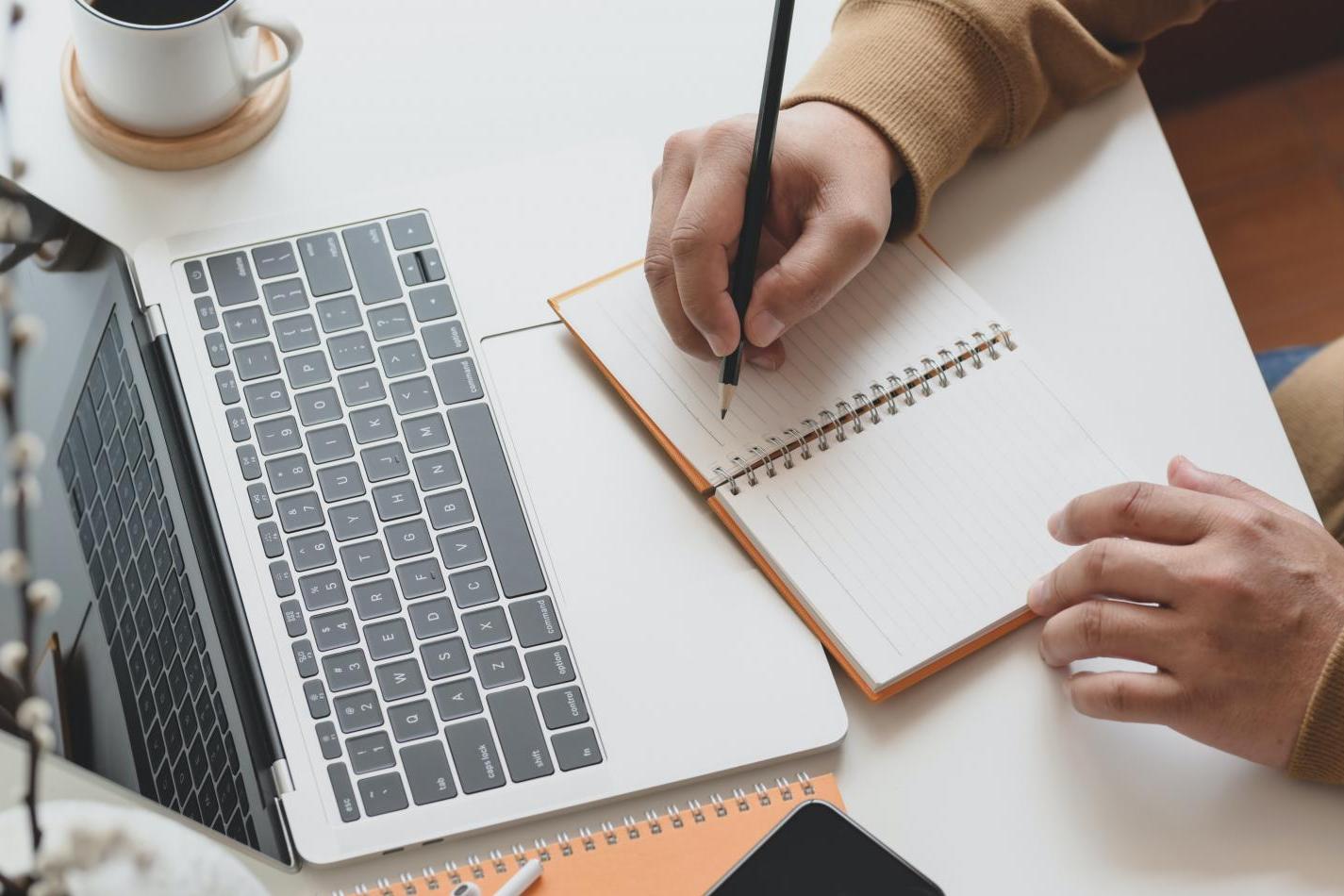
In today’s world, data analysis has become a crucial aspect of various academic disciplines and industries. It is a process that involves careful examination, interpretation, and evaluation of data to uncover meaningful insights and patterns. For students pursuing higher education, writing a data analysis dissertation is an essential requirement to showcase their research skills and expertise in their chosen field.
A data analysis dissertation explores a specific research question or problem by collecting, organizing, and analyzing data. It requires a systematic approach, critical thinking, and advanced statistical techniques to derive valid conclusions and contribute to the existing body of knowledge. This guide is designed to help you navigate through the process of writing a data analysis dissertation, providing you with valuable tips, techniques, and resources to ensure a successful and impactful research endeavor.
