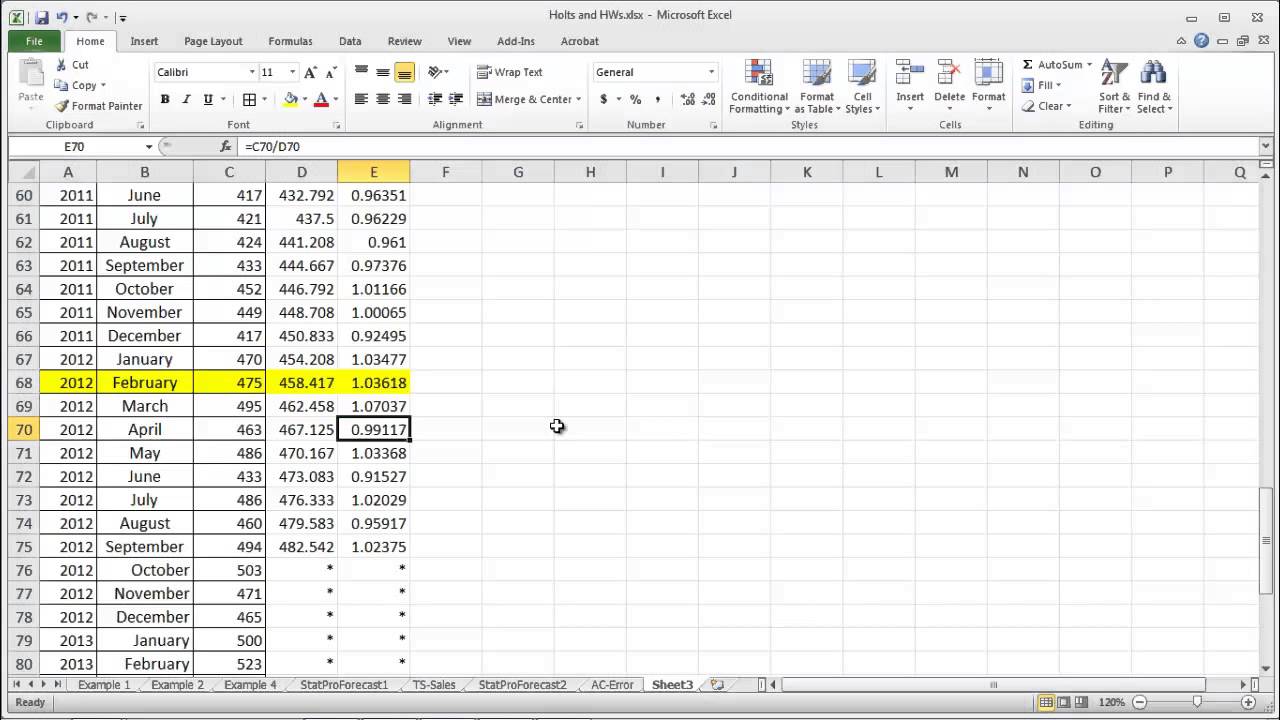
Seasonality can greatly impact the accuracy of data analysis and forecasting in Excel. Fluctuations in data due to factors like holidays, weather patterns, or seasonal trends can obscure underlying patterns and make it difficult to draw meaningful insights. This is where deseasonalizing data in Excel becomes crucial.
Deseasonalizing data involves removing the seasonal component from the dataset, allowing for a clearer understanding of the trend and making it easier to make accurate predictions. By deseasonalizing data in Excel, you can identify the underlying patterns and trends that may be obscured by seasonal variations.
In this article, we will explore various methods and techniques to deseasonalize data in Excel. Whether you are an analyst, a business owner, or simply someone who works extensively with data, understanding how to deseasonalize data in Excel will empower you to extract more accurate and valuable insights from your datasets.
Inside This Article
- What is Seasonal Data?
- Why Deseasonalize Data?
- Methods of Deseasonalizing Data in Excel
- Using Moving Averages to Deseasonalize Data
- Conclusion
- FAQs
What is Seasonal Data?
