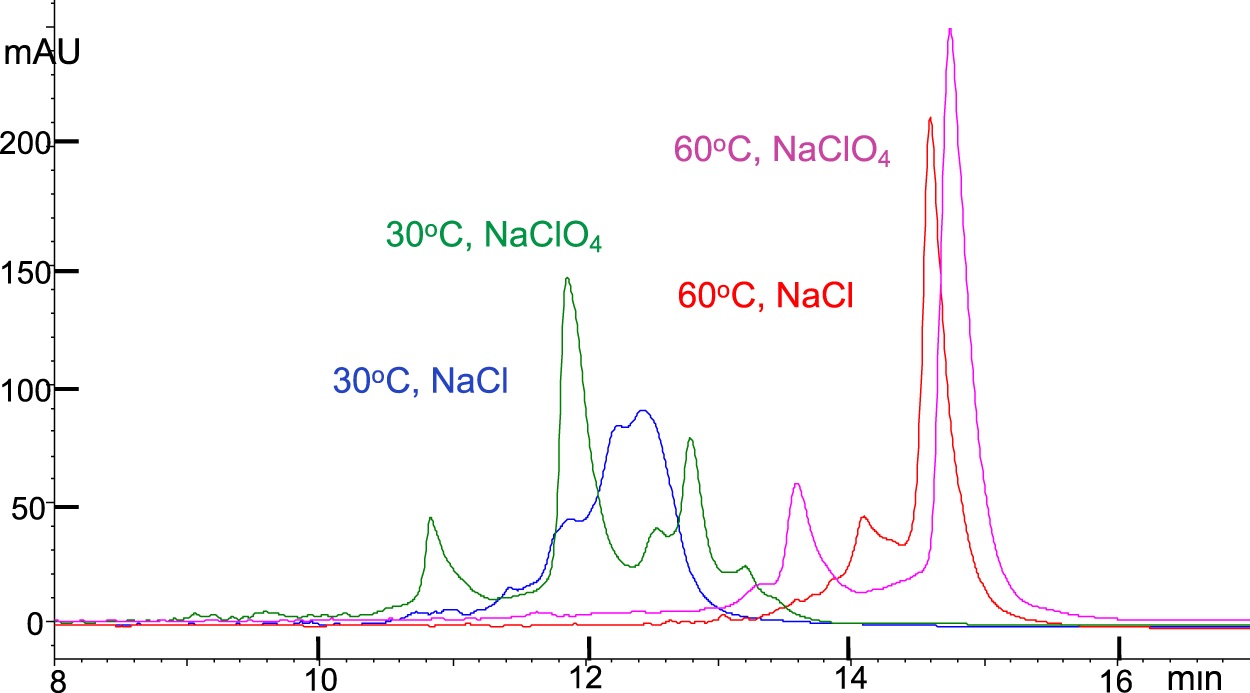
If you’re in the field of analytical chemistry, specifically dealing with HPLC (High-Performance Liquid Chromatography), you know the importance of analyzing HPLC data. HPLC is a powerful technique used to separate, identify, and quantify components in a sample. The data generated from HPLC analysis provides valuable insights into the composition and concentration of compounds in various industries, including pharmaceuticals, food and beverage, environmental science, and more.
However, navigating and interpreting HPLC data can be complex and overwhelming, especially for those new to the field. In this article, we will guide you through the process of analyzing HPLC data, providing you with the knowledge and skills necessary to make informed decisions and draw meaningful conclusions from your results. So, whether you’re a seasoned analytical chemist or just starting out, let’s dive into the world of HPLC data analysis!
Inside This Article
- How to Analyze HPLC Data
- Preprocessing of HPLC data
- Peak Identification and Integration
- Quantitative analysis of HPLC data
- Data interpretation and reporting
- Conclusion
- FAQs
How to Analyze HPLC Data
Analyzing HPLC (High Performance Liquid Chromatography) data plays a vital role in understanding the composition and quality of chemical samples. Whether you are working in a research laboratory or a quality control setting, accurate and efficient analysis of HPLC data is essential. In this article, we will guide you through the steps of analyzing HPLC data to obtain meaningful results.
