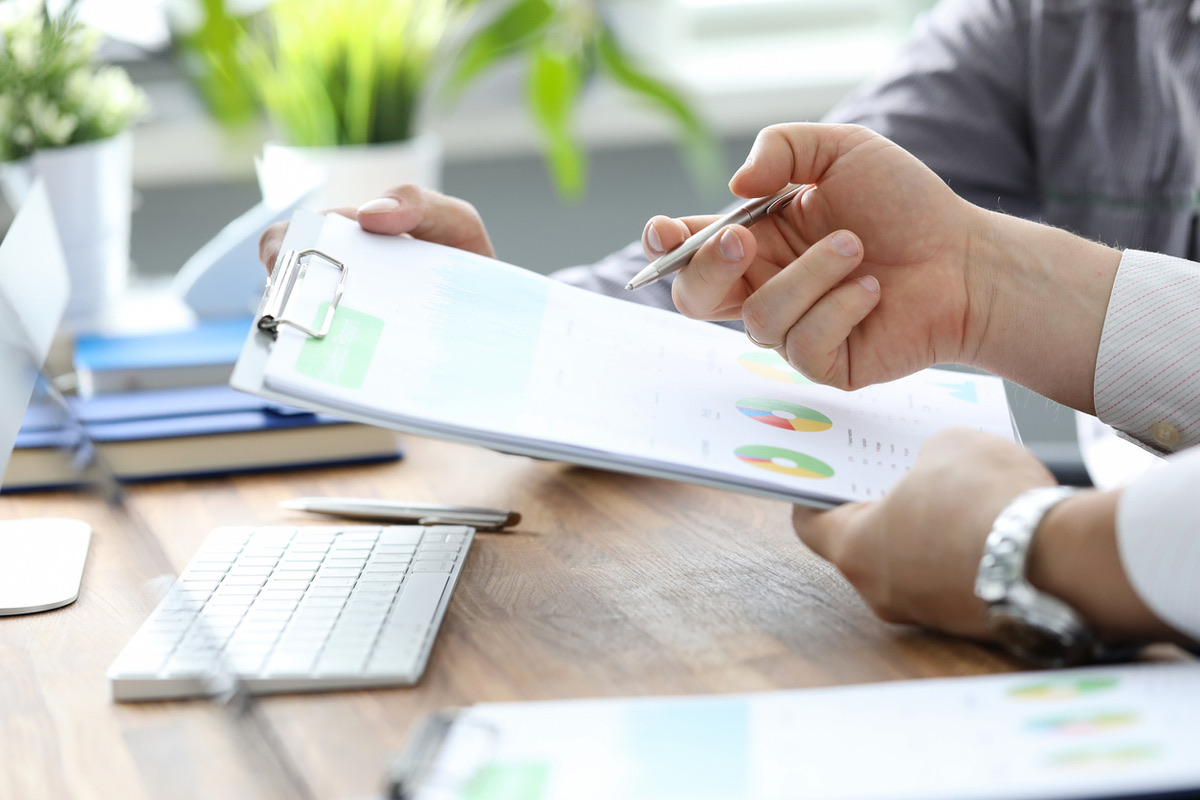
Data quality issues can have a significant impact on the efficiency and accuracy of any organization. In today’s data-driven world, where information is crucial for decision-making and analysis, it is crucial to address and resolve data quality issues promptly. Whether it’s incomplete, inconsistent, or inaccurate data, these issues can lead to misinformed decisions, poor customer experiences, and missed business opportunities.
In this comprehensive guide, we will explore the common causes of data quality issues and provide practical tips on how to identify, assess, and resolve them. By addressing data quality issues head-on, businesses can ensure the integrity and reliability of their data, resulting in more informed decision-making, improved operational efficiency, and enhanced customer satisfaction.
Inside This Article
- Common Data Quality Issues
- Understanding the Impact of Data Quality Issues
- Strategies to Improve Data Quality
- Best Practices for Addressing Data Quality Issues
- Conclusion
- FAQs
Common Data Quality Issues
Data plays a crucial role in today’s digital world, influencing business decisions, customer experience, and overall operations. However, maintaining high-quality data can be challenging, as there are several common data quality issues that organizations often face. Let’s explore some of these issues:
1. Inaccurate Data: Inaccurate data is one of the most prevalent data quality issues. It occurs when the information recorded is incorrect or outdated. This can lead to flawed analysis, poor decision-making, and negative customer experiences.
2. Duplicate Data: Duplicate data refers to the presence of the same information multiple times within a dataset. It can arise due to human error, system glitches, or data integration problems. Duplicate data can distort reporting, waste storage space, and skew analysis results.
3. Incomplete Data: Incomplete data refers to missing or lacking information within a dataset. It can occur when data collection processes are not thorough or when data is not updated regularly. Incomplete data can hinder accurate analysis, impede decision-making, and result in incomplete customer profiles.
4. Inconsistent Data: Inconsistent data occurs when the same type of information is recorded differently across different records or systems. It can stem from varying data entry practices, different data sources, or system integrations. Inconsistent data can lead to difficulties in data analysis, confusion, and errors in reporting.