Inside This Article
- Choosing a Research Topic
- Formulating Research Questions
- Selecting and Gathering Data
- Data Cleaning and Preparation
- Exploratory Data Analysis
- Statistical Analysis Methods
- Interpreting and Presenting Results
- Writing the Data Analysis Chapter
- Conclusion
- FAQs
Choosing a Research Topic
Choosing a research topic is a crucial step in writing a data analysis dissertation. It sets the direction and scope of your study, and ultimately determines the overall success of your research. Here are some key considerations to keep in mind when selecting a research topic:
1. Personal Interest: Start by identifying subjects or areas that genuinely interest you. Think about your passion and curiosity, as this will help maintain your enthusiasm throughout the research process.
2. Relevance: Ensure that your research topic is relevant to your field of study. It should address a gap in existing knowledge or contribute to the advancement of your academic discipline.
3. Feasibility: Consider the resources and time available to you. Make sure the research topic is feasible within the constraints of your academic program and the limitations of your data collection methods.
4. Originality: Aim for a research topic that is unique and original. While building upon existing literature is essential, try to add a fresh perspective or approach that brings something new to the table.
5. Significance: Evaluate the potential impact of your research topic. Ask yourself how it can contribute to the broader academic community or address real-world issues.
6. Consultation: Seek input from your advisor or dissertation committee members. They can provide guidance, offer suggestions, and help ensure that your research topic is well-aligned with your academic goals.
7. Flexibility: Keep in mind that your research topic may evolve throughout the course of your dissertation. It’s important to choose a topic that allows for flexibility and adaptability as you delve deeper into the data analysis phase.
By keeping these considerations in mind, you can select a research topic that not only captivates your interest but also contributes meaningfully to your field of study. Remember, your research topic is the foundation upon which your entire dissertation will be built, so choose wisely!
Formulating Research Questions
Formulating research questions is a crucial step in any data analysis dissertation. Research questions provide the foundation for your entire study, guiding the data collection and analysis process. They help identify the purpose of your research, outline the variables you will investigate, and provide a framework for interpreting the results.
When formulating research questions, it is important to ensure that they are focused, clear, and answerable. Here are some key considerations to keep in mind:
- Identify your research goals: Start by clearly defining what you want to achieve with your study. Are you trying to uncover trends, establish relationships between variables, or explore new phenomena? Clearly understanding your research goals will help you formulate appropriate research questions.
- Consider your research design: The type of study you are conducting will influence the nature of your research questions. For example, if you are conducting a quantitative study, you may formulate questions that seek to establish causal relationships, whereas in a qualitative study, you may focus on exploring experiences and perceptions.
- Brainstorm possible questions: Take some time to brainstorm potential research questions related to your topic. Consider different angles and perspectives that could be explored. It can be helpful to consult existing literature to get ideas or inspirations for research questions.
- Narrow down your questions: Once you have generated a list of potential research questions, it’s important to narrow down and refine them. Consider the feasibility of answering each question within the scope of your study. Focus on questions that align with your research goals and are realistically addressable.
- Ensure clarity and specificity: Your research questions should be clear, concise, and specific. Avoid vague or broad questions that may make it difficult to collect and analyze data. Instead, focus on questions that can be easily understood and targeted in your research.
Remember that research questions should guide your data collection and analysis process. They should be the driving force behind the methodology you choose, the data you collect, and the analysis techniques you employ. By formulating well-defined research questions, you can ensure that your data analysis dissertation is both focused and meaningful.
Selecting and Gathering Data
When it comes to writing a data analysis dissertation, one of the crucial steps is selecting and gathering the data needed for your study. This process requires careful consideration and planning to ensure that you obtain reliable and valid data to support your research objectives. Here are some key points to keep in mind:
1. Define your research questions: Before selecting and gathering data, you need to have a clear understanding of your research questions. These questions will guide you in determining the type of data you need to collect and the specific variables that are relevant to your study.
2. Identify the sources of data: Depending on the nature of your research, there are various sources of data that you can explore. These may include primary sources such as surveys, experiments, observations, or interviews, or secondary sources such as existing datasets, literature reviews, or archival records.
3. Consider the accessibility and availability of data: It’s important to assess whether the data you need is accessible and available. This involves considering factors such as data ownership, permission requirements, and potential restrictions or limitations in accessing certain datasets.
