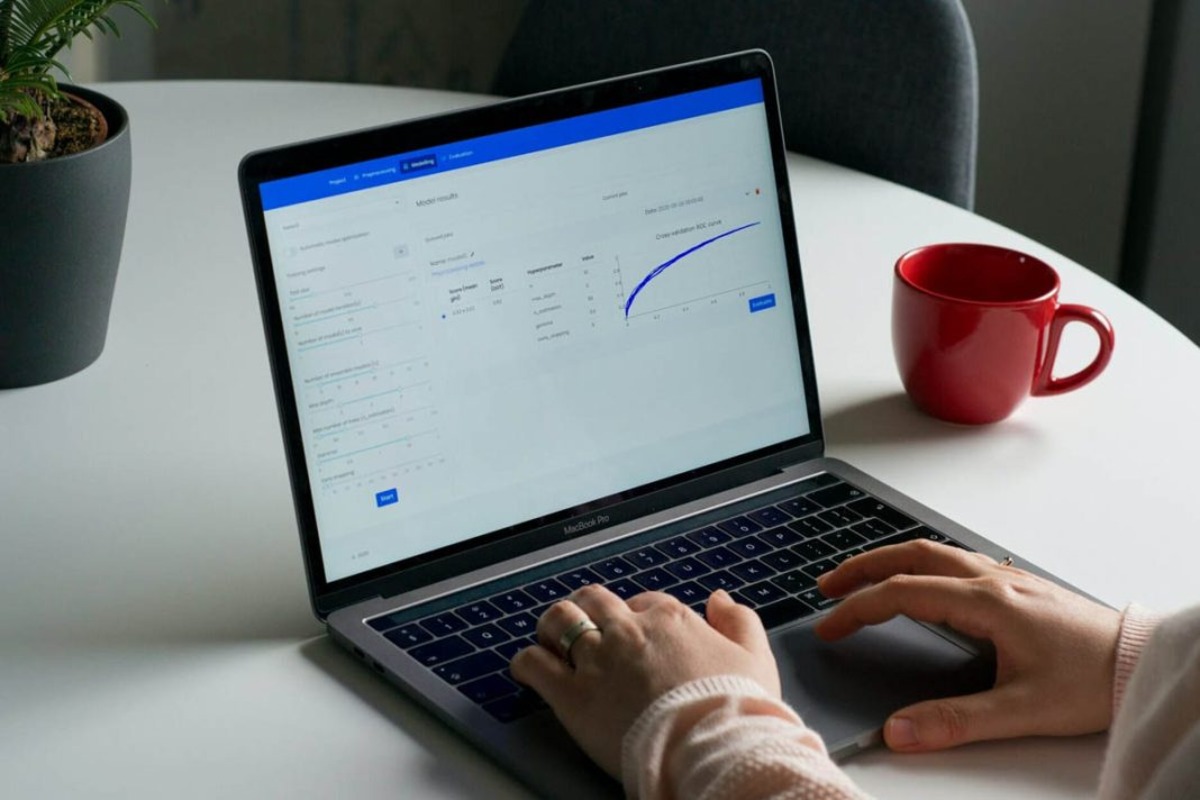
Understanding the distribution of data is of vital importance in various fields, including statistics, data analysis, and machine learning. The distribution of data provides insights into the patterns, trends, and characteristics of a dataset. It helps to identify outliers, determine the central tendency, and measure the variability of the data. Describing the distribution of data involves analyzing its shape, spread, and skewness.
By understanding the distribution of data, we can make informed decisions, draw meaningful conclusions, and develop robust models. This knowledge allows us to gain a deeper understanding of the underlying phenomenon and aids in making accurate predictions. In this article, we will explore different measures and techniques to describe the distribution of data, empowering you with the tools necessary to effectively analyze and interpret datasets.
Inside This Article
- What is distribution of data?
- Describing the Shape of a Distribution
- Measures of central tendency
- Measures of Dispersion
- Conclusion
- FAQs
What is distribution of data?
