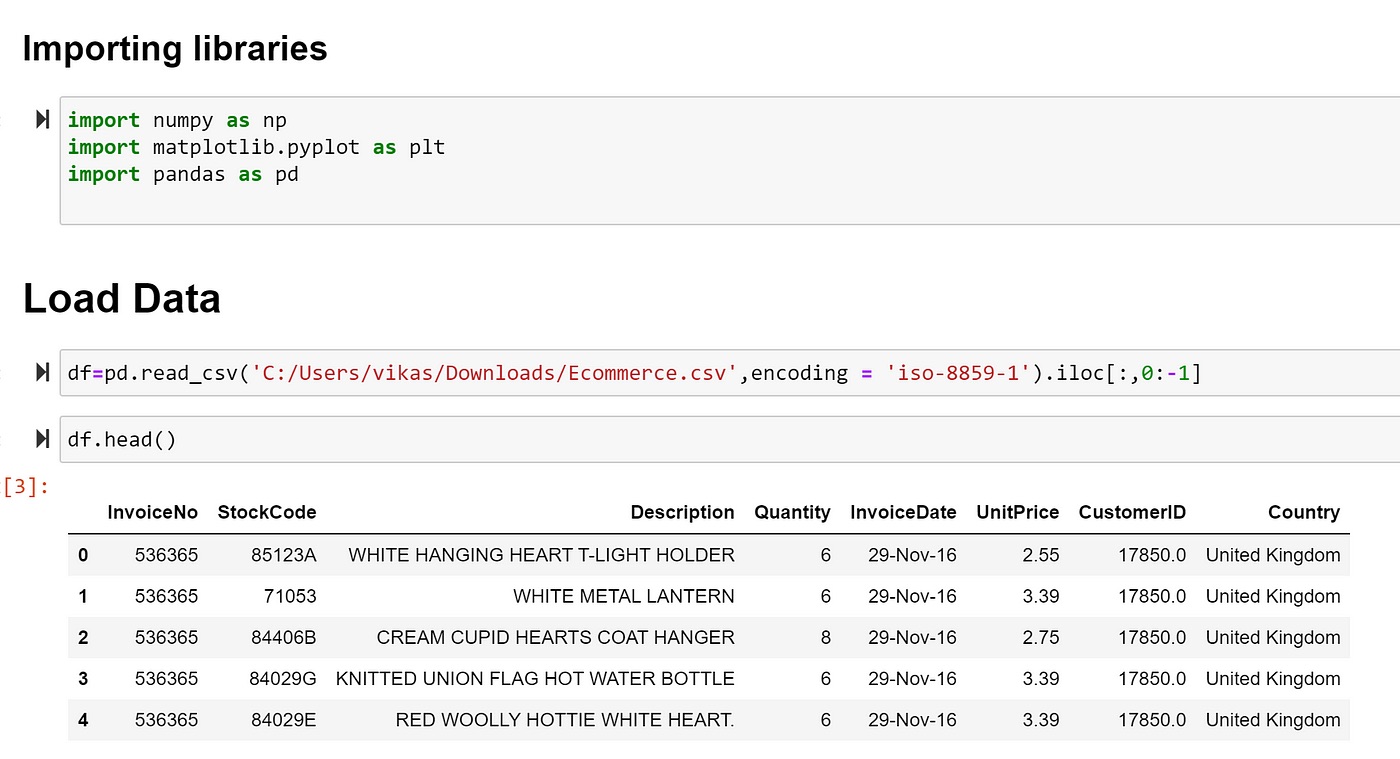
Missing data can be a challenge in any analysis or research project. Whether it’s due to measurement errors, survey non-response, or other reasons, dealing with missing data is an important task to ensure the accuracy and reliability of your findings. In this article, we will explore various techniques and strategies to effectively deal with missing data. From imputation methods to pattern analysis, we will provide you with the tools and insights you need to handle missing data like a pro. So, whether you’re a researcher, analyst, or simply someone who wants to make sense of incomplete information, read on to discover how to tackle missing data and unlock the full potential of your analysis.
Inside This Article
- What is Missing Data?
- Types of Missing Data
- Challenges in Dealing with Missing Data
- Strategies for Handling Missing Data
- Conclusion
- FAQs
What is Missing Data?
Missing data refers to the absence or incomplete information in a dataset. It occurs when data points or values are not recorded, are lost, or are not available for certain variables or observations. This can happen due to various reasons, such as human error, technical issues, survey non-response, or data corruption.
