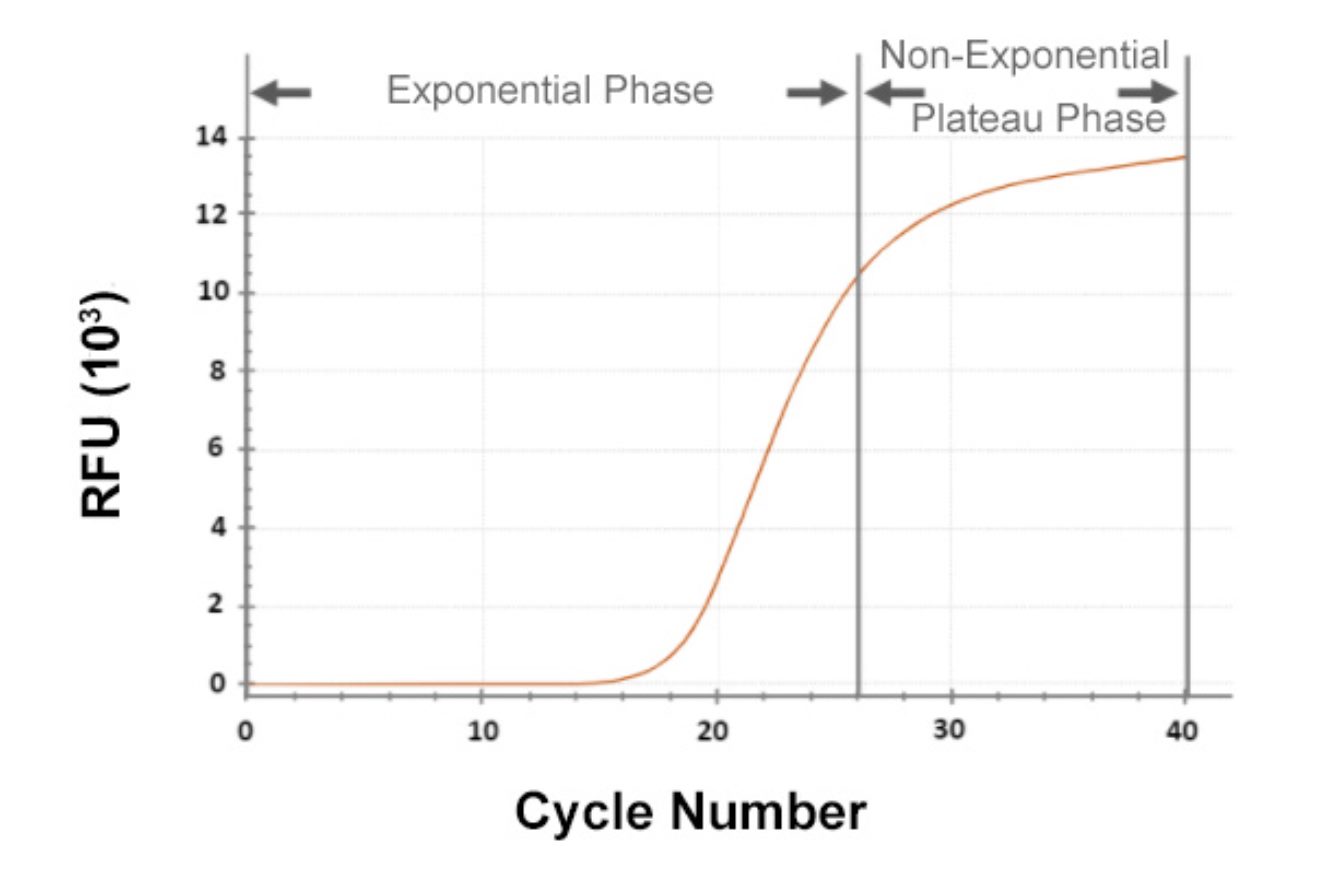
Analyzing qPCR data is a crucial step in molecular biology research, as it provides insights into gene expression levels and enables researchers to draw meaningful conclusions. qPCR, or quantitative polymerase chain reaction, is a powerful technique used to measure the amount of DNA or RNA in a sample. However, interpreting qPCR results can be complex, requiring a thorough understanding of data analysis methods and statistical techniques.
