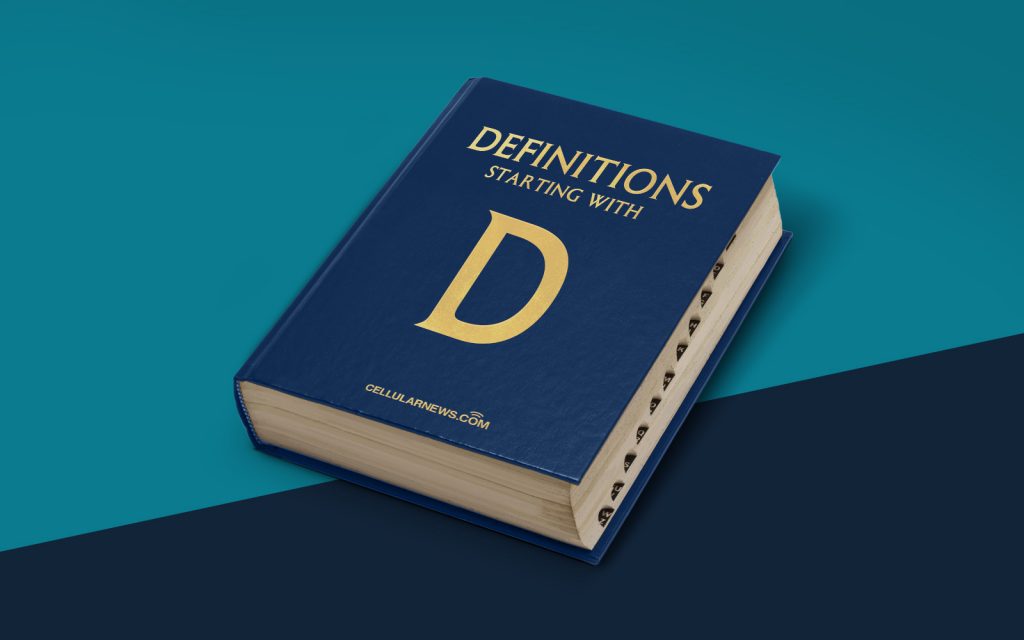
What is Data Quality Assessment (DQA)?
Welcome to the “Definitions” category on our page, where we dive deep into different concepts and explain them in a simple and concise manner. In this article, we will explore the meaning and significance of Data Quality Assessment (DQA), an essential process in the world of data analysis and management.
Data is the backbone of any organization, and its quality plays a crucial role in decision-making, problem-solving, and overall business strategies. However, with the vast amount of data available nowadays, ensuring its accuracy, completeness, and reliability is no easy task. This is where Data Quality Assessment comes into play – it is a systematic evaluation of the quality of data, designed to identify and correct any issues that may affect its usability and reliability.
Key Takeaways:
- Data Quality Assessment (DQA) is the process of evaluating the quality of data to ensure its accuracy, completeness, and reliability.
- DQA helps in identifying and correcting any issues that may affect the usability and reliability of data.
Now, let’s delve deeper into the steps involved in a typical Data Quality Assessment:
- Data Profiling: The first step in DQA is to understand the structure and characteristics of the data. This involves identifying and documenting metadata, such as data types, ranges, patterns, and relationships.
- Data Cleansing: Once the data has been profiled, it is time to clean up any inconsistencies or errors. This may involve removing duplicates, correcting misspellings, or filling in missing values.
- Data Validation: After cleansing, the data needs to be validated to ensure its accuracy and integrity. This involves comparing the data against predefined rules or standards to identify any deviations or anomalies.
- Data Enhancement: In this step, additional data is integrated or appended to improve its completeness and usefulness. This could include enriching the data with external sources or performing data transformations.
- Data Monitoring: Finally, data quality should be an ongoing process. Regular monitoring and reporting are essential to identify any issues that may arise over time and to ensure the continued integrity and usefulness of the data.
By following these steps, organizations can achieve higher data quality, leading to more accurate insights, better decision-making, and improved business outcomes.
So, why is Data Quality Assessment so important? Here are a few key reasons:
- Improved Decision-Making: High-quality data ensures that the decisions made based on this data are reliable and accurate.
- Increased Efficiency: By identifying and fixing data issues, organizations can save valuable time and resources that would otherwise be wasted on incorrect or incomplete data.
- Enhanced Customer Satisfaction: When data quality is high, organizations can provide better products and services to their customers, leading to increased satisfaction and loyalty.
- Compliance and Risk Management: Data Quality Assessment helps organizations comply with regulatory standards and manage risks associated with inaccurate or unreliable data.
In conclusion, Data Quality Assessment (DQA) is a vital process for any organization looking to leverage the power of data. By systematically evaluating the quality of data and taking appropriate corrective actions, organizations can unlock the true potential of their data and drive success in the digital age.