4. Evaluate the quality of data: Before incorporating data into your analysis, it’s crucial to evaluate its quality. This includes assessing its validity, reliability, and relevance to your research questions. You may need to carefully review the data collection methodology, check for any biases or errors, and ensure that it aligns with your research objectives.
5. Determine the sample size and sampling method: Depending on the scope of your study, you may need to select a representative sample from the population of interest. This involves determining the appropriate sample size and selecting a suitable sampling method, such as random sampling, stratified sampling, or convenience sampling.
6. Plan for data collection: Once you have identified the sources and determined the sample size and sampling method, it’s time to plan for data collection. This includes designing data collection instruments, such as surveys or interview protocols, and establishing a timeline and procedures for gathering the data.
7. Record and organize the data: As you gather the data, it’s important to establish a system for recording and organizing it. This may involve creating a dataset or spreadsheet, keeping track of data sources and variables, and ensuring proper documentation for future reference.
By carefully selecting and gathering the right data for your analysis, you are setting the foundation for a robust and rigorous dissertation. Remember to align your data collection process with your research questions and objectives, and maintain a systematic approach to ensure the integrity and validity of your findings.
Data Cleaning and Preparation
Data cleaning and preparation play a crucial role in the data analysis process. Before diving into the analysis, it is essential to ensure that the data is in the right format and free from any errors or inconsistencies. This step is necessary to maintain the integrity and reliability of the data.
The first step in data cleaning is to check for missing values. Missing data can create bias and affect the accuracy of the analysis. It is important to identify the missing values and decide on the appropriate treatment method. This can involve either deleting the incomplete records or imputing the missing values using statistical techniques.
Next, it is important to address any outliers or anomalies in the data. Outliers are extreme values that can skew the results of the analysis. Identifying and handling outliers can involve techniques such as Winsorization, where extreme values are replaced with a less extreme value within a specified range.
Another crucial aspect of data cleaning is dealing with categorical variables. Categorical variables are non-numeric variables that represent different categories or groups. They need to be properly encoded or transformed into a numeric format to be included in the analysis. This can be done through techniques such as one-hot encoding or label encoding.
Furthermore, data cleaning also involves checking for data integrity and consistency. This includes ensuring that variables are recorded in the right units and formats, and that there are no duplicate or contradictory records. Data validation techniques, such as cross-referencing with external sources or conducting logical checks, can be used to ensure data integrity.
Lastly, it is important to perform data normalization or scaling before conducting any statistical analysis. Normalization ensures that all variables are on the same scale, preventing certain variables dominating the analysis due to their larger magnitude. Common techniques for normalization include z-score normalization and min-max scaling.
Exploratory Data Analysis
Exploratory Data Analysis (EDA) is a crucial step in the data analysis process. It involves exploring and understanding the dataset to uncover patterns, trends, and hidden insights. EDA is a powerful tool that helps researchers gain a deeper understanding of their data before applying more advanced statistical techniques.
During the EDA phase, researchers examine the variables in the dataset and their relationships to identify any outliers, missing values, or anomalies that may require further investigation or cleaning. This initial exploration sets the foundation for a thorough analysis and interpretation of the data.
One of the primary goals of EDA is to visually represent the data using various statistical graphics and plots. Histograms, box plots, scatter plots, and bar charts are commonly used to understand the distribution, central tendencies, and relationships between variables. These visualizations provide insights into the shape of the data and any potential patterns or trends that may exist.
EDA also involves calculating descriptive statistics such as mean, median, standard deviation, and correlation coefficients. These summary statistics provide a numerical summary of the dataset and help researchers identify key characteristics and relationships between variables.
Another important aspect of EDA is identifying and addressing missing values or outliers in the dataset. Missing data can significantly impact the validity of the analysis, so it is crucial to identify and handle them appropriately. Researchers may choose to impute missing values or remove observations with excessive missing data, depending on the context and data quality.
EDA is an iterative process that requires the researcher to analyze and explore the data from different angles. This involves identifying subsets of interest, conducting conditional analyses, and performing significance tests to validate findings. The aim is to uncover patterns, relationships, and trends that may guide further analyses or hypothesis development.
Overall, exploratory data analysis is an essential step in understanding the characteristics and insights hidden within a dataset. By visually exploring and summarizing the data, researchers can make informed decisions about the subsequent steps in their data analysis process.
Statistical Analysis Methods
When it comes to data analysis in a dissertation, statistical analysis methods play a crucial role in uncovering meaningful insights from the collected data. These methods allow researchers to analyze and interpret the data, making them an integral part of the research process. Here are some common statistical analysis methods used in data analysis dissertations:
1. Descriptive Statistics: Descriptive statistics involve summarizing and describing the main features of the data, such as measures of central tendency (mean, median, mode) and measures of variability (standard deviation, range).