In this article, we will explore the key steps and considerations involved in analyzing qPCR data. We will discuss normalization methods, data quality assessment, statistical analysis, and interpretation of results. Whether you are a seasoned researcher or a beginner in the field, this comprehensive guide will provide you with the knowledge and tools necessary to effectively analyze your qPCR data and draw reliable conclusions from your experiments.
Inside This Article
Overview of qPCR
Quantitative Polymerase Chain Reaction (qPCR) is a powerful molecular biology technique used to measure gene expression levels and quantify DNA or RNA molecules in a sample. It is widely used in research, diagnostics, and applications such as biomarker discovery and gene expression profiling. In qPCR, the amplification of specific DNA or RNA sequences is monitored in real-time, allowing for precise quantification of the target molecules.
The qPCR process starts with the conversion of RNA molecules into complementary DNA (cDNA) using reverse transcription. The cDNA is then amplified using PCR, which involves multiple cycles of denaturation, annealing, and extension of the DNA fragments. During each cycle, the amount of amplified DNA is measured using fluorescent probes or dyes.
The fluorescence intensity is directly proportional to the amount of DNA or RNA molecules present in the sample. By comparing the fluorescence signals of the target gene to housekeeping genes or reference standards, researchers can determine the relative expression levels of the target gene and make comparisons between different samples.
One of the key advantages of qPCR is its sensitivity. It can detect and quantify very low levels of target molecules, making it ideal for studying genes with low expression or for detecting rare genetic variants. Additionally, qPCR provides real-time data, allowing for immediate analysis and interpretation.
To ensure accurate and reliable results, proper experimental design and optimization are crucial. It is important to select appropriate primers and probes that specifically target the desired DNA or RNA sequence and optimize the reaction conditions, such as annealing temperature and primer concentration. The use of control samples and reference genes is also essential to normalize the data and account for experimental variations.
Furthermore, advanced data analysis techniques and software tools are available to analyze qPCR data. These include algorithms for quantification, normalization, and statistical analysis to identify significant differences or changes in gene expression between different samples or experimental conditions.
Pre-processing of qPCR data
Pre-processing of qPCR data is a crucial step in the analysis process. It involves several tasks that help clean and prepare the data for further analysis. These tasks aim to address common issues related to the quality and reliability of the qPCR data.
One of the first steps in pre-processing is the removal of any outliers or erroneous data points that may have occurred during the experimental process. This can be achieved by setting a threshold based on the experimental conditions and removing any data points that fall outside of that range.
Normalization is another important aspect of pre-processing. qPCR data normalization ensures that the expression levels of the target genes are relative to an appropriate reference gene or set of reference genes. This step helps correct for variations in sample preparation and amplification efficiency, allowing for more accurate comparisons between samples.
In addition to normalization, data transformation is often performed to improve the distribution and variability of the data. Common transformations include log transformation, which helps address the skewness in the data and allows for more meaningful statistical analysis.
Background subtraction is another pre-processing step that helps remove any non-specific signals or noise from the qPCR data. This is especially important when working with biological samples that may contain contaminants or background noise that can interfere with the interpretation of the results.
Pre-processing of qPCR data also involves quality control measures to assess the reliability and reproducibility of the data. This includes checking for amplification efficiency, determining the cycle threshold values, and analyzing the melting curve to ensure the specificity of the amplification reaction.
Finally, it is essential to validate the pre-processing steps and assess the impact on the downstream analysis. This can be done by comparing the results before and after pre-processing, evaluating the consistency and biological relevance of the data, and performing additional validation experiments if necessary.
Overall, pre-processing of qPCR data plays a critical role in ensuring the accuracy and reliability of the analysis. By addressing common issues and applying appropriate techniques, researchers can obtain more meaningful and interpretable results from their qPCR experiments.