When we talk about the distribution of data, we are referring to how the values in a dataset are spread or distributed. In other words, it describes the pattern or arrangement of data points.
A distribution can reveal important information about a dataset, such as typical values, extremes, and the variation between values. It provides insights into the overall characteristics and behavior of the data.
In statistical terms, a distribution represents the frequencies or probabilities of different values occurring within the dataset. It allows us to understand the likelihood of specific values occurring and the range within which most values fall.
Understanding the distribution of data is crucial for various statistical analyses, as it helps in making accurate predictions, identifying outliers, and making informed decisions based on the data at hand.
Now that we have a basic understanding of what a distribution of data is, let’s explore how we can describe the shape of a distribution.
Describing the Shape of a Distribution
When analyzing data sets, it’s crucial to understand the shape of the distribution. By examining the shape, we gain insights into the underlying patterns and characteristics of the data. Describing the shape of a distribution involves identifying whether it is symmetrical, skewed, or has multiple peaks.
1. Symmetrical Distribution: A symmetrical distribution, also known as a bell-shaped or normal distribution, is characterized by equal frequencies on both sides of the center. The data points are evenly distributed, creating a perfect balance. The mean, median, and mode are all approximately equal in a symmetrical distribution.
2. Skewed Distribution: In a skewed distribution, the data is not evenly distributed. It means that one tail of the distribution is longer or stretches further than the other. There are two types of skewed distributions:
- Positively Skewed (Right Skewed): In a positively skewed distribution, the tail extends to the right. This indicates that the majority of the data points are concentrated on the left side. The mean is pulled towards the higher values, while the median and mode are lower.
- Negatively Skewed (Left Skewed): In a negatively skewed distribution, the tail extends to the left. This implies that the majority of the data points are concentrated on the right side. The mean is pulled towards the lower values, while the median and mode are higher.
3. Multi-modal Distribution: A multi-modal distribution exhibits multiple peaks or modes, indicating the presence of different groups within the data. Each peak represents a distinct set of data points. This type of distribution is often observed in complex data sets or those consisting of different subgroups.
Describing the shape of a distribution aids in understanding the central tendency and variability of the data. It provides valuable insights for further analysis and decision-making. Keep in mind that these are general descriptions, and there can be variations within each type of distribution.
Measures of central tendency
When describing the distribution of data, one important aspect to consider is the central tendency. Measures of central tendency are statistics that indicate the center or average value of a dataset. They provide a single value that represents the typical or central value of the data.
There are three commonly used measures of central tendency: the mean, the median, and the mode. Let’s take a closer look at each of these measures:
- Mean: The mean is the most commonly used measure of central tendency. It is calculated by summing up all the values in the dataset and dividing it by the total number of values. The mean is sensitive to extreme values, so if there are outliers in the dataset, it can significantly affect the mean.
- Median: The median is the middle value of a dataset when it is arranged in ascending order. If the dataset has an odd number of values, the median is the value exactly in the middle. If the dataset has an even number of values, the median is the average of the two middle values. Unlike the mean, the median is not influenced by extreme values.
- Mode: The mode is the value that appears most frequently in a dataset. It represents the value that occurs with the highest frequency. A dataset can have multiple modes if two or more values occur with the same highest frequency.
Each measure of central tendency provides different insights into the distribution of data. The mean represents the average value, the median represents the middle value, and the mode represents the most frequent value. The choice of which measure to use depends on the specific characteristics and goals of the analysis.
It’s important to note that measures of central tendency can be affected by skewed distributions or outliers. Skewed distributions have a longer tail on one side, pulling the mean in that direction. Outliers are extreme values that can heavily influence the mean but have little effect on the median or mode.
By understanding and utilizing measures of central tendency, analysts and researchers can gain valuable insights about the typical or central value of the data distribution. These measures provide a summary of the data, allowing for easier interpretation and comparison of datasets.
Measures of Dispersion
Measures of dispersion — also known as measures of variability or spread — are statistical indicators that describe the extent to which data points in a dataset are spread out. While measures of central tendency, such as mean and median, provide a summary of the central or average value of the data, measures of dispersion give insights into the variability or dispersion of the data points around that central value.
