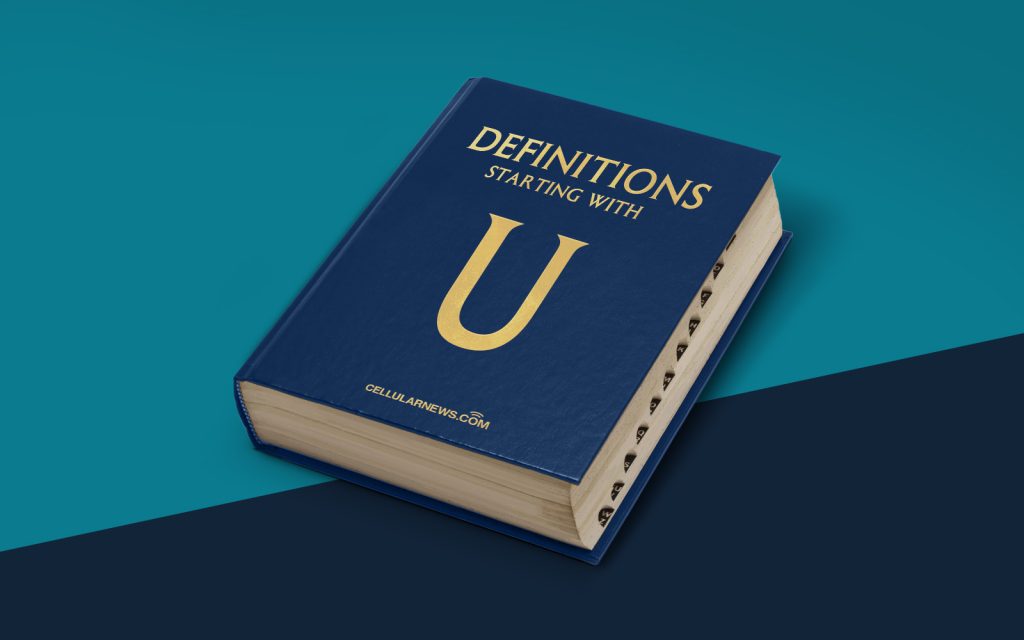
Unleashing the Power of Unlabeled Data
Welcome to the world of data! If you are familiar with the term “labeled data,” you may find yourself wondering, “What is unlabeled data?” In the realm of data science and machine learning, unlabeled data plays a crucial role in unlocking valuable insights and enhancing predictive models. In this article, we’ll explore the concept of unlabeled data, its significance, and how it can be utilized to gain a competitive advantage.
Key Takeaways
- Unlabeled data refers to a dataset where the outcomes or categories are not predetermined or identified.
- Unlabeled data is valuable because it provides an opportunity for unsupervised learning algorithms to identify patterns and structures in data, leading to new insights and opportunities.
Unraveling the Mystery of Unlabeled Data
In the realm of data analysis, researchers are often faced with two types of datasets: labeled and unlabeled. Labeled data, as the name suggests, has already been categorized or tagged with specific outcomes. This pre-existing labeling can be done manually by humans or through algorithms. On the other hand, unlabeled data refers to a dataset where the outcomes or categories are not predetermined or identified.
Unlabeled data represents a vast collection of raw information that holds immense potential. By leveraging unlabeled data, data scientists can tap into uncharted territories, uncover hidden patterns, and gain insightful knowledge. While labeled data traditionally takes center stage in supervised learning, unlabeled data opens the door to the world of unsupervised learning algorithms, where patterns and structures can be discovered without predefined labels or categories.
Unlabeled data offers numerous benefits and applications in the world of data science and machine learning:
1. Unsupervised Learning and Pattern Discovery
Unlabeled data is a goldmine for unsupervised learning algorithms. Instead of relying on predefined labels, unsupervised learning algorithms delve into the raw unstructured data to identify patterns, structures, and relationships. By recognizing patterns in the data, these algorithms can classify the data in a meaningful way, grouping together similar instances and uncovering hidden insights.
Unsupervised learning algorithms utilize various techniques such as clustering, association rule learning, and dimensionality reduction to make sense of unlabeled data. Through these techniques, patterns and structures can be discovered, providing valuable insights that may have otherwise gone unnoticed.
2. Anomaly Detection and Outlier Identification
Unlabeled data is instrumental in anomaly detection and outlier identification. Anomalies or outliers are data points that deviate significantly from the normal or expected behavior. In many real-world scenarios, anomalies can represent critical events, fraud attempts, or faulty data points, making their detection crucial.
Unsupervised learning algorithms can utilize the power of unlabeled data to establish an understanding of what is considered normal or typical behavior. By training on unlabeled data, these algorithms can learn to identify deviations from the norm, uncovering anomalies and outliers that may signify exceptional events or unusual occurrences.
Unlabeled data presents an opportunity for data scientists and organizations to unlock the hidden potential of raw information. By leveraging unsupervised learning techniques, such as pattern discovery and anomaly detection, valuable insights and competitive advantages can be gained. So, next time you come across unlabeled data, remember the untapped possibilities it holds.