Statistical analysis of qPCR data
Statistical analysis plays a vital role in interpreting qPCR (quantitative polymerase chain reaction) data accurately. It allows researchers to determine the significance of gene expression changes and evaluate potential differences between experimental conditions. In this section, we will explore some common statistical methods used in qPCR data analysis.
1. T-test: The t-test is a widely used statistical test that assesses whether the means of two groups are significantly different. In qPCR analysis, the t-test can be applied to compare gene expression levels between a control group and an experimental group. It provides valuable insights into the statistical significance of any observed differences.
2. ANOVA: Analysis of Variance (ANOVA) is a statistical method used to compare means of three or more groups. In qPCR data analysis, ANOVA can be employed to assess gene expression variations among multiple experimental conditions or treatment groups. If the ANOVA test suggests a significant difference, further post-hoc tests can be conducted to identify the specific groups that differ from each other.
3. Regression analysis: Regression analysis is a powerful tool to determine the relationship between two variables. In qPCR data analysis, it can be used to establish the correlation between gene expression levels and independent variables such as experimental time points, treatment concentrations, or genetic factors. Regression analysis can provide valuable insights into the patterns and trends of gene expression over time or under different experimental conditions.
4. Principal Component Analysis (PCA): PCA is a dimensionality reduction technique that can be applied to qPCR data to identify underlying patterns and relationships among genes. It helps researchers visualize the similarities or differences between samples and identify key genes responsible for the observed variations. PCA can be particularly useful for exploring complex qPCR datasets and identifying potential outliers or clusters.
5. Normalization: Normalization is an essential step in qPCR data analysis to correct for any technical variations or biases. Several normalization methods, such as the ?Ct method or the housekeeping gene method, can be used to account for differences in sample quantity or quality. Normalization ensures that gene expression measurements are comparable and allows for more reliable statistical analysis.
Conclusion
In conclusion, analyzing qPCR data may seem daunting, but with the right knowledge and tools, it can be a valuable process for understanding gene expression. By following the steps outlined in this article, you can confidently analyze your qPCR data and draw meaningful conclusions.
Remember, accuracy is key in qPCR analysis, so be sure to optimize your primer design, perform proper controls, and validate your results. Take advantage of online resources, software, and statistical methods to streamline your analysis and gain deeper insights from your data.
Keep in mind that qPCR is a dynamic field, and new techniques and advancements are constantly emerging. Stay updated with the latest developments and continue to expand your knowledge to become a proficient qPCR data analyst.
By mastering the art of qPCR data analysis, you will be equipped with a powerful tool for studying gene expression and contributing to scientific discoveries.
FAQs
**Q: What is qPCR data?**
A: qPCR, or quantitative polymerase chain reaction, is a technique used to quantify the amount of DNA or RNA in a DNA sample. It measures the amount of target DNA or RNA before and after amplification using fluorescent probes or dyes.
**Q: Why is analyzing qPCR data important?**
A: Analyzing qPCR data is crucial because it allows researchers to accurately measure gene expression levels, detect genetic variations, and assess the effectiveness of a particular treatment or drug. It provides valuable insights into the molecular dynamics of various biological processes.
**Q: What are the key steps involved in analyzing qPCR data?**
A: The key steps in analyzing qPCR data include pre-processing raw data, normalizing the data, determining the efficiency of the qPCR reaction, calculating relative gene expression levels, and performing statistical analysis to draw meaningful conclusions.
**Q: What are the different methods of data normalization in qPCR analysis?**
A: There are several methods of data normalization in qPCR analysis, including the ?Ct method (comparative Ct method), the ??Ct method (comparative Ct method with a control sample), and the efficiency-corrected method (using reference genes with known stable expression levels).
**Q: What are the common challenges faced in qPCR data analysis?**
A: Some common challenges in qPCR data analysis include selecting appropriate reference genes for normalization, determining the efficiency of the qPCR reaction accurately, avoiding technical variations in pipetting and sample handling, and dealing with outliers or inconsistent data points.