Measures of dispersion are crucial in understanding the shape and characteristics of a dataset. They help us determine the consistency or spread of the data and give a sense of the level of precision or accuracy within the dataset. These measures are widely used in various fields such as finance, economics, research, and data analysis.
There are several common measures of dispersion that are frequently used:
- Range: The range measures the span between the lowest and highest values in a dataset. It provides a simple and straightforward understanding of the spread of the data but can be sensitive to extreme values.
- Variance: The variance measures the average squared deviation from the mean. It quantifies the amount of dispersion by considering each data point’s deviation from the mean, giving more weight to extreme deviations.
- Standard Deviation: The standard deviation is the square root of the variance. It provides a measure of dispersion that is in the same unit as the original data, making it easier to interpret and compare across datasets.
- Mean Absolute Deviation: The mean absolute deviation (MAD) calculates the average absolute difference between each data point and the mean, providing a measure of dispersion that is not influenced by extreme values.
Each of these measures has its strengths and weaknesses and is used in different scenarios based on the nature and characteristics of the data. For example, the range is simple to calculate but can be affected by outliers, while the variance and standard deviation are more sensitive to extreme values, making them suitable for datasets with normally distributed data.
Understanding and utilizing measures of dispersion helps in assessing the spread of data, identifying outliers, and evaluating the overall variability within a dataset. By considering both measures of central tendency and measures of dispersion, we gain a comprehensive view of the distribution of data, providing valuable insights for data analysis and decision-making.
Conclusion
In conclusion, understanding the distribution of data is crucial for making informed decisions and drawing accurate conclusions in various fields such as statistics, data analysis, and machine learning. By examining the shape, center, and spread of a dataset, we can gain valuable insights about its characteristics and make predictions with greater confidence.
Whether it’s analyzing customer purchase patterns, predicting market trends, or identifying outliers in experimental data, describing the distribution helps us understand the underlying patterns and make data-driven decisions.
Through techniques such as histogram visualization, measures of central tendency, and measures of dispersion, we can effectively describe the distribution of data. By applying these concepts and techniques, we can transform raw data into valuable insights that drive decision-making and problem-solving processes.
So, the next time you encounter a dataset, remember the importance of describing its distribution and utilize the appropriate tools and methods to gain a deeper understanding of the data’s characteristics.
FAQs
1. What is the distribution of data?
The distribution of data refers to the way in which data points are spread or distributed across a dataset. It provides insights into the patterns and characteristics of the data, helping us understand its central tendency, variability, and shape.
2. Why is it important to describe the distribution of data?
Describing the distribution of data is important because it allows us to draw meaningful conclusions and make informed decisions. By identifying the central tendency, such as mean or median, we can understand the typical value in a dataset. Additionally, understanding the variability helps us assess the range of values and the level of dispersion. Furthermore, analyzing the shape of the distribution helps us identify any skewness or outliers, giving us a better understanding of the underlying patterns.
3. What are the common measures used to describe the distribution of data?
There are several common measures used to describe the distribution of data. These include the mean, median, mode, range, variance, standard deviation, and percentiles. Each of these measures provides different insights into the distribution and helps us understand its characteristics more comprehensively.
4. How can we visually represent the distribution of data?
There are various ways to visually represent the distribution of data. One common method is using histograms, which display the frequency or count of values within different intervals or bins. Box plots are another popular choice, showing the quartiles, median, and any outliers present in the data. Additionally, line graphs, scatter plots, and bar charts can provide visual representations of the distribution depending on the nature of the data and the insights we seek.
5. What are some common types of distributions?
There are many types of distributions that can be observed in data. Some common types include the normal distribution, which is symmetric and bell-shaped, often observed in many natural phenomena. The uniform distribution is another type where all values have equal probabilities, forming a flat and rectangular shape. Other distributions include the exponential distribution, skewed distributions, and bimodal distributions, each with its own unique characteristics.