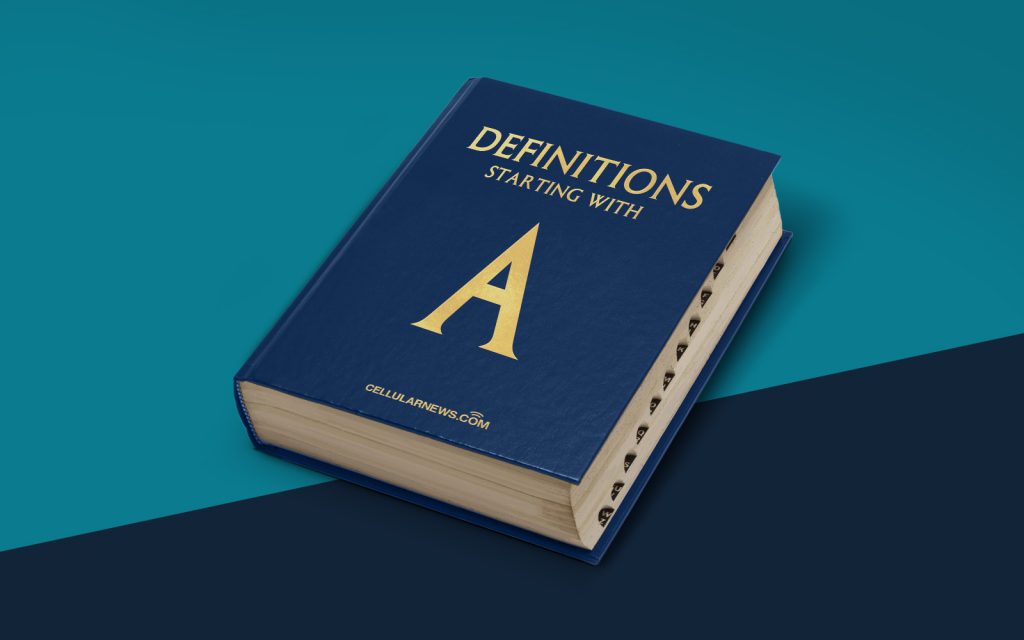
Welcome to the World of Automatic Summarization!
Have you ever come across a lengthy document or article and thought, “I wish there was a way to get the key points without having to read through all of this?” Well, you’re in luck! Automatic summarization is here to help. In this article, we will explore the fascinating world of automatic summarization and understand how it can save you time and effort.
Key Takeaways:
- Automatic summarization condenses lengthy documents or articles into shorter versions, extracting the most important information.
- There are two main types of automatic summarization: extractive and abstractive.
Unveiling the Mystery: What is Automatic Summarization?
Automatic summarization is a process that aims to reduce the length of a document while retaining its essential information. It accomplishes this by identifying the most important sentences or phrases and condensing them into a concise summary. It’s like having a personal assistant who reads through the entire document and picks out the key points, saving you precious time and effort.
Automatic summarization is a critical component of natural language processing (NLP) and text mining. The goal is not only to provide a shorter version of a document but also to capture its core meaning and essential concepts.
Now, let’s dive a little deeper into the two main types of automatic summarization:
Extractive Summarization: Extracting Nuggets of Information
In extractive summarization, the algorithm identifies and pulls out the most important sentences or phrases from the source document to create a summary. It essentially extracts the “nuggets” of information that capture the essence of the original content. This approach is comparable to highlighting key points in a text and presenting them as a summary. It ensures that the summary contains verbatim fragments from the original document, maintaining its authenticity.
Extractive summarization relies on statistical models and algorithms to determine the importance of sentences based on factors such as word frequency, position within the text, or their relation to other sentences. By selecting and assembling these sentences, extractive summarization generates a concise summary that represents the main ideas and factual information.
Abstractive Summarization: Creating a Summary from Scratch
Abstractive summarization, on the other hand, goes a step further and generates a summary by understanding the content and paraphrasing it. It doesn’t rely solely on extracting sentences but instead creates new sentences that capture the meaning of the original text. Think of it as a human writer who reads a document and crafts a summary in their own words.
This advanced approach requires natural language understanding, semantic analysis, and language generation techniques. Abstractive summarization algorithms interpret the document’s context, identify key concepts, and generate condensed sentences that convey the same information as the original text. It allows for more flexibility and can produce summaries that are not limited to the exact phrasing of the input document.
In Conclusion
Automatic summarization is a powerful tool that saves time and effort by condensing lengthy documents into shorter, more manageable versions. Whether you need to skim through research papers, news articles, or business reports, automatic summarization can provide you with the essential information you need without reading every word.
Now that you understand the world of automatic summarization, you can take advantage of this technology to stay informed, make faster decisions, and extract the key insights from a sea of information.