Seasonal data refers to the pattern of data that exhibits regular and predictable fluctuations at specific time intervals. These fluctuations tend to repeat themselves over a fixed period, such as monthly, quarterly, or annually. There are various factors that can contribute to seasonal patterns in data, including weather conditions, holiday seasons, or even economic cycles.
For example, if you were to analyze sales data for ice cream, you would most likely observe higher sales during the summer months and lower sales during the winter months. This recurring pattern of higher and lower sales is a clear indication of the seasonality present in the data.
Identifying and understanding seasonal data is crucial for businesses and analysts as it can provide valuable insights into trends, patterns, and forecasting. By recognizing these seasonal variations, organizations can make informed decisions about inventory management, production planning, and marketing strategies to optimize their operations.
It is important to note that seasonality can not only impact sales data but also other types of data such as website traffic, customer behavior, and financial metrics. Being able to recognize and account for seasonality is essential for accurate analysis and interpretation of data.
Why Deseasonalize Data?
Seasonal data refers to data that exhibits regular patterns or fluctuations within specific time frames, such as monthly, quarterly, or yearly cycles. While such patterns can provide valuable insights, they can also mask the underlying trends and make it challenging to analyze the data accurately. This is where deseasonalizing data becomes crucial.
Deseasonalizing data involves removing the seasonal component from the dataset, allowing you to focus on the underlying trend and make more accurate forecasts or comparisons. By eliminating the seasonal effects, you can uncover hidden patterns, identify long-term trends, and make more informed business decisions.
Deseasonalizing data is particularly important for industries that experience significant fluctuations throughout the year, such as retail, tourism, or agriculture. By understanding the underlying trends and removing the seasonal variations, businesses can identify demand patterns, optimize inventory management, and plan marketing strategies more effectively.
Additionally, deseasonalized data can be beneficial for financial analysis and forecasting. By accounting for seasonal fluctuations in the data, financial analysts can make more accurate projections, detect anomalies, and assess the true performance and growth potential of a business.
Overall, deseasonalizing data allows for a deeper understanding of the underlying trends and patterns, enabling organizations and individuals to make better decisions, allocate resources effectively, and optimize their strategies for long-term success.
Methods of Deseasonalizing Data in Excel
Deseasonalizing data is an essential step in data analysis, particularly when dealing with time series data. It allows analysts to identify and remove the seasonal patterns from the data, allowing for a clearer understanding of the underlying trends and making accurate predictions or forecasting.
Luckily, Excel offers several methods for deseasonalizing data, providing users with flexibility and ease of use. Let’s explore some of the popular techniques:
- Seasonal Indexing: This method involves calculating the seasonal index for each period, which represents how much the observed value deviates from the average for that specific season. The seasonal index is a ratio that enables us to adjust the data for seasonality. By dividing the original data by the seasonal index, we can deseasonalize the data and focus on the underlying trends.
- Ratio-to-Moving Average: This technique involves dividing the observed values by the corresponding moving averages for each period. Moving averages smooth out the data and help identify the unchanging trend. The ratio-to-moving average method allows us to remove the impact of seasonality from the data by dividing the observed values by the moving average for the respective period.
- Dummy Variable Regression: In this method, we create dummy variables to represent each season or period. These dummy variables take a value of 1 for the particular season and 0 for all other seasons. By including these dummy variables in a regression model, we can estimate the effect of each season on the data, effectively deseasonalizing it.
- Exponential Smoothing: Exponential smoothing is a popular technique for time series analysis. It involves assigning weights to past observations and using these weighted averages to forecast future values. By applying exponential smoothing, we can remove the seasonal component from the data, revealing the underlying trends.
- X-12-ARIMA: X-12-ARIMA is a powerful software tool for deseasonalizing data. It utilizes an advanced seasonal adjustment method that combines autoregression and moving average techniques. X-12-ARIMA provides a comprehensive analysis of the seasonal patterns in the data and allows for accurate removal of seasonality.
These are just a few of the many methods available in Excel for deseasonalizing data. Depending on the nature of your data and the level of seasonality, you may find one method more suitable than the others. It’s always recommended to experiment with different techniques and choose the one that provides the most accurate and meaningful results for your analysis.