2. Inferential Statistics: Inferential statistics are used to make inferences or predictions about a population based on sample data. This includes hypothesis testing, confidence intervals, and regression analysis.
3. Chi-Square Analysis: Chi-square analysis is used to determine if there is a significant association between categorical variables. It is often used to analyze survey data or test hypotheses about the relationship between variables.
4. t-tests: T-tests are used to compare the means of two groups and determine if there is a significant difference. This method is commonly used in experiments where researchers want to compare the effectiveness of different treatments or interventions.
5. ANOVA: Analysis of Variance (ANOVA) is used to compare the means of three or more groups to determine if they are significantly different. This method is useful when there are multiple treatment groups or when comparing the effects of different variables on the outcome.
6. Regression Analysis: Regression analysis is used to examine the relationship between a dependent variable and one or more independent variables. It helps identify the strength and direction of the relationship, as well as predicting the value of the dependent variable based on the independent variables.
7. Factor Analysis: Factor analysis is used to identify underlying factors or dimensions in a dataset. It helps reduce the complexity of the data by grouping variables with similar characteristics and identifying the underlying constructs.
8. Cluster Analysis: Cluster analysis is used to identify groups or clusters based on similarities between variables or cases. It is particularly useful in segmentation analysis, where researchers want to identify distinct profiles or segments within a larger dataset.
9. Time Series Analysis: Time series analysis is used to analyze data that is collected over time, such as stock prices, sales data, or weather patterns. It helps identify trends, patterns, and forecast future values based on historical data.
10. Survival Analysis: Survival analysis is used to analyze time-to-event data, such as the time it takes for a patient to develop a certain condition or experience an event. It helps estimate survival probabilities and identify factors that influence the time to the event of interest.
These are just a few examples of statistical analysis methods that researchers can use to analyze their data in a dissertation. The choice of method depends on the research questions, study design, and type of data collected. It is important to select the appropriate statistical analysis method that aligns with the research objectives and provides meaningful insights.
Interpreting and Presenting Results
Once you have completed the statistical analysis of your data, the next crucial step in writing your data analysis dissertation is interpreting and presenting the results. This is where you make sense of the numbers and statistics and extract meaningful insights from your data.
Interpreting the results involves examining the statistical significance of your findings and determining the implications of your research. It is important to carefully analyze each statistical measure and consider its relevance to your research questions or hypotheses.
Key points to consider when interpreting the results:
- Statistical significance: Determine if your results are statistically significant. This indicates whether the observed differences or relationships in the data are likely to be due to chance or if they are truly meaningful.
- Effect size: Assess the magnitude of the effect or relationship observed in the data. A large effect size indicates a strong relationship, while a small effect size suggests a weak or negligible relationship.
- Contextual interpretation: Consider the broader context of your research and how your findings relate to existing literature or theories. Discuss any unexpected or contradictory results and offer possible explanations.
- Limitations: Acknowledge the limitations of your study and the potential biases or weaknesses that may have influenced the results. This demonstrates your awareness of the study’s shortcomings and enhances the credibility of your interpretation.
After interpreting the results, it’s time to present them in a clear and organized manner. Effective presentation of the results is essential for conveying your findings to your audience and supporting your arguments.
Tips for presenting results:
- Data visualization: Use charts, graphs, and tables to visually represent your findings. Visuals can make complex data more accessible and facilitate better understanding.
- Clear labeling and captions: Ensure that all elements in your visual representations are clearly labeled and accompanied by appropriate captions. This provides context and enhances comprehension.
- Concise and focused explanations: Explain the key highlights of your findings in a succinct and focused manner. Avoid unnecessary technical jargon and provide enough context for non-experts to grasp the main insights.
- Include supporting evidence: Refer to relevant statistical measures, significant findings, or notable trends to back up your interpretations. This adds credibility and strengthens the validity of your results.
- Engage with the reader: Provide a narrative that guides the reader through your findings, explaining the implications and potential real-world applications. Engaging the reader’s interest can make your results more compelling and memorable.
Remember, the ultimate goal of interpreting and presenting the results is to communicate your research findings effectively. By providing a clear and comprehensive explanation of your data analysis, you can contribute to the existing body of knowledge and make a significant contribution to your field of study.