5. Irrelevant Data: Irrelevant data refers to information that is not applicable or useful for the intended purpose. It can include outdated records, irrelevant attributes, or data that is no longer relevant due to changes in business requirements. Irrelevant data can clutter databases, increase storage costs, and hinder effective analysis.
6. Data Integrity Issues: Data integrity issues include data that has been compromised, tampered with, or corrupted. This can occur due to security breaches, unauthorized access, or system failures. Data integrity issues can lead to mistrust in the data, compromised decision-making, and potential legal and regulatory implications.
7. Data Inconsistency Across Systems: Data inconsistency across systems happens when different systems or applications in an organization store and manage data differently. This can create challenges when integrating data from multiple sources, making data analysis and reporting complex and time-consuming.
8. Data Governance and Compliance: Data governance and compliance issues arise when organizations fail to establish proper data governance practices and adhere to regulatory requirements. This can lead to data privacy breaches, non-compliance penalties, and damage to the organization’s reputation.
By addressing these common data quality issues, organizations can ensure that they have accurate, complete, and reliable data to support their business operations, decision-making processes, and customer-centric initiatives.
Understanding the Impact of Data Quality Issues
When it comes to managing data, quality is paramount. Data quality issues can have a significant impact on businesses, affecting decision-making, customer satisfaction, and overall operational efficiency. Understanding the consequences of these issues is vital in order to address them effectively.
Poor data quality can lead to incorrect and unreliable information. This, in turn, can result in flawed decisions that can have far-reaching consequences. Imagine relying on inaccurate sales data to forecast future revenue or basing marketing campaigns on outdated customer information. The potential for costly mistakes and missed opportunities is high.
Data quality issues can also erode customer trust and satisfaction. Inaccurate or incomplete customer data can lead to communication errors, such as sending promotional materials to the wrong addresses or contacting customers who have opted out. This can damage your brand reputation and lead to customer churn.
Moreover, data quality problems can have a domino effect throughout an organization. Inaccurate or inconsistent data can make it difficult for teams to collaborate effectively and can hinder cross-departmental operations. The time and effort spent on addressing data discrepancies can divert resources from more valuable tasks, reducing productivity and efficiency.
Another consequence of data quality issues is compliance and regulatory risks. Depending on the industry, organizations may be subject to data protection regulations, such as the General Data Protection Regulation (GDPR). Failing to maintain accurate and secure data can result in hefty fines and legal repercussions.
Additionally, poor data quality can hinder business intelligence and analytics initiatives. Reliable and high-quality data is essential for generating meaningful insights and making informed business decisions. Without trust in the data, analytics efforts can be compromised, leading to missed opportunities and subpar performance.
Overall, the impact of data quality issues is multi-faceted and can have far-reaching consequences. It is essential for organizations to prioritize data quality and implement strategies to address and mitigate these issues effectively.
Strategies to Improve Data Quality
Data quality issues can have a significant impact on an organization’s operations, decision-making, and overall efficiency. To address these issues, it is crucial to implement effective strategies that can improve the quality of your data. Here are some key strategies to consider:
1. Define Data Quality Standards: Start by establishing clear and specific data quality standards for your organization. This includes defining the accuracy, completeness, consistency, and validity criteria for your data. By having these standards in place, you can ensure that everyone understands the expectations for data quality.
2. Conduct Data Audits: Regularly perform data audits to identify and correct any inconsistencies or errors in your data. This involves analyzing data records, comparing them against the defined data quality standards, and flagging any discrepancies that need attention. Data audits help increase data accuracy and reliability.
3. Implement Data Validation: Set up data validation rules and mechanisms to check the integrity and validity of your data. This can include automated checks for duplicate records, data formatting errors, and missing information. Data validation helps prevent the entry of incorrect or incomplete data into your system.
4. Improve Data Entry Processes: Review and refine your data entry processes to minimize the likelihood of errors. This can involve providing training to data entry personnel, implementing validation checks during data entry, and using data entry templates or forms to ensure consistency. Streamlining data entry processes can significantly improve data quality.
5. Establish Data Governance: Create a robust data governance framework that outlines the roles, responsibilities, and processes for managing and maintaining data quality. This includes assigning data stewards who are responsible for monitoring and enforcing data quality standards, as well as establishing data quality management processes and procedures.
6. Regularly Cleanse and Standardize Data: Implement routine data cleansing and standardization processes to remove any duplicate or irrelevant data, correct formatting errors, and ensure consistent data values. This involves using data cleansing tools or software that can identify and fix common data quality issues automatically.
7. Enhance Data Integration and Data Quality Tools: Invest in tools and technologies that can improve data integration and data quality management. These tools can help automate data validation, cleansing, and standardization processes, as well as provide real-time data quality monitoring and reporting capabilities.