1. Preprocessing of HPLC data:
Before diving into the analysis, it is important to preprocess the HPLC data to ensure its accuracy and quality. This involves tasks like baseline correction, noise reduction, and smoothing of the chromatograms. By optimizing the preprocessing parameters, you can enhance the signal-to-noise ratio and overall data quality.
2. Peak identification and integration:
The next step is to identify and integrate the peaks in the chromatogram. Peaks represent individual components within the sample, and their identification and integration allow for quantitative analysis. Software tools like chromatographic data systems (CDS) can assist in automating this process, reducing manual effort and ensuring consistency.
3. Quantitative analysis of HPLC data:
Once the peaks have been identified and integrated, quantitative analysis can be performed. This involves determining the concentrations of the analytes present in the sample. Calibration curves can be used to relate the peak areas or heights to the concentration of the analyte.This information is crucial for assessing the quality and purity of the sample, and for comparing it to predefined specifications or regulatory limits.
4. Data interpretation and reporting:
The final step in HPLC data analysis is the interpretation and reporting of the results. This includes analyzing the data in the context of the research goals or quality control requirements. Statistical analysis and data visualization techniques can be employed to gain insights into trends, outliers, and relationships between variables. The results should be documented and reported accurately, allowing for informed decision making and further analysis, if necessary.
By following these steps, you can effectively analyze HPLC data and extract valuable information about the composition and quality of your samples. Remember that proper preprocessing, peak identification and integration, quantitative analysis, and data interpretation are key to obtaining reliable and meaningful results in HPLC analysis.
Preprocessing of HPLC data
When it comes to analyzing HPLC data, preprocessing plays a crucial role in ensuring accurate and reliable results. Before diving into the detailed analysis, it is important to prepare the data by structuring and organizing it in a way that facilitates further analysis. This involves several steps, each aimed at optimizing the quality of the data for subsequent processing.
The first step in preprocessing HPLC data is to remove any noise or baseline fluctuations. HPLC data often contains small oscillations and irregularities due to system and environmental factors. To address this, various smoothing techniques, such as moving average or Savitzky-Golay filtering, can be applied to eliminate the noise without significantly affecting the peaks of interest.
Next, a baseline correction is necessary to correct for any shifts or slopes in the baseline. Baseline variations can arise from factors such as detector drift, column deterioration, or changes in mobile phase composition. By fitting a baseline model, such as a straight line or polynomial function, and subtracting it from the raw data, the baseline can be leveled, enabling accurate peak analysis.
In addition to noise and baseline corrections, data alignment is another crucial preprocessing step. HPLC data collected over different runs or instruments may suffer from slight retention time shifts, making it difficult to compare or integrate peaks accurately. By aligning the data based on common peaks or landmarks, such as internal standards or well-known compounds, the retention time discrepancies can be adjusted, ensuring consistency in peak identification and quantification.
Lastly, data normalization is important when comparing HPLC analyses from different samples or conditions. Normalization involves scaling the data to a common reference point, such as the total peak area or the intensity of an internal standard. This not only facilitates comparison across samples but also helps to minimize variations caused by differences in injection volume or concentration.
Peak Identification and Integration
In High-Performance Liquid Chromatography (HPLC) analysis, peak identification and integration play a crucial role in determining the composition and concentration of compounds in a sample. This process involves the identification and quantification of individual peaks in the chromatogram, which corresponds to different components present in the sample.
Peak identification is the process of assigning a specific compound or analyte to a particular peak on the chromatogram. This is usually done by comparing the retention time of the peak with the retention time of known standards or reference compounds. By matching the retention time, scientists can confidently attribute a peak to a specific compound, thereby aiding in compound identification.
Integration, on the other hand, involves calculating the area under the curve of each peak in the chromatogram. The integration process measures the peak heights or intensities and calculates the area that represents the quantity or concentration of the respective compound. This data is crucial for quantitative analysis and comparing the levels of different compounds in the sample.