Writing the Data Analysis Chapter
The data analysis chapter is a crucial component of your dissertation, as it presents the findings and interpretation of your research. This chapter provides the opportunity to showcase your analytical skills and demonstrate how you have addressed your research questions or hypotheses.
To write an effective data analysis chapter, consider the following steps:
-
Begin by revisiting your research questions or hypotheses. The data analysis chapter should aim to answer these questions or test these hypotheses based on the data you have collected.
-
Organize your analysis logically. Start by describing the characteristics of your data set, including the type of data collected and any limitations or missing values.
-
Choose appropriate analytical techniques for your data set. This may involve using statistical tests, regression models, or qualitative analysis methods, depending on the nature of your research and the data collected.
-
Explain the steps you took to analyze the data. Provide details about the software or tools used, the parameters selected, and any preprocessing or cleaning steps undertaken.
-
Present your findings in a clear and concise manner. Use tables, graphs, or charts to illustrate your results. Provide a narrative explanation of what the data shows and how it relates to your research questions.
-
Interpret your results and discuss their implications. Connect your findings back to your research questions and explain any patterns, trends, or relationships that emerged from the analysis. Highlight both the significance and limitations of your findings.
-
Address any unexpected or conflicting results. If your analysis produces unexpected or contradictory findings, discuss potential explanations or alternative interpretations.
-
Relate your results to existing literature. Discuss how your findings align or contradict with previous research in your field. Identify areas for further investigation or suggest potential contributions to the existing body of knowledge.
Throughout the chapter, use clear and concise language to communicate your analysis. Avoid jargon or technical terms that may confuse your readers. Additionally, provide sufficient detail for readers to understand and evaluate your analysis.
Remember to adhere to the formatting guidelines set by your institution or department. This includes properly citing any sources or references used in your analysis. Consistency in formatting and style enhances the professionalism of your work.
Writing the data analysis chapter requires careful attention to detail and a solid understanding of your research objectives. By following these guidelines, you can create a compelling and informative chapter that showcases your findings and contributes to the knowledge in your field.
Conclusion
In conclusion, writing a data analysis dissertation can be a challenging but rewarding endeavor. It requires careful planning, diligent research, and a systematic approach to analyzing and interpreting data. By following the steps outlined in this guide, you can effectively navigate the process and produce a high-quality dissertation that contributes to the field of data analysis.
Remember to start with a clear research question, collect comprehensive and reliable data, choose appropriate analysis methods, and interpret the results accurately. Additionally, make sure to organize your dissertation logically, use proper citation techniques, and proofread thoroughly to ensure clarity and coherence.
Ultimately, writing a data analysis dissertation is an opportunity to showcase your expertise and make a valuable contribution to the field. Through rigorous analysis and thoughtful interpretation, you can uncover insights and draw meaningful conclusions that advance knowledge in your area of study. Embrace the challenge, stay focused, and remember that every step you take brings you one step closer to completing your dissertation successfully.
FAQs
1. What is a data analysis dissertation?
A data analysis dissertation is a research project that focuses on analyzing and interpreting data to address a specific research question or hypothesis. It involves collecting and organizing data, employing statistical methods and techniques, and drawing meaningful conclusions from the analysis.
2. How do I choose a topic for my data analysis dissertation?
Choosing a topic for your data analysis dissertation can be an exciting yet challenging task. Start by identifying an area of interest within your field of study. Consider current research gaps, emerging trends, and real-world problems that need further investigation. Consult with your advisor or mentor for guidance and narrowing down your topic based on the available data and resources.
3. What kind of data can be used in a data analysis dissertation?
Data used in a data analysis dissertation can vary depending on the research field and the objectives of the study. Common types of data include quantitative data (numerical or categorical) and qualitative data (texts, interviews, observations). The data can be collected through surveys, experiments, existing datasets, or a combination of different sources.
4. What software or tools can I use for data analysis in my dissertation?
There are several software and tools available for data analysis, depending on the type of data and the complexity of the analysis. Popular options include statistical software like SPSS, SAS, and R, which offer a range of statistical tests, data visualization, and modeling capabilities. Additionally, Excel and Python programming language can also be used for data manipulation and analysis.
5. How do I present the findings of my data analysis dissertation?
Presenting the findings of your data analysis dissertation requires clear and concise communication. Begin by providing an overview of the research question and methodology. Then, present the analyzed data using appropriate tables, graphs, or charts. Explain the results, including any significant findings or patterns observed. Finally, discuss the implications of your findings and how they contribute to the existing knowledge in your field.