Missing data can have a significant impact on the accuracy and validity of data analysis. It may lead to biased results, reduce statistical power, and affect the generalizability of findings. Therefore, it is crucial to understand the nature of missing data and how it should be handled in data analysis.
Missing data can manifest in different forms. It can be completely missing, where an entire variable is missing for certain observations. It can also be partially missing, where some values within a variable are missing for specific observations. Additionally, missing data can be categorized as missing completely at random (MCAR), missing at random (MAR), or missing not at random (MNAR) based on the underlying mechanism causing the missingness.
Dealing with missing data requires careful consideration and appropriate strategies to minimize its potential impact. It involves understanding the patterns and reasons for missingness, imputing or estimating missing values, and assessing the potential biases introduced by missing data.
Now that we have a basic understanding of missing data, let’s explore the different types of missing data in the next section.
Types of Missing Data
When dealing with missing data, it is essential to understand the different types of missing data that can occur. Here are some common types:
1. Missing Completely at Random (MCAR): This occurs when the missingness has no relationship to either observed or unobserved data. In other words, the missing values are randomly distributed across the dataset.
2. Missing at Random (MAR): In this case, the probability of missingness depends on observed data but not on unobserved data. The missingness is related to the available information in the dataset.
3. Missing Not at Random (MNAR): MNAR refers to a situation where the missingness is dependent on the value of the missing data itself or other unobserved factors. This type of missing data is the most challenging to handle, as the missingness is not random and can introduce bias in the analysis.
4. Single Imputation: Single imputation is a method of filling in missing values with a single estimated value. This approach assumes that the imputed values are the actual values, which can lead to biased estimates and an underestimation of parameter uncertainty.
5. Multiple Imputation: Multiple imputation involves creating multiple plausible values to replace the missing data. Multiple imputation takes into account the uncertainty associated with the imputed values and produces unbiased estimates when correctly implemented.
6. Imputation by Mean: This method replaces missing values with the mean value of the observed data for that variable. It is a simple and commonly used imputation technique but may lead to an underestimation of variance.
7. Imputation by Regression: Regression imputation uses regression models to predict missing values based on the observed data. This method can provide more accurate imputations by taking into account the relationships between variables.
8. Hot Deck Imputation: Hot deck imputation involves borrowing a value from a similar non-missing case to fill in the missing value. It is a non-random imputation method that preserves the relationships between the variables in the dataset.
Understanding the types of missing data can guide you in selecting appropriate strategies for handling missing values in your dataset. Depending on the type and extent of missingness, different imputation methods or statistical techniques may be employed to minimize bias and maintain the integrity of your analysis.
Challenges in Dealing with Missing Data
Missing data poses significant challenges when it comes to data analysis. It can impact the validity and reliability of statistical inferences, making it crucial to address these challenges properly. Here are some of the key challenges in dealing with missing data:
1. Bias: The presence of missing data can introduce bias into the analysis. When data is missing in a non-random manner, it can lead to biased estimates and affect the accuracy of the results. It is important to identify the missing data mechanism and adjust for it to reduce bias.
2. Reduced sample size: Missing data can result in a reduced sample size, leading to decreased statistical power. With a smaller sample, it becomes more challenging to detect meaningful relationships or significant differences in the data. Researchers need to carefully consider the impact of missing data on sample size calculations and power analysis.
3. Loss of information: When data is missing, valuable information is lost. This loss of information can lead to decreased precision in estimations and potential loss of insights. It becomes crucial to handle missing data effectively to minimize the loss of valuable information.
4. Imputation challenges: Imputation is the process of replacing missing values with plausible estimates. However, imputing missing data comes with its own challenges. The imputation method used should be appropriate for the missing data mechanism, and the imputed values should reflect the uncertainty associated with the missing data accurately.
5. Missing data patterns: Different patterns of missing data can present unique challenges. For example, missing values may be dependent on other variables, leading to additional complexities in the analysis. Understanding and identifying the patterns of missing data is essential to make informed decisions about handling it.
6. Potential for introducing errors: When dealing with missing data, there is a risk of introducing errors if the missing data is not handled properly. Errors can occur during imputation, analysis, or interpretation of results. Careful consideration and application of appropriate missing data techniques are necessary to minimize the potential for errors.
7. Time and resource constraints: Dealing with missing data can be time-consuming and resource-intensive. It requires careful planning, data exploration, and implementation of suitable techniques for handling missing data. Researchers may need to invest additional time and resources to ensure the validity and reliability of their analyses.
