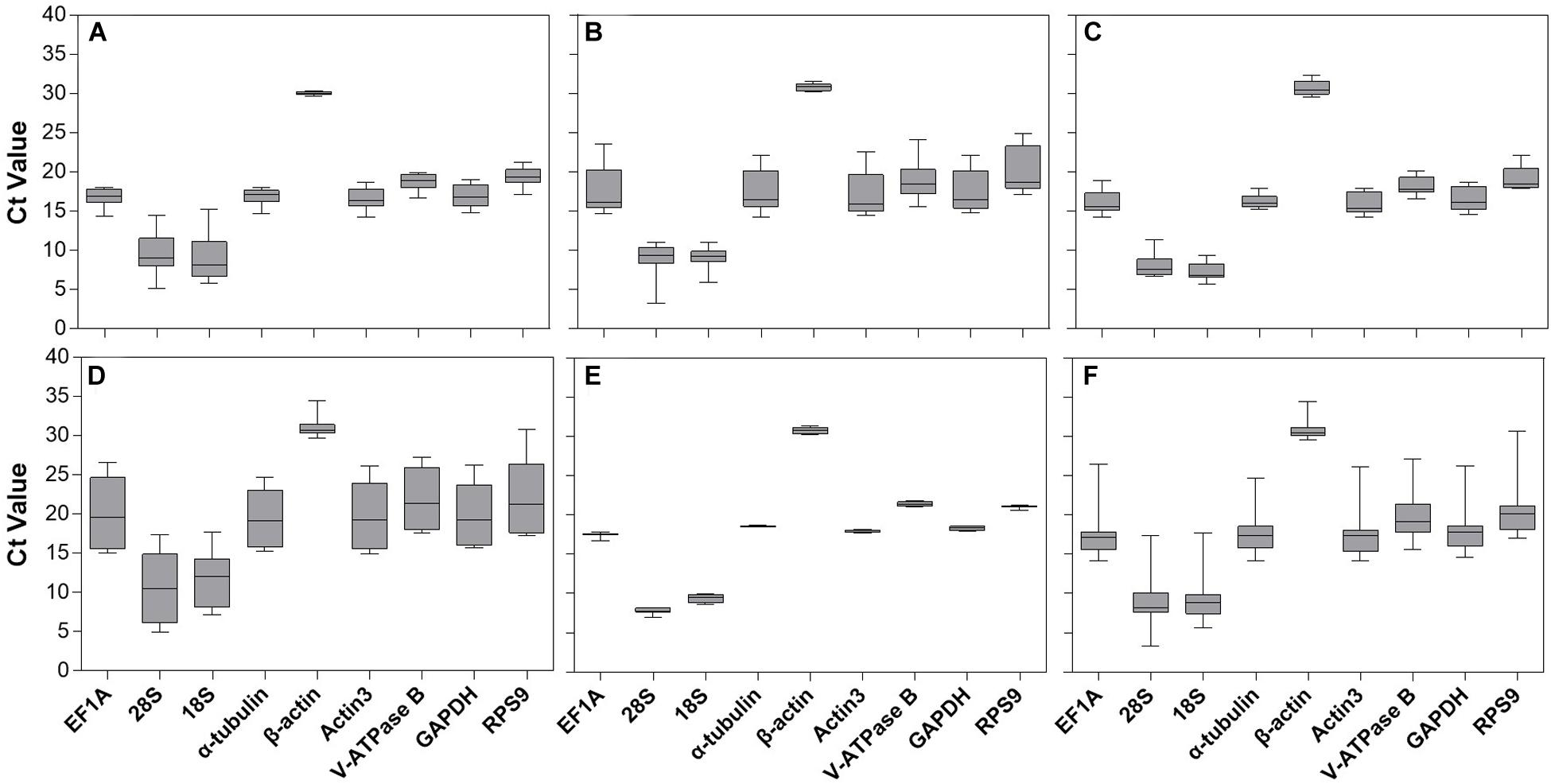
Cellectis’ CAR-T cell therapy is revolutionizing the field of cancer treatment. With promising results in clinical trials and the potential to provide a more targeted and effective approach to fighting cancer, CAR-T cell therapy has captured the attention of researchers, physicians, and patients alike.
In this article, we will delve into the science behind CAR-T cell therapy, explore its application in treating different types of cancer, and highlight some of the ongoing research and developments in this exciting field. So, if you’re curious about how CAR-T cell therapy works and how it is changing the landscape of cancer treatment, you’ve come to the right place.
