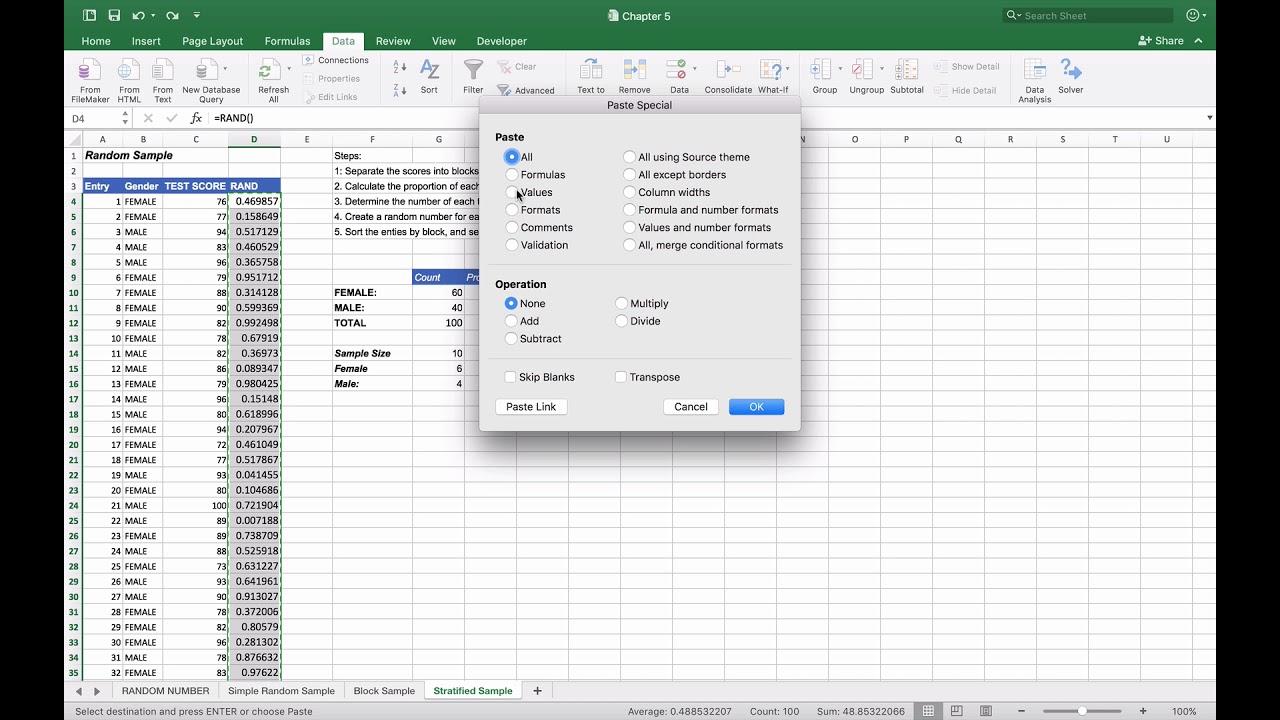
Stratifying data is a crucial step in data analysis that allows for a deeper understanding and insights into different segments or groups within a dataset. It involves dividing data into meaningful categories or strata based on specific criteria or variables, such as age, gender, income, or location.
By stratifying data, researchers and analysts can uncover patterns, trends, and differences among various subgroups, enabling more accurate interpretations and informed decision-making. This technique is commonly used in market research, healthcare studies, and social sciences to gain a comprehensive understanding of a population.
In this article, we will explore the concept of data stratification, discuss its importance, and provide step-by-step guidance on how to effectively stratify data. Whether you’re a data analyst, researcher, or simply curious about data analysis, learning how to stratify data will empower you to extract valuable insights from your datasets.
Inside This Article
- Importance of Data Stratification
- Methods of Data Stratification
- Benefits of Data Stratification
- Conclusion
- FAQs
Importance of Data Stratification
Data stratification is an essential process in data analysis that involves dividing a dataset into distinct groups or layers based on specific criteria. This technique plays a crucial role in several fields, including statistics, market research, and even healthcare. By organizing data into meaningful subsets, data stratification provides valuable insights and allows for more accurate analysis and decision-making.
One of the main reasons why data stratification is important is its ability to reduce bias and improve the accuracy of statistical results. When dealing with large datasets, there is often a risk of having imbalanced or skewed data. Stratifying the data helps to ensure that each subset contains a representative distribution of the variables being studied, which can eliminate sampling errors and enhance the validity of research findings.
Data stratification also enables researchers and analysts to identify patterns and trends within specific subgroups of data. By segmenting the dataset based on relevant factors such as demographics, geographic location, or purchasing behavior, stratification can uncover hidden insights that may be missed when analyzing the dataset as a whole. This level of granularity allows for a more comprehensive understanding of the underlying data and facilitates more targeted decision-making.
Moreover, data stratification is particularly useful in market research and customer segmentation. By dividing customers into distinct groups based on their preferences, behaviors, or characteristics, businesses can personalize their marketing strategies and tailor their offerings to different target segments. This not only improves customer satisfaction but also enhances the effectiveness of marketing campaigns, ultimately leading to higher conversion rates and increased revenue.
Furthermore, data stratification plays a critical role in healthcare research and patient care. By stratifying patient data based on factors such as age, gender, medical history, and disease severity, healthcare professionals can better understand the effectiveness of different treatments and interventions for specific patient groups. This can lead to more personalized and effective treatment plans, improved patient outcomes, and ultimately, a higher quality of care.
