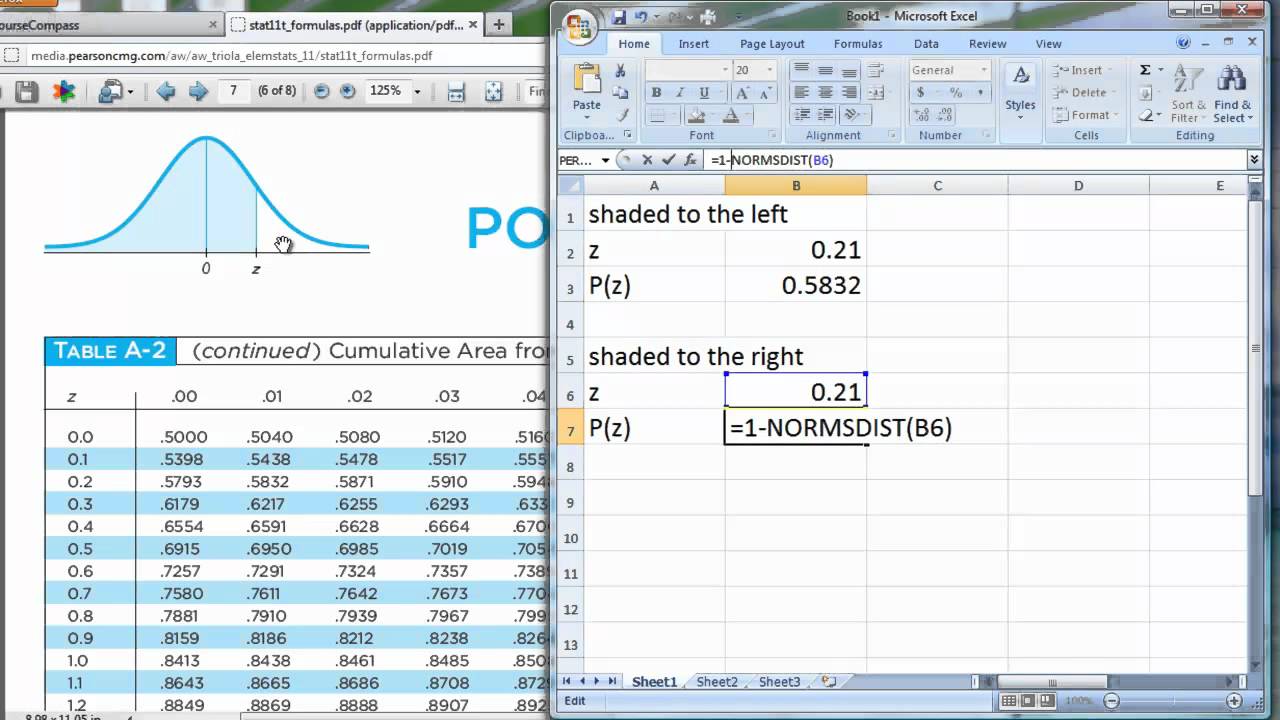
When working with data in Excel, it is important to determine whether it follows a normal distribution. Knowing the distribution of your data can help you make more informed statistical analyses and draw accurate conclusions. A normal distribution, also known as a Gaussian distribution or a bell curve, is characterized by a symmetrical shape and a peak at the center.
In this article, we will explore how to check if data is normally distributed in Excel. We will discuss different methods and techniques that can be used to assess the normality of your data and ensure the reliability of your analysis. Whether you are working on a research project, conducting surveys, or analyzing financial data, understanding the normality of your data is crucial for accurate interpretation and decision-making.
Inside This Article
- Subtitle 1: Overview of Data Normality
- Subtitle 2: Using Descriptive Statistics for Normality Testing
- Subtitle 3: Creating a Frequency Distribution Plot
- Subtitle 4: Implementing Statistical Tests for Normality
- Conclusion
- FAQs
Subtitle 1: Overview of Data Normality
When analyzing data, it is crucial to determine whether it follows a normal distribution. A normal distribution, also known as a bell curve, is a statistical concept that describes a symmetrical pattern of data centered around a mean value. This distribution is widely used in statistical analysis and serves as the foundation for many inferential techniques.