Addressing these challenges effectively is essential to ensure the integrity and accuracy of data analysis. By employing appropriate strategies and techniques, researchers can mitigate the impact of missing data and obtain reliable insights from their datasets.
Strategies for Handling Missing Data
Dealing with missing data is a common challenge encountered when analyzing datasets. Fortunately, there are various strategies that can be employed to address this issue. Let’s explore some effective strategies for handling missing data:
- Deletion: This strategy involves removing any observations or variables that contain missing data. There are two main ways to implement deletion: listwise deletion and pairwise deletion. Listwise deletion removes entire cases with missing data, while pairwise deletion retains cases with missing data by using available information in the analysis.
- Imputation: Imputation is the process of estimating missing values based on the observed data. There are different methods for imputation, such as mean imputation, where the missing values are replaced with the mean of the available data for that variable. Other methods include using regression models or nearest neighbor algorithms to impute missing values.
- Indicator variables: This strategy involves creating new variables to indicate whether a certain value is missing. These indicator variables can be included in the analysis to account for the missingness and provide additional information during the analysis.
- Model-based methods: Model-based methods involve incorporating missing data mechanisms into statistical models. These methods enable the estimation of missing values based on the relationships between variables in the dataset. Some examples of model-based methods include maximum likelihood estimation and multiple imputation.
- Domain-specific knowledge: In some cases, domain-specific knowledge can be leveraged to address missing data. For example, if the missing data is related to a certain type of measurement, experts in the field may be able to provide insights or recommend appropriate imputation methods.
- Multiple imputation: Multiple imputation is a strategy that involves creating multiple plausible imputations for missing values. By generating multiple imputations, the uncertainty associated with the missing data can be accounted for in the analysis. This strategy is especially useful when the missing data is not completely at random.
It is important to carefully consider the appropriate strategy for handling missing data based on the specific dataset and research question at hand. Each strategy has its own advantages and limitations, so weighing the pros and cons is crucial in order to make informed decisions. By employing these strategies, researchers and analysts can minimize the impact of missing data on their analyses and obtain reliable and valid results.
Conclusion
Dealing with missing data can be a challenging task, but with the right tools and strategies, it is possible to minimize its impact on your analysis. By understanding the reasons behind missing data and utilizing appropriate techniques such as imputation, you can ensure that your conclusions are accurate and reliable. Remember to assess the missing data patterns and explore the potential biases that could arise from their presence.
Additionally, it is crucial to communicate your approach and findings transparently to your audience, acknowledging the limitations introduced by missing data. By doing so, you can maintain the integrity of your analysis and present a more comprehensive picture of the situation. Embracing a proactive and methodical approach to handling missing data will empower you to make informed decisions and draw meaningful insights from your data sets.
Ultimately, dealing with missing data is an integral part of the data analysis process. It requires careful consideration, effective techniques, and clear communication. By adopting these practices, you can confidently navigate through missing data challenges and harness the power of your data to drive informed decision-making.
“`html
FAQs
Q: What is missing data?
A: Missing data refers to the absence of information or values in a dataset. It can occur due to various reasons, such as measurement errors, survey non-response, or technical issues during data collection.
Q: Why is missing data a problem?
A: Missing data can distort the analysis and interpretation of a dataset. It can lead to biased results, reduced statistical power, and inaccurate conclusions. It is crucial to handle missing data appropriately to ensure the validity and reliability of research findings.
Q: How can missing data be dealt with?
A: There are several methods to handle missing data, including listwise deletion (removing cases with missing values), pairwise deletion (including available cases in analysis), imputation (replacing missing values with estimated values), and advanced techniques like multiple imputation. The choice of method depends on the nature and extent of missing data, as well as the research objectives.
Q: What is imputation?
A: Imputation is a technique used to estimate missing values based on the available information in a dataset. It involves replacing missing values with plausible substitutes to create a complete dataset for analysis. Imputation can be done using simple methods like mean imputation, or more sophisticated techniques like regression imputation or multiple imputation.
Q: How can I minimize missing data in my research?
A: To minimize missing data, it is important to ensure a robust data collection process. This includes using validated and reliable measures, conducting thorough training for data collectors, implementing quality control measures, and addressing any potential issues that may lead to missing data. Additionally, pre-testing surveys and conducting pilot studies can help identify and mitigate data collection problems in advance.
“`