There are different methods and algorithms used for peak identification and integration in HPLC analysis. These methods can vary depending on the specific HPLC instrument and software being used. The most common approach is the use of peak detection algorithms that automatically identify and integrate the peaks in the chromatogram based on certain predefined parameters such as peak width, height, and signal-to-noise ratio.
Once the peaks are identified and integrated, it is necessary to validate the results through various quality control measures. This involves verifying the peak shape, baseline integrity, and calculating the signal-to-noise ratio to ensure accurate and reliable data. If any anomalies or inconsistencies are detected, further investigation or troubleshooting may be required.
In addition to peak identification and integration, it is also important to consider factors such as peak purity, resolution, and retention time variability in HPLC analysis. These factors can affect the accuracy and reliability of the results, especially in complex samples with overlapping or closely eluting peaks. Proper calibration and optimization of the HPLC system can help mitigate these issues and improve the overall accuracy and sensitivity of the analysis.
Quantitative analysis of HPLC data
Quantitative analysis plays a vital role in HPLC data interpretation. It involves determining the concentration of target compounds in a given sample based on the peak areas or heights obtained from the chromatogram. This analysis provides valuable information for various applications, such as pharmaceutical analysis, environmental testing, and quality control in the food and beverage industry.
To perform quantitative analysis, calibration curves are constructed using standard solutions with known concentrations of the target compounds. These standards are injected into the HPLC system, and their peak areas or heights are measured. By plotting the peak areas or heights against the known concentrations, a linear regression equation can be obtained. This equation serves as a basis for quantifying the target compounds in the sample.
Once the calibration curve is established, the peak areas or heights of the target compounds in the sample are measured. These measurements are then substituted into the regression equation to determine their concentrations. The accuracy of the quantitative analysis depends on the linearity and sensitivity of the calibration curve, as well as the precision of the HPLC system.
In addition to the calibration curve, other factors should be considered during quantitative analysis. For instance, the retention times of the target compounds should align with those of the standards to ensure accurate identification. Any variations in the column temperature, mobile phase composition, or flow rate can affect the retention times and subsequently impact the accuracy of quantitative analysis.
Furthermore, it is important to validate the quantitative analysis method by assessing its specificity, accuracy, precision, linearity, and robustness. This validation process ensures that the method is reliable and can be consistently utilized for determining the concentrations of target compounds in different samples.
Quantitative analysis of HPLC data provides crucial information for researchers, scientists, and analysts in various industries. It allows for the accurate determination of compound concentrations, aiding in quality control, research, and regulatory compliance. By employing robust analytical methods and adhering to proper data interpretation techniques, researchers can confidently analyze HPLC data and obtain reliable quantitative results.
Data interpretation and reporting
Data interpretation is a crucial step in the analysis of HPLC data. Once the peaks have been identified and integrated, the next step is to interpret the results. This involves analyzing the peak shapes, retention times, and peak areas to determine the concentration and purity of the analyte.
One important aspect of data interpretation is the comparison of the obtained results with standard reference values or specifications. By comparing the peak retention times and areas with those of known standards, it is possible to confirm the identity of the analyte and assess its purity. Deviations from the expected values may indicate the presence of impurities or other variations in the sample.
Another important aspect of data interpretation is the calculation of the sample concentration. This is typically done by comparing the peak area of the analyte with a calibration curve or standard curve. The calibration curve is prepared by analyzing solutions with known concentrations of the analyte. By plotting the peak area against the concentration, a linear relationship can be established, which allows for the determination of the sample concentration.
In addition to concentration, other parameters can be derived from the HPLC data, such as peak asymmetry and resolution. Peak asymmetry refers to the shape of the peak and can provide information about the efficiency of the separation. A symmetric peak indicates a well-resolved and efficient separation, while an asymmetric peak may indicate column deterioration or other issues.