8. Foster Data Quality Awareness: Educate and train your employees on the importance of data quality and their role in maintaining it. Promote a culture of data quality awareness and accountability throughout the organization. This can include providing ongoing training programs, creating data quality guidelines, and rewarding employees for their contributions to data quality improvement.
By implementing these strategies, you can proactively address data quality issues and ensure that your organization has reliable and accurate data to drive decision-making and operational excellence.
The section title “
Best Practices for Addressing Data Quality Issues
” indicates that you will be discussing the best approaches to tackle data quality issues. Let’s dive into some key strategies that can help organizations navigate and overcome these challenges.
1. Identify the root cause: The first step in addressing data quality issues is to identify the underlying cause. This involves conducting a thorough analysis of the data to determine where the errors or inconsistencies are originating from. By understanding the root cause, organizations can effectively implement targeted solutions.
2. Establish data quality standards: It is crucial to establish clear data quality standards that define the accuracy, completeness, and consistency required for the data. These standards should be aligned with the specific needs and objectives of the organization. By clearly defining these standards, organizations can set a benchmark for data quality and ensure that it remains consistent over time.
3. Implement data validation processes: Implementing robust data validation processes is essential in maintaining data quality. This involves checking data for accuracy, completeness, and consistency at various stages, such as data entry, integration, and migration. By validating data against predefined rules and standards, organizations can identify and correct any discrepancies in the data.
4. Adopt data cleansing techniques: Data cleansing involves identifying and correcting errors, inconsistencies, and inaccuracies within the data. This can include removing duplicate records, standardizing formatting, and correcting typographical errors. Adopting automated data cleansing techniques can significantly streamline this process, ensuring that the data remains accurate and reliable.
5. Promote data governance: Data governance refers to the overall management of data within an organization. Establishing a data governance framework helps in defining roles, responsibilities, and processes for ensuring data quality. It ensures that there are clear accountability and ownership of data quality issues, along with proper data management practices across the organization.
6. Conduct regular data audits: Regular data audits are crucial to monitor and assess the overall health of an organization’s data. This involves conducting periodic reviews and inspections to identify any data quality issues that may have emerged. By regularly auditing the data, organizations can proactively address any anomalies and improve data quality continually.
7. Invest in data quality tools and technologies: There are various data quality tools and technologies available in the market that can assist organizations in addressing data quality issues. These tools can help automate data validation, cleansing, and monitoring processes, improving efficiency and accuracy. Investing in such tools can significantly enhance an organization’s ability to manage and maintain data quality.
8. Establish data quality metrics and monitoring: It is essential to establish data quality metrics and continuously monitor them to track the progress of data quality improvement efforts. This can include metrics such as data accuracy rates, completeness levels, and error rates. Regular monitoring allows organizations to identify trends, measure the effectiveness of implemented solutions, and make necessary adjustments to improve data quality further.
By following these best practices, organizations can effectively address data quality issues and ensure that their data remains clean, accurate, and reliable. By doing so, they can make informed decisions, enhance operational efficiency, and maintain a competitive edge in today’s data-driven business environment.
Conclusion
Addressing data quality issues is crucial for any organization that wants to make informed decisions and achieve operational efficiency. By recognizing the importance of data quality and implementing the right strategies, businesses can mitigate the risks associated with poor data quality and unlock the full potential of their data.
With the right tools and expertise, organizations can establish robust data governance frameworks, implement data validation and cleansing processes, and ensure data accuracy, consistency, completeness, and timeliness. Prioritizing data quality also leads to improved customer experiences, better decision-making, and increased competitiveness in the market. By investing in data quality initiatives, businesses can harness the power of reliable and trustworthy data to drive growth, innovation, and success.
So, don’t let data quality issues hold your organization back. Take action today and pave the way for better data-driven insights and outcomes.
FAQs
1. Why is data quality important?
Data quality is important because it directly impacts the reliability and credibility of the information being used for decision-making, analysis, and reporting. Poor data quality can lead to inaccurate insights, ineffective strategies, and ultimately, wasted time and resources.
2. What are some common data quality issues?
Some common data quality issues include duplicate records, incomplete or missing data, inconsistencies, outdated information, and inaccuracies. These issues can arise due to manual data entry errors, system glitches, data integration problems, or inadequate data validation processes.
3. How can I identify data quality issues?
To identify data quality issues, you can perform data profiling and analysis. This involves examining the data for patterns, inconsistencies, and anomalies. You can also implement data quality metrics and measures to assess the accuracy, completeness, validity, and timeliness of the data.
4. What are the consequences of ignoring data quality issues?
Ignoring data quality issues can have significant negative consequences. It can lead to incorrect analysis and decisions, damage to the organization’s reputation, regulatory compliance issues, and financial losses. It can also erode trust among stakeholders and hinder data-driven initiatives.
5. What are some strategies to address data quality issues?
To address data quality issues, you can implement data cleansing techniques such as removing duplicate records, filling in missing data, and standardizing inconsistent values. Regular data audits and monitoring can help identify and resolve data quality issues. Establishing data governance practices, defining data quality standards, and involving stakeholders in data quality improvement efforts are also important strategies.