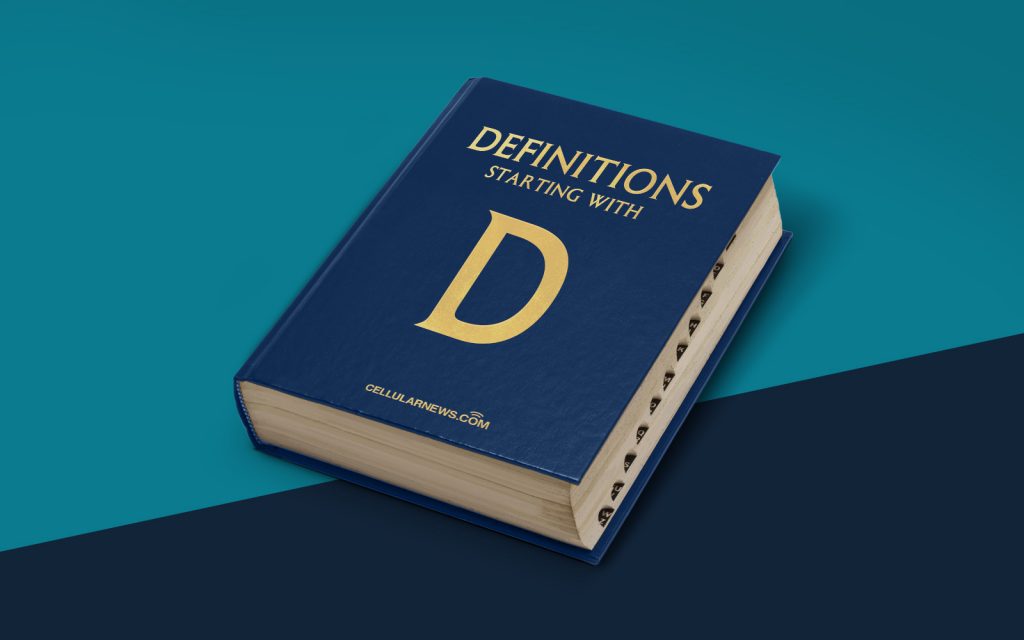
What is Data Cholesterol?
Welcome to our “Definitions” category, where we explain complex concepts in a simple and digestible manner. Today, we’ll be shedding light on the intriguing term, “Data Cholesterol.”
Data Cholesterol refers to the accumulation of irrelevant, outdated, or inaccurate data within a dataset or database. It is similar to the concept of “bad cholesterol” in our bodies, which can lead to negative health consequences. In the same way, Data Cholesterol can have adverse effects on data quality and hinder decision-making processes.
Key Takeaways:
- Data Cholesterol refers to the accumulation of irrelevant, outdated, or inaccurate data.
- High levels of Data Cholesterol can negatively impact data quality and decision-making processes.
In today’s digital age, organizations generate and accumulate vast amounts of data. However, not all data is created equal. Just like we maintain a healthy lifestyle to minimize the risk of high cholesterol, it’s crucial for organizations to proactively manage and monitor their data quality to reduce the impact of Data Cholesterol.
But you may wonder, what causes Data Cholesterol to accumulate?
The culprits behind the development of Data Cholesterol can be:
- Inaccurate Data Entry: Mistakes made during the data entry process can introduce inaccuracies into the dataset, contributing to Data Cholesterol.
- Outdated Data: Time-sensitive data that is not regularly updated loses its relevance and can be considered as Data Cholesterol.
- Redundant Data: Duplicated or redundant data within a dataset serves no purpose and only contributes to the accumulation of Data Cholesterol.
- Irrelevant Data: Data that is no longer relevant to the organization’s core objectives or business processes can be classified as Data Cholesterol.
Data Cholesterol can hinder data analytics efforts, as decision-makers will have to sift through a sea of irrelevant or inaccurate information. This can lead to misguided insights, inefficiencies, and missed opportunities for growth and optimization.
So, how can organizations prevent or lower their Data Cholesterol levels?
Here are a few effective strategies to combat Data Cholesterol:
- Data Cleansing: Regularly review, identify, and remove outdated or irrelevant data to maintain a clean and healthy dataset.
- Data Governance: Implement robust data governance frameworks to ensure data quality standards are met and maintained.
- Data Validation: Establish validation processes to verify the accuracy and integrity of data during the entry phase.
- Data Integration: Integrate data from various sources to create a unified and consistent dataset, reducing the chances of redundancies and inaccuracies.
- Regular Audits: Conduct periodic audits to identify and address any instances of Data Cholesterol proactively.
By adopting these strategies, organizations can reduce their Data Cholesterol levels, improve data quality, and make more informed data-driven decisions.
Remember, just as we take care of our bodies to maintain optimal health, it’s essential for organizations to prioritize data quality management and keep their data free from the ill effects of Data Cholesterol.
We hope this article has helped demystify the concept of Data Cholesterol. Stay tuned for more informative content in our “Definitions” category!