Resolution, on the other hand, quantifies the degree of separation between adjacent peaks. A higher resolution indicates better separation, which is desirable in HPLC analysis. By evaluating these parameters, it is possible to assess the quality of the analysis and make any necessary adjustments to improve the results.
Once the data has been interpreted, it is important to report the findings accurately. This includes documenting the peak retention times, areas, and concentrations of the analyte, as well as any relevant parameters like peak asymmetry and resolution. It is also important to provide any additional information about the sample preparation or equipment used in the analysis.
Reporting the data in a clear and concise manner is essential, as it allows for easy communication and reproduction of the analysis. This is particularly important for quality control purposes or when sharing the results with other researchers or regulatory bodies. A well-documented and comprehensive report ensures that the analysis can be verified and replicated, and provides a solid foundation for further research or investigations.
Conclusion
In conclusion, analyzing HPLC data is a crucial step in understanding the composition and quality of samples in various scientific fields. By following the proper techniques and utilizing the necessary tools, researchers and analysts can extract valuable information from HPLC data, such as peak identification and quantification, sample purity, and compound identification.
Through the application of advanced data analysis methods and software, HPLC data can provide insights into the quality control of pharmaceuticals, the identification of pollutants in environmental samples, and the monitoring of food and beverage quality, among many other applications. The ability to effectively analyze HPLC data empowers scientists and researchers to make well-informed decisions based on accurate and reliable results.
By understanding the principles of HPLC, carefully calibrating instruments, and employing data analysis techniques, scientists and analysts can enhance their ability to interpret and draw meaningful conclusions from HPLC data. As technology continues to advance, the field of HPLC data analysis will continue to evolve, leading to more efficient and accurate results, ultimately pushing the boundaries of scientific discovery.
FAQs
1. What is HPLC?
HPLC, short for High-Performance Liquid Chromatography, is a powerful analytical technique used to separate, identify, and quantify components in a sample. It involves the flow of a liquid solvent under high pressure through a column packed with a stationary phase. This separation technique is widely used in various industries, including pharmaceuticals, environmental analysis, and food testing.
2. What is HPLC data analysis?
HPLC data analysis refers to the process of interpreting and extracting meaningful information from the data obtained through an HPLC analysis. This involves examining the chromatograms, peaks, retention times, and other parameters to make quantitative and qualitative assessments of the sample components. The data analysis phase is crucial for extracting valuable insights and drawing conclusions from the HPLC experiment.
3. What are the common steps involved in analyzing HPLC data?
The common steps involved in analyzing HPLC data include data acquisition, pre-processing, peak detection, integration, calibration, peak identification, quantification, and reporting. Data acquisition involves recording the signals from the detector during the HPLC run. Pre-processing involves filtering, smoothing, or baseline correction of the acquired raw data. Peak detection and integration focus on identifying and measuring the height, area, and retention time of the peaks. Calibration is done by relating the peak areas to known concentrations to determine the sample concentrations. Peak identification involves comparing retention times with reference standards, while quantification determines the amount of each component present. Finally, reporting generates a concise summary of the results.
4. What are some software tools used for HPLC data analysis?
There are several software tools available for HPLC data analysis, including industry-standard programs like Agilent OpenLAB, Waters Empower, Shimadzu LabSolutions, and Thermo Scientific Chromeleon. These software packages provide a user-friendly interface for efficient data analysis, visualization, and reporting. They typically offer features such as peak integration, calibration curve generation, data manipulation, integration with spectral libraries, and advanced statistical analysis.
5. What are the challenges in HPLC data analysis?
HPLC data analysis can be challenging due to several factors, including peak overlapping, baseline noise, peak tailing, retention time shifts, or the presence of impurities. Ensuring accurate peak detection, integration, and quantification can be particularly demanding. Data interpretation and troubleshooting require a deep understanding of chromatographic principles, method development, and troubleshooting techniques. It is also important to consider factors such as system suitability, method validation, and compliance with regulatory requirements to ensure reliable and reproducible results.