Using Moving Averages to Deseasonalize Data
Moving averages can be a powerful tool in deseasonalizing data in Excel. A moving average is a technique that smooths out the fluctuations in data by calculating the average of a specified number of consecutive data points. It helps reveal the underlying pattern or trend in the data, removing the seasonal variations.
To use moving averages for deseasonalizing data, follow these steps:
- Arrange the data in chronological order, with the seasonal patterns clearly visible.
- Select the number of periods you want to include in the moving average calculation. For example, if you have monthly data and want to deseasonalize it, you can use a 12-month moving average.
- Create a new column next to your original data to store the deseasonalized values.
- Calculate the moving average for each data point using Excel’s AVERAGE function.
- Subtract the moving average from the original data point to obtain the deseasonalized value.
- Repeat steps 4 and 5 for each data point in the series.
By subtracting the moving average from each data point, you remove the seasonal component and are left with the deseasonalized values. These deseasonalized values can then be used for further analysis, forecasting, or comparison.
It’s important to note that the choice of the number of periods for the moving average calculation will depend on the frequency and length of the seasonal pattern in your data. You may need to experiment with different moving average periods to find the best fit for your data.
Additionally, moving averages may not be suitable for all types of data. If your data exhibits strong trends or irregular patterns, other deseasonalization methods such as exponential smoothing or regression analysis may yield better results.
Overall, using moving averages in Excel can be a valuable technique for deseasonalizing data. It helps remove the distortions caused by seasonal variations and allows you to focus on the underlying trends and patterns in your data.
Conclusion
Performing seasonal analysis is crucial to understanding patterns and trends in data. However, dealing with seasonality can pose challenges when analyzing data in Excel. Fortunately, by applying deseasonalization techniques, you can remove the seasonal component and extract the underlying patterns in your data.
Through this article, we have explored various methods to deseasonalize data in Excel, such as the moving average approach, ratio-to-moving-average method, and seasonal decomposition of time series. Each method has its own advantages and may be suitable for different types of data.
Remember, deseasonalizing your data allows you to identify and analyze the underlying changes in your data, making it easier to make informed decisions and predictions. Whether you are working with sales data, financial data, or any other seasonal data, implementing deseasonalization techniques in Excel can greatly enhance your data analysis capabilities.
Now that you have gained insight into deseasonalizing data in Excel, you can unlock the true potential of your data and uncover valuable insights to drive better decision-making and improve your business performance. So, go ahead and start applying these techniques- you’ll be amazed at the hidden patterns and trends that await you!
FAQs
- 1. What is data deseasonalization in Excel?
- 2. Why is it important to deseasonalize data in Excel?
- 3. How can I deseasonalize data in Excel?
- 4. Are there any Excel functions or tools available for data deseasonalization?
- 5. Can I deseasonalize data in Excel for any type of dataset?
Data deseasonalization in Excel is a technique used to remove the seasonal patterns and fluctuations from a dataset. It allows you to analyze and compare data without the influence of seasonal factors, such as monthly or yearly variations. It is especially useful when you want to identify underlying trends or forecast future values based on the deseasonalized data.
Deseasonalizing data in Excel is important because it helps to reveal the true underlying trends and patterns in the data. By removing seasonal fluctuations, you can better understand the long-term trends, make accurate forecasts, and make informed decisions based on the deseasonalized data.
There are several methods you can use to deseasonalize data in Excel. One common approach is to calculate and remove the seasonal indices. This involves dividing each data point by its respective seasonal index to normalize the data. Another method is to use advanced statistical techniques like ARIMA modeling to capture and remove the seasonal component from the dataset.
While Excel doesn’t have built-in functions specifically designed for data deseasonalization, you can use a combination of mathematical formulas, pivot tables, and other data analysis tools to achieve the desired results. Additionally, there are add-ins and plugins available that offer more advanced deseasonalization capabilities for Excel users.
Yes, you can deseasonalize data in Excel for various types of datasets, including financial data, sales data, and economic data. However, it is important to note that the effectiveness of deseasonalization techniques may vary depending on the characteristics of the dataset and the nature of the seasonal patterns present.