Inside This Article
- Overview
- Selecting a Reference Gene
- Data Normalization Methods
- Calculation of Relative Expression
- Conclusion
- FAQs
Overview
When it comes to quantitative real-time PCR (qPCR), accurate data normalization is crucial to ensure reliable results and meaningful interpretation. Normalization is the process of adjusting the gene expression values in qPCR experiments to account for differences in sample input amounts, RNA quality, and other technical variations. It is essential for comparing gene expression levels between samples and across different experimental conditions.
Normalized qPCR data allows researchers to account for variations in the RNA starting material, reverse transcription efficiency, and amplification efficiency. By normalizing the data, it becomes possible to accurately compare the expression levels of the gene of interest across different samples, treatments, or time points.
There are several approaches to data normalization in qPCR, including the selection of an appropriate reference gene, the use of housekeeping genes, or the application of normalization algorithms. Each approach has its advantages and considerations, and the choice depends on the experimental setup, gene stability, and other factors.
In this article, we will explore the importance of selecting a reference gene, discuss different data normalization methods, and outline the calculation of relative expression values. By understanding these concepts, you will be better equipped to normalize your qPCR data accurately and derive meaningful insights from your experimental results.
Selecting a Reference Gene
When performing quantitative PCR (qPCR) experiments, selecting a suitable reference gene is crucial for accurate data normalization. A reference gene, also known as a housekeeping gene, is used as a control to account for variations in sample preparation, RNA quality, and amplification efficiency. Here are some important considerations when selecting a reference gene:
1. Stability: The reference gene should have stable expression across different experimental conditions. It should not be affected by the treatment or experimental variables being studied. Commonly used reference genes include GAPDH, ?-actin, and 18S rRNA.
2. Expression level: The reference gene should have a moderate expression level comparable to the target gene. If the reference gene is highly expressed, it may saturate the PCR amplification, leading to inaccurate quantification. On the other hand, if the reference gene is lowly expressed, it may cause amplification biases.
3. Validation: It is essential to validate the stability of the reference gene under the specific experimental conditions. This can be done by performing expression analysis across different samples or cell lines, and using statistical algorithms such as geNorm or NormFinder to determine the most stable reference gene.
4. Tissue specificity: Some reference genes may exhibit tissue-specific expression patterns. It is important to choose a reference gene that is constitutively expressed across different tissues, especially if your experiments involve multiple tissues or organs.
5. Literature review: Before finalizing the reference gene, it is advisable to consult the existing literature and check for commonly used reference genes in similar experimental setups. This can provide valuable insights and increase the robustness of your analysis.
By carefully selecting a suitable reference gene, you can ensure accurate and reliable normalization of your qPCR data. This step is critical for obtaining meaningful and interpretable results in gene expression studies.
Data Normalization Methods
Data normalization is a crucial step in analyzing qPCR data to ensure accurate and reliable results. It helps correct for variations in sample input and technical factors during the experimental process. There are several data normalization methods commonly used in qPCR data analysis. Let’s explore them below:
1. Relative Gene Expression: This method involves calculating the relative expression of the target gene compared to a reference gene. The chosen reference gene should have stable expression levels across all samples. The expression level of the target gene is then normalized by dividing it by the expression level of the reference gene. This method provides a relative measure of the target gene expression.
2. Delta-Delta Ct: The delta-delta Ct method, also known as the 2^(-??Ct) method, compares the difference in threshold cycle (Ct) values between the target and reference genes. It involves calculating the delta-Ct value by subtracting the Ct value of the reference gene from the Ct value of the target gene. The delta-delta Ct value is then calculated by subtracting the delta-Ct value of the control group from the delta-Ct value of the treatment group. This method provides a fold change in gene expression.
3. External Reference: In this method, an external reference is used for data normalization. The external reference can be a known stable gene or a synthetic reference gene. The expression level of the target gene is divided by the expression level of the external reference gene to obtain the normalized data.
4. Reference Pool: The reference pool method involves creating a pool of reference genes by combining multiple suitable reference genes. The average expression level of these reference genes is used to normalize the expression level of the target gene. This method provides a robust normalization approach by reducing the impact of variations in individual reference gene expression.