Methods of Data Stratification
When it comes to data analysis, stratification plays a crucial role in organizing and categorizing data for deeper insights. By dividing data into meaningful subgroups or strata, we can uncover patterns, trends, and correlations that may not be apparent when analyzing the data as a whole. Here are some common methods of data stratification:
- Simple Random Stratification: This method involves dividing the data into strata by randomly assigning observations to different groups. Each stratum should have a similar representation of the different variables being studied, ensuring that the sample is representative of the entire data set.
- Proportionate Stratification: In this method, the proportions of different variables within each stratum are proportional to their representation in the overall data set. This ensures that each stratum is represented in the sample according to its importance or prevalence in the population.
- Disproportionate Stratification: This method is used when specific subgroups within the data set need to be oversampled or undersampled to obtain a more accurate representation. It allows researchers to focus on specific strata or variables that are of particular interest or importance.
- Cluster Sampling: This method involves dividing the data into clusters based on geographical location, organizational units, or other criteria. Random samples are then taken from each cluster, which can be more cost-effective and efficient than sampling each individual observation.
- Systematic Sampling: In this method, observations are selected at regular intervals from a sorted data set. This ensures that the sample is representative of the entire data set and reduces the chances of bias or skewed results.
- Sequential Sampling: This method involves selecting samples one at a time, with the selection process influenced by the previously selected samples. It is particularly useful when the data set is dynamic and constantly changing, allowing researchers to adapt their sampling strategy accordingly.
Benefits of Data Stratification
Data stratification is a powerful technique that can bring several benefits to organizations analyzing large volumes of data. By organizing and categorizing data based on specific criteria, businesses can gain valuable insights and make more informed decisions. Here are some of the key benefits of data stratification:
- Improved Accuracy: By stratifying data, organizations can ensure that they are working with accurate and reliable information. By dividing data into meaningful groups, they can eliminate any biases or errors that may be present in the overall dataset. This increases the accuracy of analysis and decision-making processes.
- Enhanced Understanding: Data stratification allows businesses to gain a deeper understanding of different segments within their datasets. By categorizing data based on specific criteria such as demographics, geographic location, or purchase behavior, organizations can uncover meaningful patterns and trends that may have been hidden in the overall dataset. This enhanced understanding can lead to more targeted marketing campaigns and personalized customer experiences.
- Identifying Key Insights: Stratifying data helps businesses identify key insights and focus on specific areas of interest. By dividing data into smaller, more manageable subsets, organizations can analyze each subset individually to uncover unique patterns or anomalies. This can lead to the discovery of valuable insights that would have otherwise been overlooked in the vastness of the overall dataset.
- Optimized Resource Allocation: Data stratification enables organizations to optimize their resource allocation. By analyzing the different segments within their datasets, businesses can allocate their resources more efficiently. They can identify areas of high potential or areas that need improvement, allowing them to allocate their time, budget, and manpower accordingly.
- Improved Decision-Making: With data stratification, organizations can make more informed and data-driven decisions. By analyzing stratified data, businesses have access to a comprehensive view of various segments and can evaluate the impact of different strategies or actions in each segment. This allows for more targeted decision-making that takes into account the unique characteristics and needs of each segment.
Overall, data stratification offers numerous benefits to organizations seeking to analyze and utilize their data effectively. By improving accuracy, enhancing understanding, identifying key insights, optimizing resource allocation, and improving decision-making, businesses can harness the power of their data to drive success and achieve their goals.
Conclusion
In conclusion, understanding how to stratify data is an essential skill for any data analyst or researcher. By dividing data into homogeneous groups based on relevant characteristics, stratification allows for accurate analysis and interpretation of results. It helps to minimize bias and variability, leading to more reliable insights and informed decision-making.
Stratification can be applied in various fields, such as market research, healthcare, social sciences, and more. It enables researchers to uncover meaningful patterns and relationships within the data, leading to deeper insights and a better understanding of complex phenomena. Properly stratified data provides the foundation for robust statistical analysis and predictive modeling, allowing for more precise and actionable research findings.
By utilizing stratified data, businesses and organizations can make data-driven decisions, develop targeted marketing strategies, improve customer segmentation, and identify trends and patterns that may not be apparent when analyzing the data as a whole.
Overall, stratifying data is a powerful technique that enhances the accuracy and validity of data analysis, leading to more impactful and actionable results. Whether you are a beginner or an experienced analyst, incorporating stratification into your data analysis toolkit can greatly benefit your research endeavors.
FAQs
1. What is data stratification?
Data stratification is a technique used to divide a dataset into distinct groups or strata based on certain characteristics or variables. This stratification helps analyze and understand the data more effectively, allowing for targeted analysis and decision-making.
2. Why is data stratification important?
Data stratification is important because it allows for a more in-depth analysis of specific groups within a dataset. By dividing the data into smaller, more homogeneous strata, it becomes easier to identify patterns, trends, or differences that may not be apparent when analyzing the data as a whole.
3. What are the benefits of data stratification?
Data stratification offers several benefits. Firstly, it helps in identifying and understanding differences between subgroups within a dataset, providing more accurate insights. It also enables targeted decision-making and interventions by focusing on specific strata. Moreover, stratification can enhance the efficiency of statistical analyses and improve the precision of results.
4. How is data stratified?
Data is stratified by identifying relevant variables or factors and dividing the dataset into groups or strata based on those variables. The selection of these variables depends on the specific objectives of the analysis and the characteristics of the dataset. Common stratification factors include age, gender, geographic location, income level, and more.
5. What are some common methods of data stratification?
There are various methods of data stratification, including random stratification, proportional stratification, and optimal stratification. Random stratification involves dividing the data into equal-sized strata randomly. Proportional stratification involves dividing the data in a way that each stratum represents a proportional sample of the whole population. Optimal stratification aims to minimize the variability within each stratum to achieve more precise results.