Identifying whether your data is normally distributed is important as it allows you to make informed decisions about which statistical tests and techniques are appropriate for analyzing your data. If your data is normally distributed, you can confidently apply parametric tests such as t-tests or analysis of variance (ANOVA). However, if your data is not normally distributed, non-parametric tests like the Mann-Whitney U test or Kruskal-Wallis test may be more suitable.
While it is unrealistic to expect data to perfectly adhere to a normal distribution, a general assessment of data normality can be made through statistical analysis and graphical visualization. In this article, we will explore various methods to check if data is normally distributed in Excel.
Before we delve into the specific techniques, it is essential to understand the key characteristics of a normal distribution. A normal distribution exhibits the following traits:
- Symmetry: The distribution is symmetrical, with the mean, median, and mode all aligned at the center of the distribution.
- Bell-shaped curve: The data forms a smooth, bell-shaped curve, with the majority of data points clustered around the center and tapering off towards the tails.
- Equal measures of dispersion: The distribution exhibits equal measures of dispersion on either side of the mean, as indicated by the standard deviation.
Now that we have a basic understanding of data normality and its key features, let’s explore some methods to assess if our data follows a normal distribution in Excel.
Subtitle 2: Using Descriptive Statistics for Normality Testing
When it comes to testing the normality of data in Excel, descriptive statistics can be a powerful tool. Descriptive statistics provide useful information about the central tendency, dispersion, and shape of a dataset. These measures can give us insights into whether the data follows a normal distribution or not.
One common descriptive statistic used for normality testing is skewness. Skewness measures the asymmetry of a dataset. A skewness value of zero indicates that the data is perfectly symmetrical, while positive or negative skewness values indicate right or left skewness respectively.
Another important statistic is kurtosis, which measures the peakedness of a dataset’s distribution. A kurtosis value of three signifies a normal distribution, while values greater than three indicate higher peakness (leptokurtic) and values less than three suggest flatter distributions (platykurtic).
In Excel, you can easily calculate skewness and kurtosis using the built-in functions. The SKEW function calculates the skewness of a dataset, while the KURT function calculates the kurtosis. Once you have these values, you can use them as indicators to assess the normality of the dataset.
It’s important to note that while skewness and kurtosis can provide valuable insights, they are not definitive tests for normality. They are just indicators that can guide the analysis. To perform a more rigorous normality test, you will need to use specific statistical tests, which will be discussed later.
In addition to skewness and kurtosis, other descriptive statistics like mean, median, and standard deviation can also be helpful in assessing normality. If the mean and median are similar, and the standard deviation is relatively low, it suggests that the data is likely to be normally distributed.
By calculating and reviewing these descriptive statistics in Excel, you can get a better understanding of the distribution of your data and make an initial assessment of its normality. However, it’s important to remember that descriptive statistics alone are not enough to conclusively determine whether data is normally distributed or not. For a more comprehensive analysis, it’s recommended to use additional statistical tests.
Subtitle 3: Creating a Frequency Distribution Plot
Creating a frequency distribution plot is a helpful way to visually assess the distribution of data and determine if it follows a normal distribution. Essentially, this plot displays the number of occurrences, or frequency, of data values within specific intervals or bins.
To create a frequency distribution plot in Excel, you can follow these steps:
- First, organize your data in a column or row within an Excel worksheet.
- Select a blank column next to the data or choose an empty column where you want the frequency distribution to be displayed.
- Go to the “Data” tab in the Excel ribbon and click on “Data Analysis” in the “Analysis” group. If you don’t see this option, you may need to enable the Analysis ToolPak add-in.
- In the Data Analysis dialog box, select “Histogram” and click “OK”.
- In the Histogram dialog box, specify the input range as the data you want to analyze and the bin range as the range where you want the bins to appear.
- Enable the option for “Chart Output” if you want to create a histogram chart along with the frequency distribution table.
- Click “OK” to generate the frequency distribution plot.
Once you have created the frequency distribution plot, you can assess the shape of the distribution and evaluate if it appears to be normally distributed. Look for a symmetric bell-shaped curve, where the data is clustered around the mean.
Keep in mind that a frequency distribution plot alone is not a definitive test for normality, but it can provide valuable insights into the distribution of your data. If the plot exhibits a symmetrical bell shape, it suggests a normal distribution. However, if the data appears skewed or has multiple peaks, it may indicate a deviation from normality.