5. Efficiency Correction: This method corrects for variations in PCR efficiency between the target and reference genes. It involves using a formula that incorporates the PCR efficiency of both the target and reference genes to normalize the data. This method ensures more accurate normalization by taking into account differences in amplification efficiency.
6. Global Mean Normalization: In this method, the mean Ct value of all the genes in a sample is calculated, and the Ct values of individual genes are normalized by subtracting the global mean Ct value. This method accounts for the overall differences in gene expression levels between samples.
It is important to note that the choice of data normalization method should be based on careful evaluation of the experimental conditions and the characteristics of the genes being analyzed. Considerations such as stability of reference genes, sample variability, and amplification efficiency need to be taken into account to ensure accurate data normalization and reliable results.
Calculation of Relative Expression
Once you have selected a reference gene and obtained the raw qPCR data for your target gene and reference gene, the next step is to calculate the relative expression of your target gene.
The relative expression is a measure of how much the expression of your target gene changes relative to the expression of the reference gene. It allows you to compare the gene expression levels between different samples or conditions.
There are several methods available for calculating the relative expression, but one commonly used method is the delta-delta Ct (??Ct) method.
In the ??Ct method, the first step is to calculate the Ct values for your target gene and reference gene for each sample. The Ct value, or cycle threshold, is the cycle number at which the fluorescence signal crosses a predefined threshold.
The next step is to calculate the ?Ct, which is the difference in Ct values between the target gene and the reference gene for each sample. This can be done using the formula: ?Ct = Ct(target gene) – Ct(reference gene).
The final step is to calculate the ??Ct, which is the difference in ?Ct values between your experimental group and a reference group. The reference group is typically a control group or a group with a known expression level of your target gene.
Once you have the ??Ct value, you can calculate the relative expression using the formula: Relative Expression = 2^(-??Ct).
This formula calculates the fold change in gene expression between the experimental group and the reference group. A value greater than 1 indicates upregulation or increased expression, while a value less than 1 indicates downregulation or decreased expression.
The relative expression can be further analyzed using statistical methods to determine the significance of the differences in gene expression between groups.
It is important to note that the ??Ct method assumes that the PCR efficiency is the same for both the target gene and the reference gene. If there are significant differences in PCR efficiency, it can lead to inaccurate results. In such cases, it is recommended to use alternative methods like the efficiency-corrected ??Ct method.
Overall, the calculation of relative expression is a crucial step in qPCR data analysis. It provides valuable insights into the changes in gene expression and allows for meaningful comparisons between samples or conditions.
Conclusion
In conclusion, normalizing qPCR data is a crucial step in ensuring accurate and reliable results in gene expression analysis. By applying appropriate normalization methods, researchers can account for variations in sample preparation, reverse transcription efficiency, and other technical factors that may introduce bias in the qPCR data.
Normalization methods like ??Ct and other housekeeping genes normalization approaches help to mitigate the impact of technical variability, allowing for more meaningful comparisons across samples and experimental conditions. It is essential to choose a normalization strategy that suits the specific experimental design and research question.
Remember, a thorough understanding of the principles and techniques involved in qPCR normalization is essential for accurate interpretation of gene expression data. By following best practices, researchers can ensure that their results are reliable and reproducible, ultimately contributing to advancements in molecular biology and related fields.
FAQs
Q: What is qPCR data normalization?
A: qPCR data normalization is the process of accounting for variation in gene expression levels across different samples or conditions. It helps to remove technical biases and normalize the data so that meaningful comparisons can be made between samples.
Q: Why is normalization important in qPCR?
A: Normalization is important in qPCR because it ensures that any differences in gene expression levels are not due to experimental variations or technical factors. It allows for accurate comparisons between samples and helps to identify true differences in gene expression.
Q: What are the different methods of normalizing qPCR data?
A: There are several methods of normalizing qPCR data, including the use of reference genes, geometric mean normalization, total counts normalization, and advanced statistical methods such as the delta-delta Ct method.
Q: What are reference genes and how are they used for normalization in qPCR?
A: Reference genes are genes that are believed to have stable expression levels across different samples or conditions. They are used as internal controls to normalize qPCR data. The expression levels of the target genes are then measured relative to the expression levels of the reference genes.
Q: What are the challenges in qPCR data normalization?
A: One of the main challenges in qPCR data normalization is the selection of suitable reference genes. It is crucial to choose reference genes that have stable expression levels across all samples or conditions being studied. Additionally, there can be technical variations and experimental biases that need to be accounted for during normalization.