It’s important to note that a frequency distribution plot is just one approach to assess normality, and it’s recommended to use it in conjunction with other statistical tests for a more comprehensive analysis.
Subtitle 4: Implementing Statistical Tests for Normality
Once you have examined the visual representation of your data using a frequency distribution plot, you may want to conduct a formal statistical test to determine whether your data is normally distributed. There are several commonly used statistical tests for normality, and in this section, we will explore a few of the most widely used ones.
1. Shapiro-Wilk Test: The Shapiro-Wilk test is a popular test for normality and is widely used when the sample size is small to moderate. It calculates a test statistic based on the correlation between the ordered values of the data and the expected values if the data were normally distributed. If the p-value associated with the test is less than the chosen significance level (typically 0.05), we reject the null hypothesis of normality.
2. Kolmogorov-Smirnov Test: The Kolmogorov-Smirnov test is another commonly used test for normality. It compares the cumulative distribution function (CDF) of your data with the CDF of a normal distribution. The test calculates a test statistic based on the maximum difference between the empirical CDF and the theoretical CDF. If the p-value associated with the test is less than the chosen significance level, we reject the null hypothesis of normality.
3. Anderson-Darling Test: The Anderson-Darling test is a modification of the Kolmogorov-Smirnov test and is often considered more powerful, especially for small sample sizes. The test computes a test statistic based on the ordered values of the data and the expected values under the null hypothesis of normality. If the p-value associated with the test is less than the specified significance level, we reject the null hypothesis of normality.
4. Lilliefors Test: The Lilliefors test is a variant of the Kolmogorov-Smirnov test and is specifically designed for testing normality. It estimates the critical values necessary to evaluate the test statistic directly from the data without relying on tables of critical values. If the p-value associated with the test is less than the chosen significance level, we reject the null hypothesis of normality.
It is important to note that these tests are sensitive to sample size. When the sample size is small, even slight departures from normality can lead to rejection of the null hypothesis. On the other hand, with large sample sizes, the tests may have high power to detect even minor departures from normality, leading to rejection of the null hypothesis.
Remember that statistical tests for normality are not definitive proof of the distribution’s shape; they are just tools to assess the assumption of normality. It is essential to consider the results of these tests along with other diagnostic measures and the context of the data.
In conclusion, being able to identify whether data is normally distributed in Excel is a valuable skill for data analysts and researchers. Understanding the distribution of data is crucial for making informed decisions and drawing accurate conclusions from statistical analyses.
By using the various methods available in Excel, such as visual inspections, summary statistics, and hypothesis tests, you can determine if your data follows a normal distribution. Remember, a visual inspection can provide a quick indication, while summary statistics and hypothesis tests offer more conclusive evidence.
Whether you are conducting research, performing data analysis, or making business decisions, knowing how to check if data is normally distributed in Excel empowers you to confidently interpret and utilize your data. So, take the time to explore these methods and ensure the validity of your findings.
FAQs
1. What is data distribution?
Data distribution refers to the pattern or spread of data values in a dataset. It helps us understand how the data is spread out across different values or ranges.
2. What is meant by “normal distribution”?
A normal distribution, also known as a Gaussian distribution, is a statistical distribution where the data is symmetrically distributed around the mean, forming a bell-shaped curve. In a normal distribution, most of the data values are concentrated around the mean, with fewer values towards the tails of the distribution.
3. Why is it important to check if data is normally distributed?
Checking if data is normally distributed is important because many statistical tests and models assume that the data follows a normal distribution. If the data is not normally distributed, these tests and models may provide inaccurate or unreliable results. Therefore, it is crucial to assess the distribution of the data before applying any statistical techniques.
4. How can we check if data is normally distributed in Excel?
In Excel, you can check if the data is normally distributed using the Histogram tool, Q-Q plot, or by performing statistical tests such as the Shapiro-Wilk test or the Anderson-Darling test. These methods help assess whether the data follows a normal distribution based on visual observations or statistical measures.
5. Can we transform non-normally distributed data into a normal distribution?
Yes, it is possible to transform non-normally distributed data into a normal distribution through various transformations like logarithmic, square root, or inverse transformations. These transformations can help achieve a more normal-like distribution, which can be beneficial for further statistical analysis or modeling purposes.